Dual Prior Learning for Blind and Blended Image Restoration
IEEE Transactions on Image Processing(2022)
摘要
Unsupervised single image restoration approach, Deep Image Prior (DIP), aims to restore images by learning enough raw image statistic priors from the corrupted observation. However, it is not uncommon that an image is contaminated by the multiple unknown distortions. Thus it is hard to disentangle the clean and the hybrid distortion signals by solely relying on image prior learning to restore the images. To overcome this problem, we propose the Dual Prior Learning (DPL) method by taking both image and distortion priors into account. DPL goes beyond DIP by considering an additional step to explicitly learn the blended distortion prior. Furthermore, to coordinate the learning of two priors and avoid them learning the same knowledge, we exploit unpaired training data to enforce a weakly supervision in an adversarial manner to encourage disentangling two priors. Extensive experiments show the effectiveness and appealing performance of the proposed DPL on restoring images with challenging unknown blended distortions.
更多查看译文
关键词
Weakly-supervised image restoration,deep image prior,dual prior learning,disentanglement
AI 理解论文
溯源树
样例
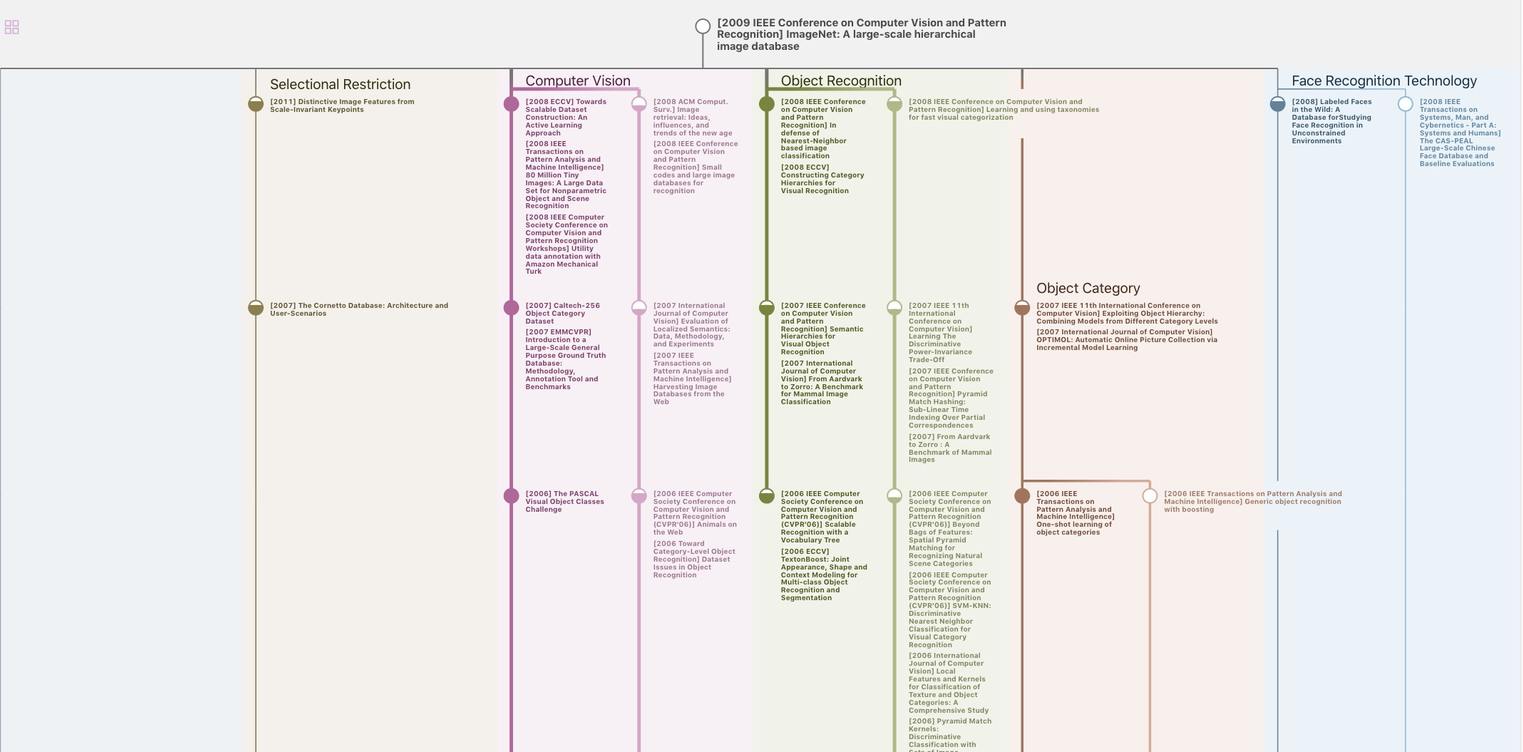
生成溯源树,研究论文发展脉络
Chat Paper
正在生成论文摘要