TributaryPCA: Distributed, Streaming PCA for in Situ Dimension Reduction with Application to Space Weather Simulations
PROCEEDINGS OF THE 7TH INTERNATIONAL WORKSHOP ON DATA ANALYSIS AND REDUCTION FOR BIG SCIENTIFIC DATA (DRBSD-7)(2021)
摘要
Computer simulations continue to grow in size and complexity and are moving towards exascale. Simulations at this scale can generate outputs that exceed both storage capacity and the bandwidth available for transferring to storage, making traditional offline statistical inference challenging. Therefore, it is desirable to embed statistical analyses in the simulation framework while the simulation is running - a strategy called in situ inference - to alleviate the burden of storage. In this work, we focus on adapting Principal Component Analysis (PCA) - a statistical method for reducing dimensionality of big data - to the in situ setting. We develop TributaryPCA: a distributed version of Oja's algorithm for streaming PCA that uses the Message Passing Interface (MPI) standard. Our approach significantly reduces data storage requirements of offline PCA and avoids excessive communication across compute nodes. We illustrate the method using data generated from the SHIELDS Framework for space weather simulation.
更多查看译文
关键词
Dimension reduction,Distributed data,In situ inference,Principal component analysis,Space weather
AI 理解论文
溯源树
样例
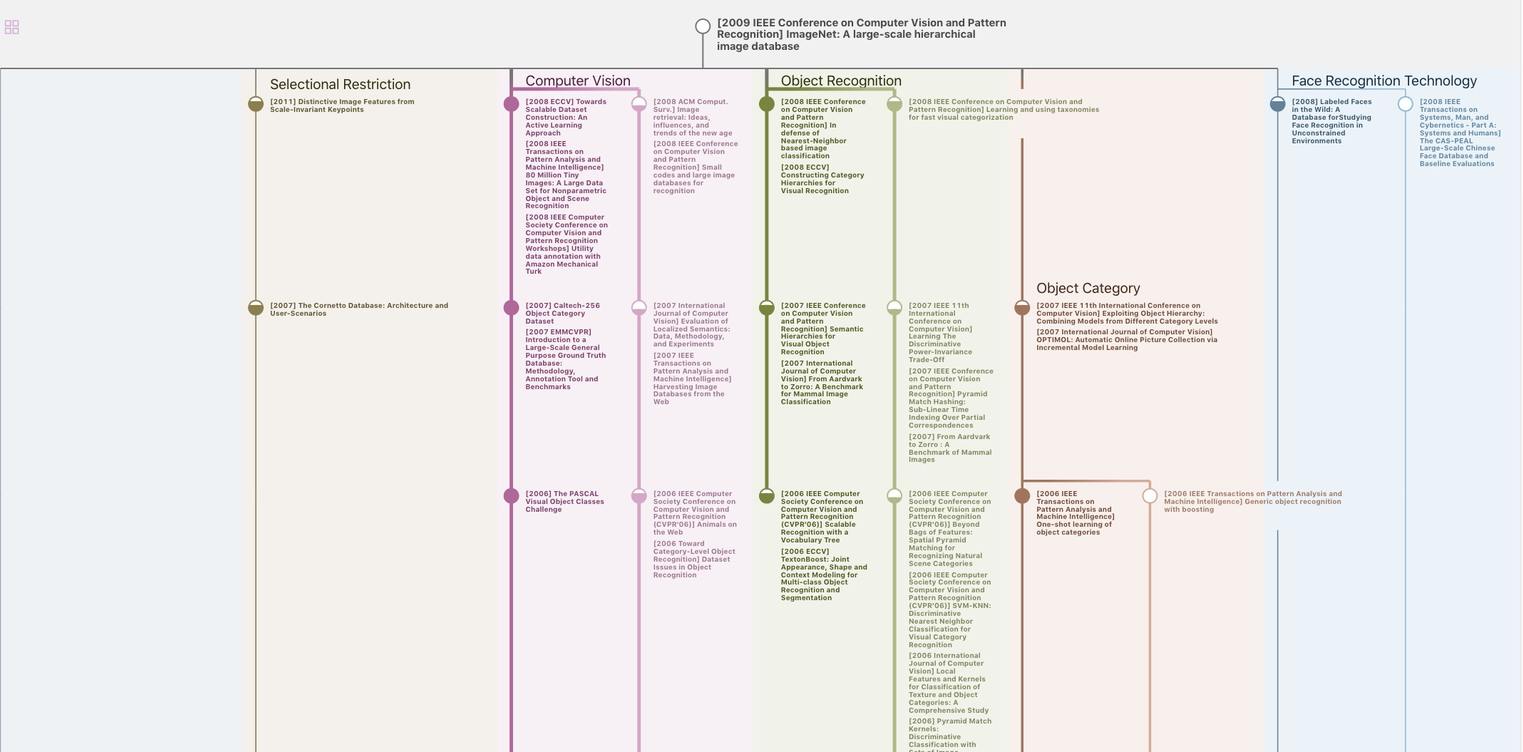
生成溯源树,研究论文发展脉络
Chat Paper
正在生成论文摘要