An Online Semi-Definite Programming with a Generalized Log-Determinant Regularizer and Its Applications
MATHEMATICS(2021)
摘要
We consider a variant of the online semi-definite programming problem (OSDP). Specifically, in our problem, the setting of the decision space is a set of positive semi-definite matrices constrained by two norms in parallel: the L-infinity norm to the diagonal entries and the Gamma-trace norm, which is a generalized trace norm with a positive definite matrix Gamma. Our setting recovers the original one when Gamma is an identity matrix. To solve this problem, we design a follow-the-regularized-leader algorithm with a Gamma-dependent regularizer, which also generalizes the log-determinant function. Next, we focus on online binary matrix completion (OBMC) with side information and online similarity prediction with side information. By reducing to the OSDP framework and applying our proposed algorithm, we remove the logarithmic factors in the previous mistake bound of the above two problems. In particular, for OBMC, our bound is optimal. Furthermore, our result implies a better offline generalization bound for the algorithm, which is similar to those of SVMs with the best kernel, if the side information is involved in advance.
更多查看译文
关键词
online semi-definite programming, log-determinant, sparse loss matrix, side information, online binary matrix completion
AI 理解论文
溯源树
样例
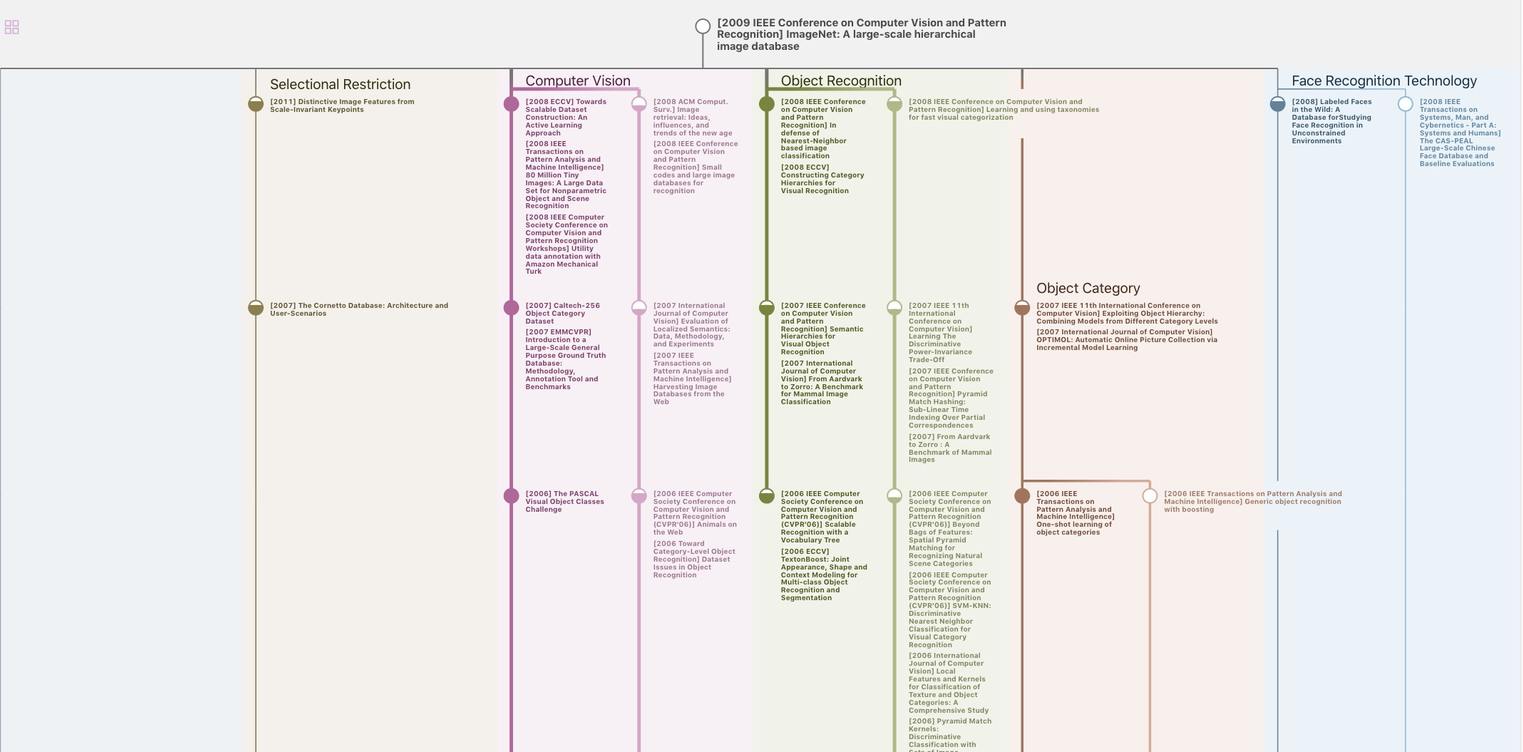
生成溯源树,研究论文发展脉络
Chat Paper
正在生成论文摘要