Group Masked Autoencoder Based Density Estimator for Audio Anomaly Detection.
DCASE(2020)
摘要
In this paper, we address the problem of detecting previously unseen anomalous audio events, when the training dataset itself does not contain any examples of anomalies. While the traditional density estimation techniques, such as Gaussian Mixture Model (GMM) showed promise in past for the problem at hand, recent advances in neural density estimation techniques, have made them suitable for anomaly detection task. In this work, we develop a novel neural density estimation technique based on the Group-Masked Autoencoder, that estimates the density of an audio time series by taking into account the intra-frame statistics of the signal. Our proposed approach has been validated using the DCASE 2020 challenge dataset (Task 2 Unsupervised Detection of Anomalous Sounds for Machine Condition Monitoring). We demonstrate the effectiveness of our approach by comparing against the baseline autoencoder model, and also against recently proposed Interpolating Deep Neural Network (IDNN) model.
更多查看译文
AI 理解论文
溯源树
样例
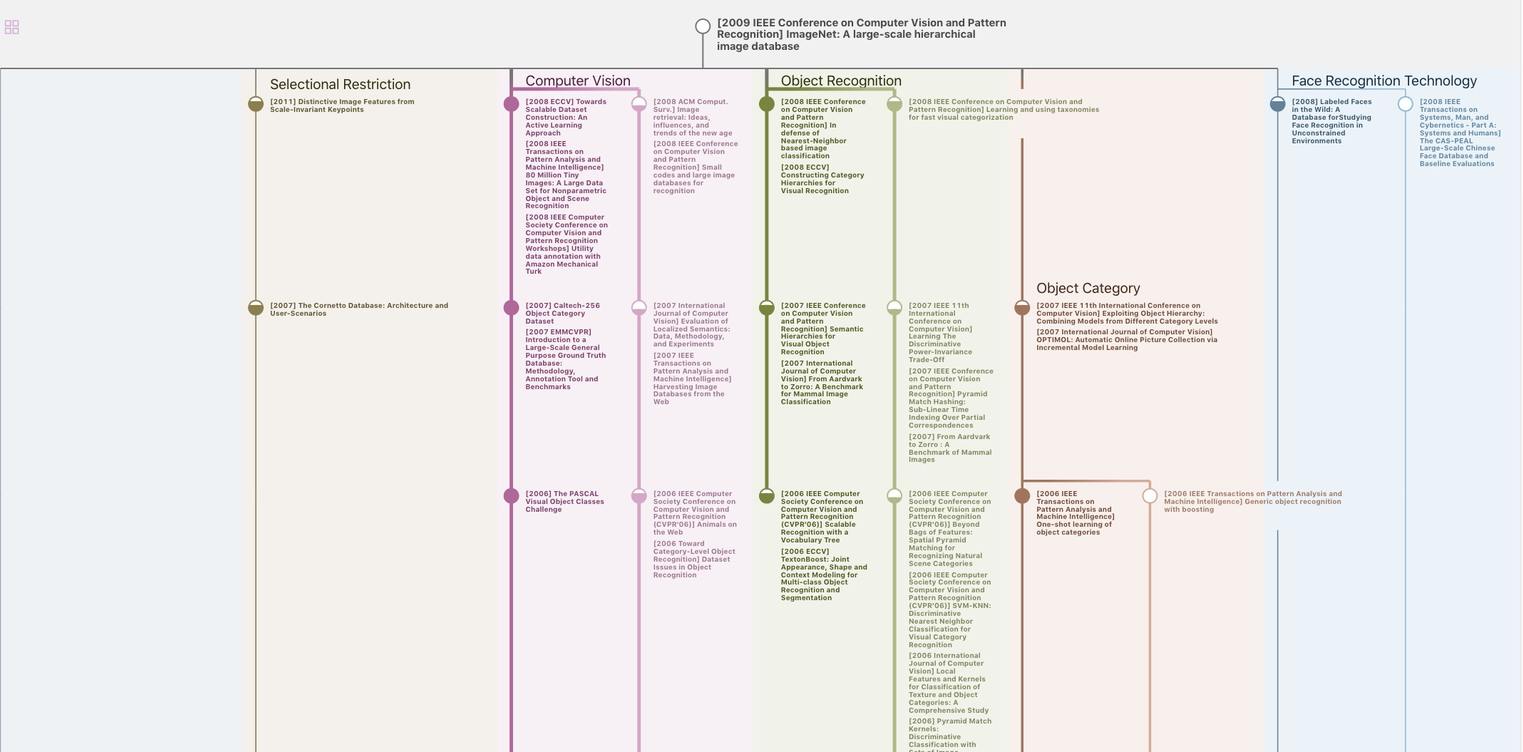
生成溯源树,研究论文发展脉络
Chat Paper
正在生成论文摘要