Background-Aware Classification Activation Map for Weakly Supervised Object Localization
IEEE TRANSACTIONS ON PATTERN ANALYSIS AND MACHINE INTELLIGENCE(2023)
摘要
Weakly supervised object localization (WSOL) relaxes the requirement of dense annotations for object localization by using image-level annotation to supervise the learning process. However, most WSOL methods only focus on forcing the object classifier to produce high activation score on object parts without considering the influence of background locations, causing excessive background activations and ill-pose background score searching. Based on this point, our work proposes a novel mechanism called the background-aware classification activation map (B-CAM) to add background awareness for WSOL training. Besides aggregating an object image-level feature for supervision, our B-CAM produces an additional background image-level feature to represent the pure-background sample. This additional feature can provide background cues for the object classifier to suppress the background activations on object localization maps. Moreover, our B-CAM also trained a background classifier with image-level annotation to produce adaptive background scores when determining the binary localization mask. Experiments indicate the effectiveness of the proposed B-CAM on four different types of WSOL benchmarks, including CUB-200, ILSVRC, OpenImages, and VOC2012 datasets.
更多查看译文
关键词
Object localization,weakly supervised learning,weakly supervised object localization
AI 理解论文
溯源树
样例
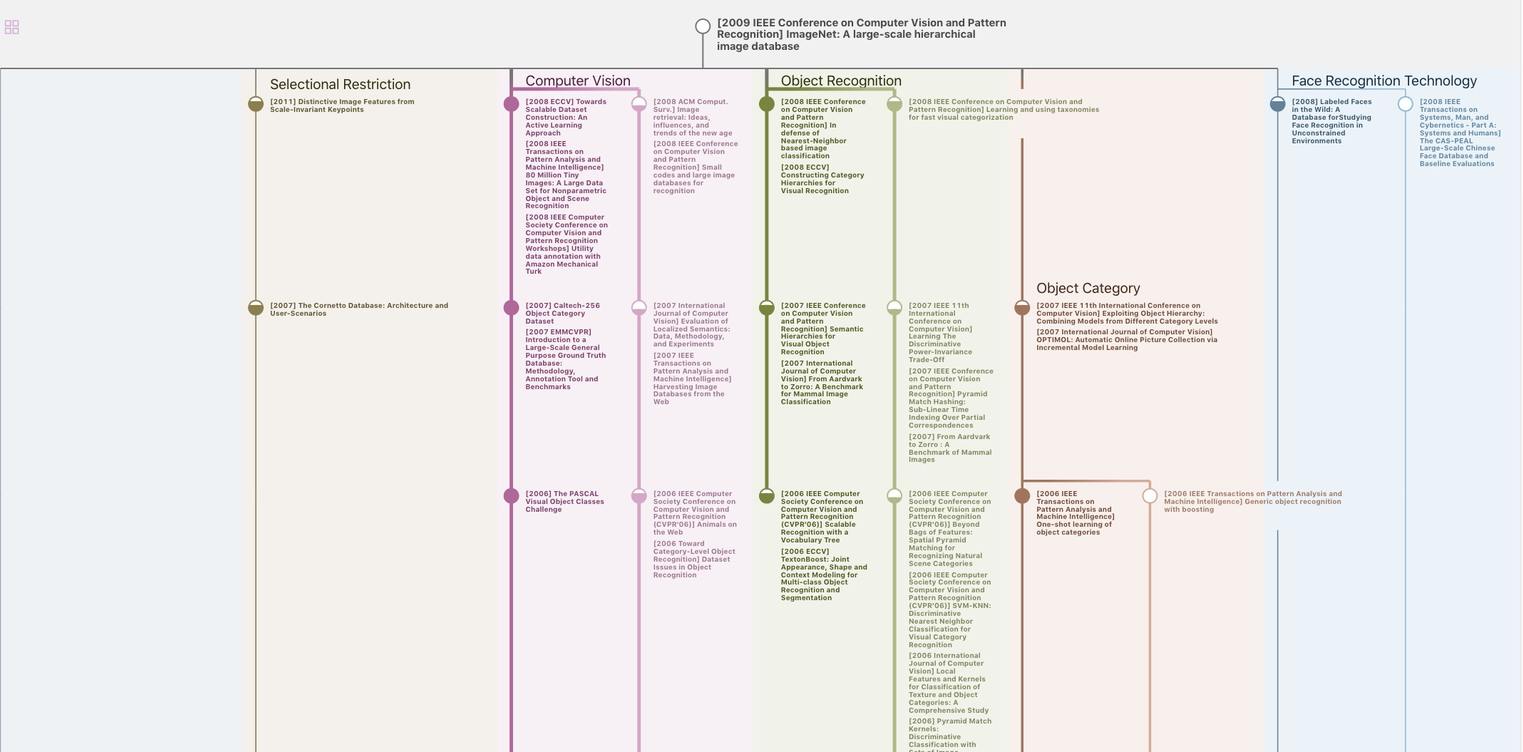
生成溯源树,研究论文发展脉络
Chat Paper
正在生成论文摘要