Multiclass Terrain Classification using Sound and Vibration from Mobile Robot Terrain Interaction
2021 IEEE/RSJ INTERNATIONAL CONFERENCE ON INTELLIGENT ROBOTS AND SYSTEMS (IROS)(2021)
摘要
Offroad mobile robot perception systems must be able to learn robust terrain classification models. Models built from computer vision often fail in their ability to generalize to new environments where appearance characteristics change. Sound and vibration signals from robot-terrain interaction can be used to classify the terrain from characteristics that vary less between environments. Previous work using sound and vibration for terrain classification has only classified ground terrain types. We extend here to building a 7-class multiclass classifier that can classify both ground and above-ground terrain types in challenging outdoor off-road settings, thereby increasing the semantic richness of the terrain classification. Our contributions include: 1) We instrument a robotic vehicle with a variety of sound and vibration sensors mounted at different vehicle locations and directions, as well as color cameras. 2) We collect interactive and visual field data from many outdoor off-road sites with different environments. 3) We build multiclass classifiers for different combinations of sound and vibration signals, and we autonomously learn the optimal signal combination. We compare this against a single microphone from our previous work [1]. 4) We benchmark both of these results against a state-of-the art vision system. All of these multiclass classifiers are tested at different locations from where they are trained. By using one microphone instead of the vision system, we increase balanced accuracy from 70% to 82%. By using the optimal sound and vibration combination, we increase balanced accuracy from 82% to 87%. All four of these contributions are field robotics in nature: we build a sensor system and then we use that system to collect new field data that allows for a comparative evaluation of different modules of the system. Such datasets do not exist that include these varying sensors on varying field terrain. We are also contributing to machine learning research by a) showing how the acoustic classification from our previous work can be extended to new sensors, and then b) implementing an additional learning process for choosing the optimal combination.
更多查看译文
关键词
previous work,state-of-the art vision system,multiclass classifiers,balanced accuracy,optimal sound,vibration combination,field robotics,sensor system,field terrain,acoustic classification,multiclass terrain classification,mobile robot terrain interaction,mobile robot perception systems,robust terrain classification models,computer vision,appearance characteristics change,vibration signals,robot-terrain interaction,7-class multiclass classifier,above-ground terrain types,robotic vehicle,vibration sensors,different vehicle locations,interactive field data,visual field data,optimal signal combination
AI 理解论文
溯源树
样例
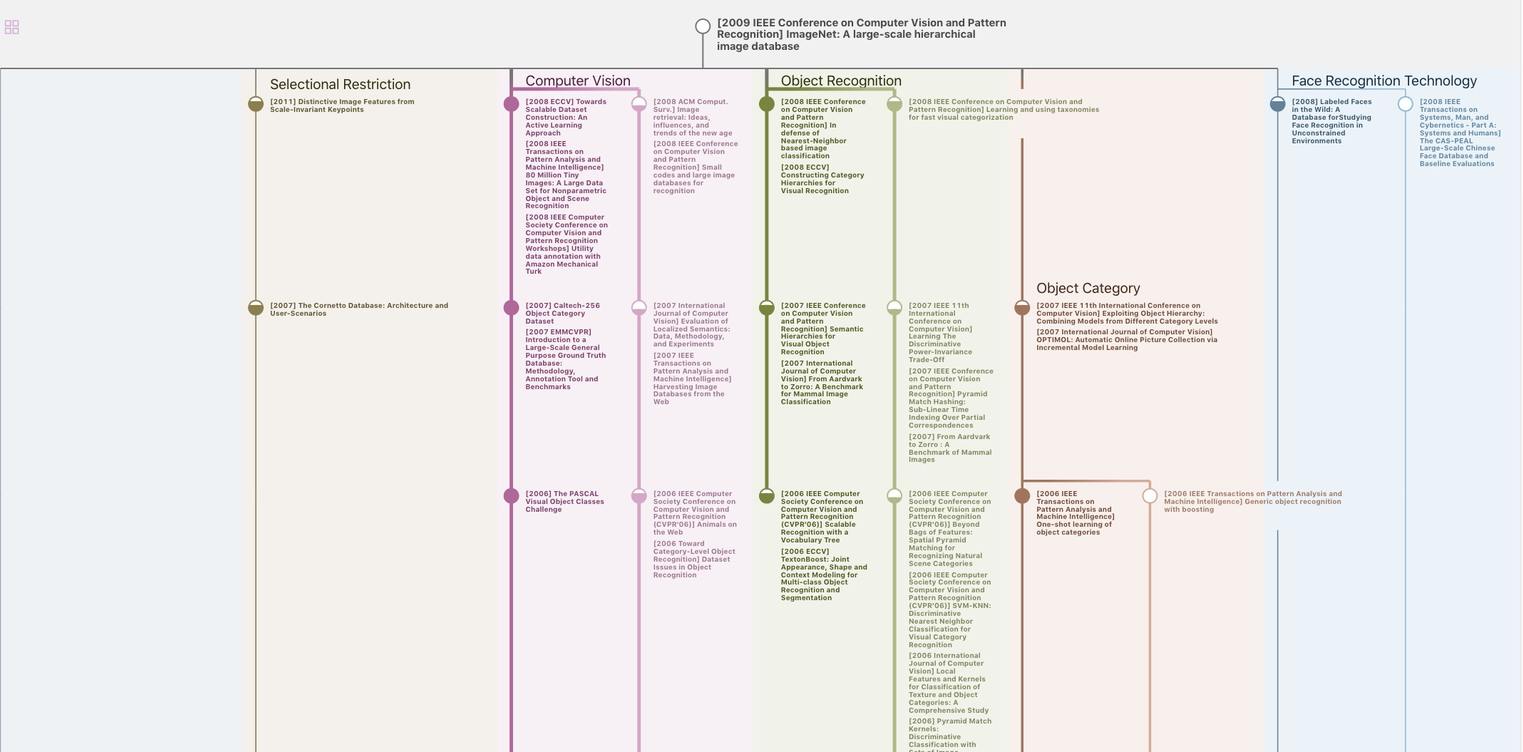
生成溯源树,研究论文发展脉络
Chat Paper
正在生成论文摘要