Adaptive Optimization of Traffic Signal Timing via Deep Reinforcement Learning
JOURNAL OF ADVANCED TRANSPORTATION(2021)
摘要
With rapid development of the urbanization, how to improve the traffic lights efficiency has become an urgent issue. The traditional traffic light control is a method that calculates a series of corresponding timing parameters by optimizing the cycle length. However, fixing sequence and duration of traffic lights is inefficient for dynamic traffic flow regulation. In order to solve the above problem, this study proposes a traffic light timing optimization scheme based on deep reinforcement learning (DRL). In this scheme, the traffic lights can output an appropriate phase according to the traffic flow state of each direction at the intersection and dynamically adjust the phase length. Specifically, we first adopt Proximal Policy Optimization (PPO) to improve the convergence speed of the model. Then, we elaborate the design of state, action, and reward, with the vehicle state defined by Discrete Traffic State Encoding (DTSE) method. Finally, we conduct experiments on real traffic data via the traffic simulation platform SUMO. The results show that, compared to the traditional timing control, the proposed scheme can effectively reduce the waiting time of vehicles and queue length in various traffic flow modes.
更多查看译文
AI 理解论文
溯源树
样例
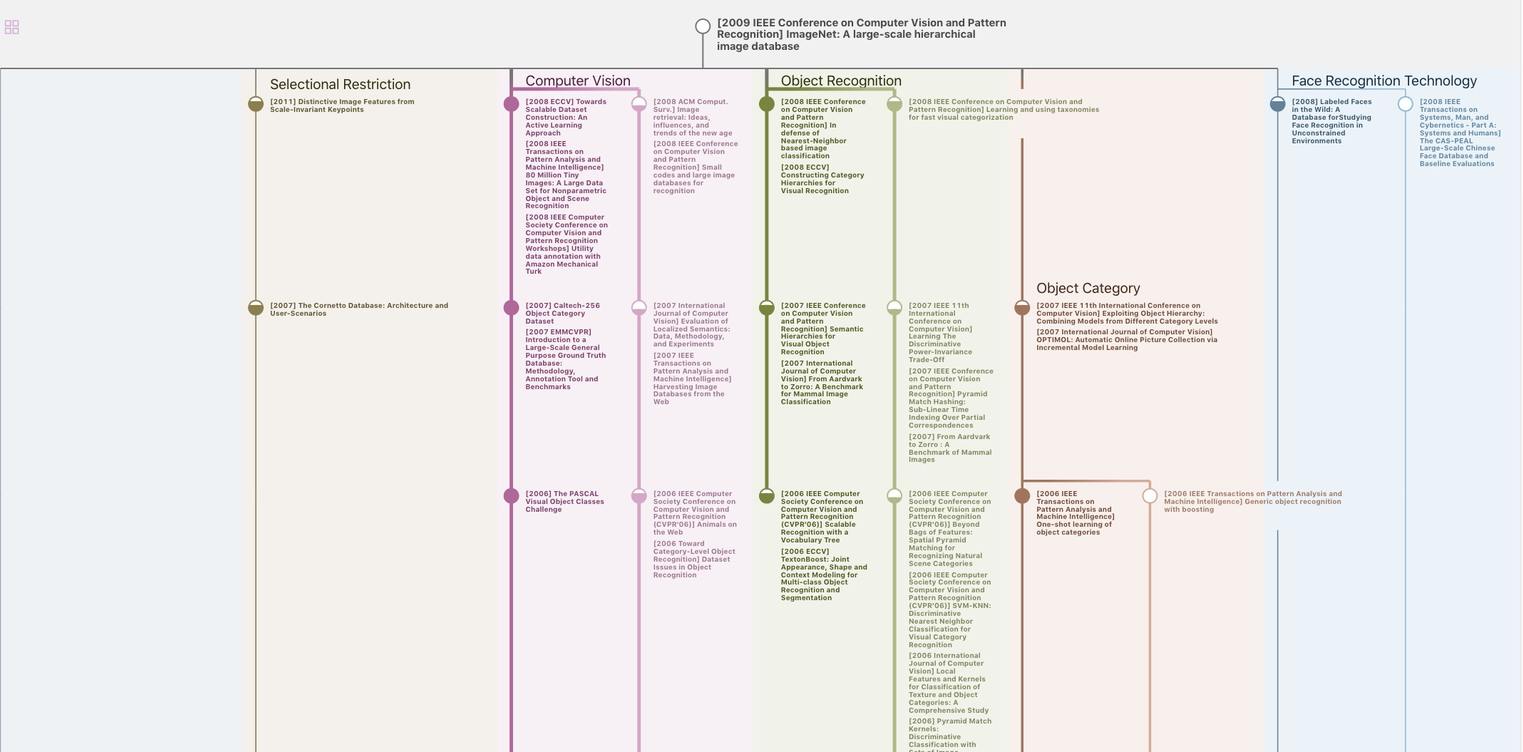
生成溯源树,研究论文发展脉络
Chat Paper
正在生成论文摘要