Shallow decision trees for explainable k-means clustering
arxiv(2023)
摘要
A number of recent works have employed decision trees for the construction of explainable partitions that aim to minimize the k-means cost function. These works, however, largely ignore metrics related to the depths of the leaves in the resulting tree, which is perhaps surprising considering how the explain -ability of a decision tree depends on these depths. To fill this gap in the literature, we propose an efficient algorithm with a penalty term in its loss function to favor the construction of shallow decision trees - i.e., trees whose leaves are not very deep, which translate to clusters that are defined by a small number of attributes and are therefore easier to explain. In experiments on 16 datasets, our algorithm yields better results than decision-tree clustering algorithms recently presented in the literature, typically achieving lower or equivalent costs with considerably shallower trees.(c) 2022 Elsevier Ltd. All rights reserved.
更多查看译文
关键词
Clustering,Explainability,K -means,Decision trees
AI 理解论文
溯源树
样例
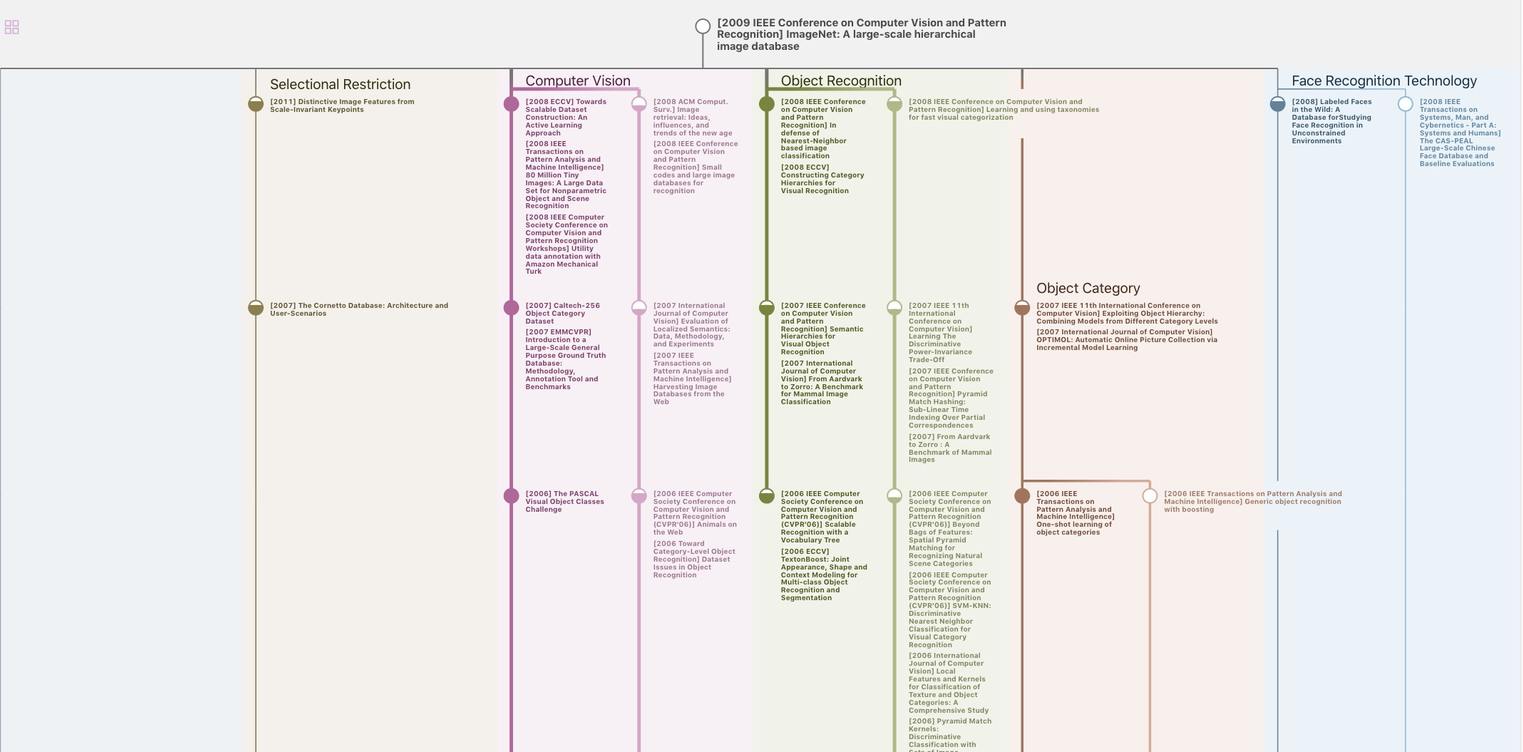
生成溯源树,研究论文发展脉络
Chat Paper
正在生成论文摘要