Classification-level and Class-level Complement Information Measures Based on Neighborhood Decision Systems
COGNITIVE COMPUTATION(2021)
摘要
Information measures in neighborhood decision systems underlie information processing and uncertainty measurement, especially regarding neighborhood rough sets. Their constructions on covering structuring and neighborhood counting can acquire theoretical extensions and practical compactness but become difficult and rare when comparing the sample counting approach and its defects. In terms of three-way information measures (i.e., information entropy, conditional entropy, and mutual information), classification-level and class-level complement information measures are established by extending equivalence decision systems to neighborhood decision systems; thus, the construction on nonrepetitive neighborhoods becomes ingenious, and the study of criss-cross structuring and granulation properties becomes valuable. At the classification level, neighborhood-complement information measures are proposed by imitation; they perfectly expand existing complement information measures, and thus, they offer an extended isomorphism regarding systematicity. At the class level, neighborhood-complement information measures are determined by decomposition to generate a hierarchical isomorphism, and they also induce equivalence-complement information measures to yield a degenerate isomorphism. Then, granulation nonmonotonicity and monotonicity of neighborhood-complement information measures are revealed at these two levels, and their uncertainty mechanisms are analyzed deeply by three-level granular structures. Finally, all complement measures are calculated programmatically, and their relationships and nonmonotonicity or monotonicity are effectively verified by virtue of table examples and data experiments. In summary, systematically, there are four criss-cross modes of three-way complement information measures based on two knowledge granulations and two decision levels; the variably extended and degenerated isomorphisms and hierarchically decomposed and integrated isomorphisms are thoroughly uncovered, the granulation nonmonotonicity and monotonicity are deeply mined, and all achievements are found to have good application prospects for feature reduction and rule induction in machine learning.
更多查看译文
关键词
Neighborhood rough sets, Complement information measures, Granular computing, Granulation monotonicity and nonmonotonicity, Three-level analyses, Three-way decisions
AI 理解论文
溯源树
样例
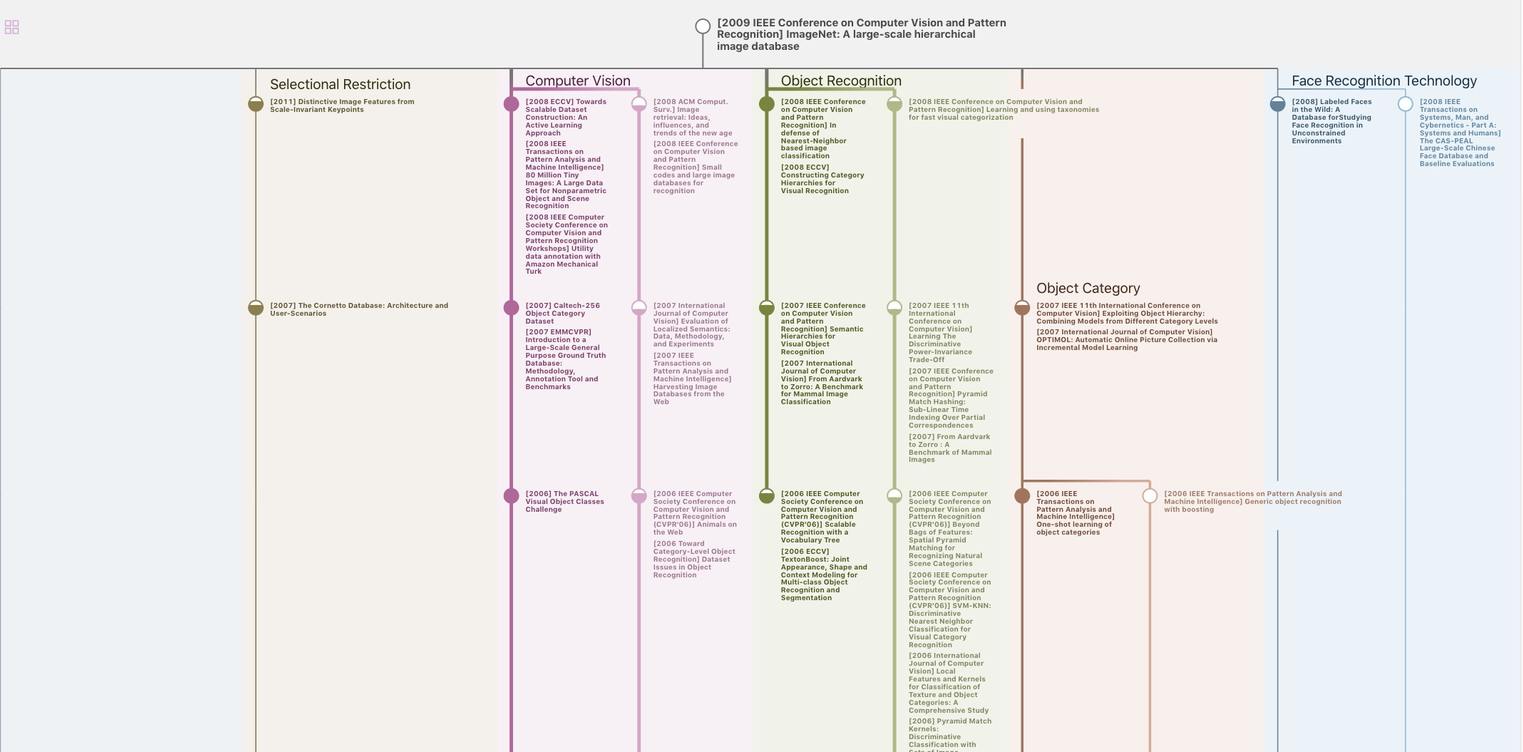
生成溯源树,研究论文发展脉络
Chat Paper
正在生成论文摘要