Self-corrected unsupervised domain adaptation
FRONTIERS OF COMPUTER SCIENCE(2021)
摘要
Unsupervised domain adaptation (UDA), which aims to use knowledge from a label-rich source domain to help learn unlabeled target domain, has recently attracted much attention. UDA methods mainly concentrate on source classification and distribution alignment between domains to expect the correct target prediction. While in this paper, we attempt to learn the target prediction end to end directly, and develop a Self-corrected unsupervised domain adaptation (SCUDA) method with probabilistic label correction. SCUDA adopts a probabilistic label corrector to learn and correct the target labels directly. Specifically, besides model parameters, those target pseudo-labels are also updated in learning and corrected by the anchor-variable, which preserves the class candidates for samples. Experiments on real datasets show the competitiveness of SCUDA.
更多查看译文
关键词
unsupervised domain adaptation, adversarial earning, deep neural network, pseudo-labels, label corrector
AI 理解论文
溯源树
样例
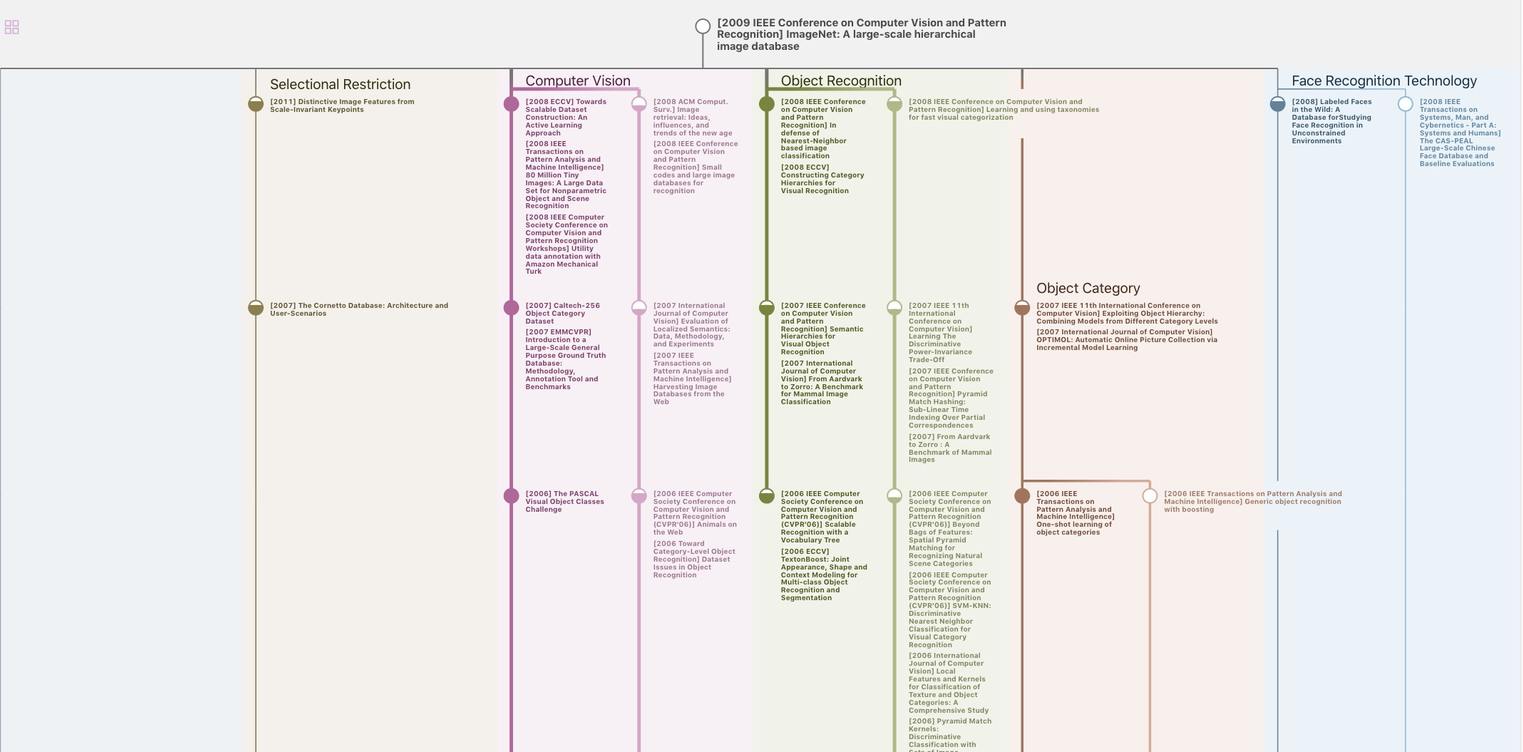
生成溯源树,研究论文发展脉络
Chat Paper
正在生成论文摘要