Underwater 3D Object Reconstruction for Fish Length Estimation Using Convolutional Neural Networks
2021 International Symposium on Intelligent Signal Processing and Communication Systems (ISPACS)(2021)
摘要
The challenge in stereo matching for underwater 3D object modeling is to compute dense depth data with the minimal smoothness at high frame rate. To address this issue, in this paper we propose an object based stereo matching for underwater 3D fish reconstruction using convolutional neural networks (CNNs). For each image in a stereo frame, an instance segmentation CNN is used to segment fish objects from the background. The set of fish objects in the left image is matched against those in the right to detect the object pairs using the proposed support-weights approach. For each pair, the common disparity value is then computed. Next, fish objects in these images are cropped and matched to do the pixel-wise residual disparity computation using the video interpolation CNN. The computed fish disparities and depth values are finally used to estimate the sizes of fish. Instead of estimating the fish length using a single frame, we track each fish across frames of the input stereo video to compute the fish length frame by frame. The mean fish length is finally computed as the result. An underwater dataset with the fish actual length measured by human is constructed to verify the effectiveness of our approach. Experimental results show that the error rate of the proposed approach is less than 6%.
更多查看译文
关键词
Deep learning,Training,Solid modeling,Image segmentation,Three-dimensional displays,Signal processing algorithms,Estimation
AI 理解论文
溯源树
样例
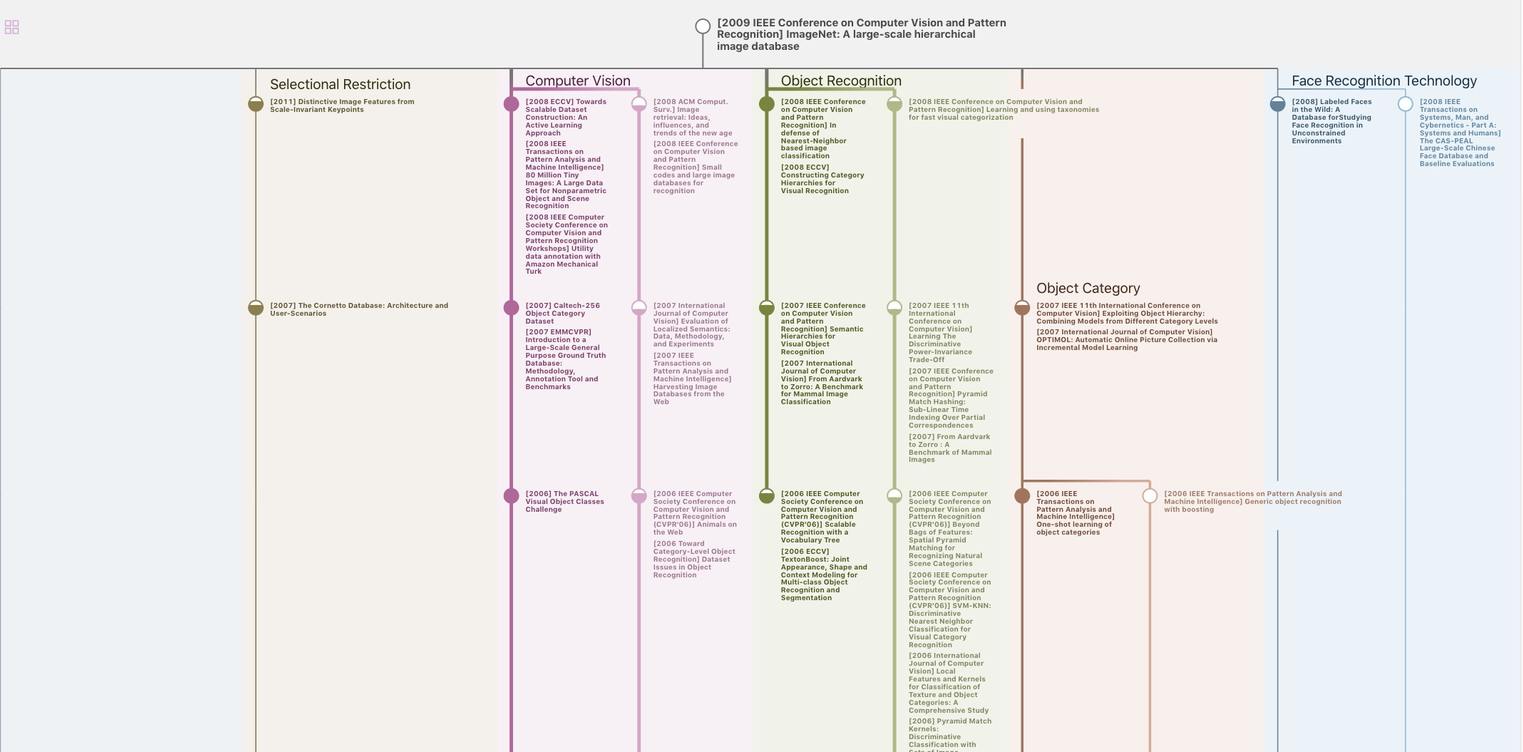
生成溯源树,研究论文发展脉络
Chat Paper
正在生成论文摘要