Deep learning and radiomics based automatic diagnosis of hippocampal sclerosis.
The International journal of neuroscience(2021)
摘要
Accurate and rapid segmentation of the hippocampus can help doctors perform intractable temporal lobe epilepsy (TLE) preoperative evaluations to identify good surgical candidates. This study aims to establish a radiomics system for the automatic diagnosis of hippocampal sclerosis with the help of machine learning. A total of 240 cases were analysed to develop a diagnostic model. First, an automatic hippocampal segmentation process was established that exploits a priori knowledge of the relatively fixed location of the hippocampus in brain partitions, as well as a deep-learning segmentation network based on an Attention U-net. Then, we extracted 527 radiomics features from each side of the segmented hippocampus. The iterative sparse representation based on feature selection and a support vector machine classifier were finally used to establish the diagnostic model of hippocampal sclerosis. The diagnostic model consists of two consecutive steps: distinguish hippocampal sclerosis (HS) from normal control (NC) and detect whether the HS is located on the left or right side. When the automatic diagnosis model identified HS and NC, the sensitivity and specificity reached 0.941 and 0.917 in the 10-fold cross-validation set and 0.920 and 0.909 in the independent testing set. When the diagnostic model detected HS lateralization, the sensitivity and specificity reached 0.923 and 0.920 in cross-validation and 0.909 and 0.929 in independent testing. Our results show that the developed radiomics model can help detect TLE patients with hippocampal sclerosis and has the potential to simplify preoperative evaluations and select surgical candidates.
更多查看译文
关键词
Hippocampal sclerosis,attention U-net,deep learning,radiomics
AI 理解论文
溯源树
样例
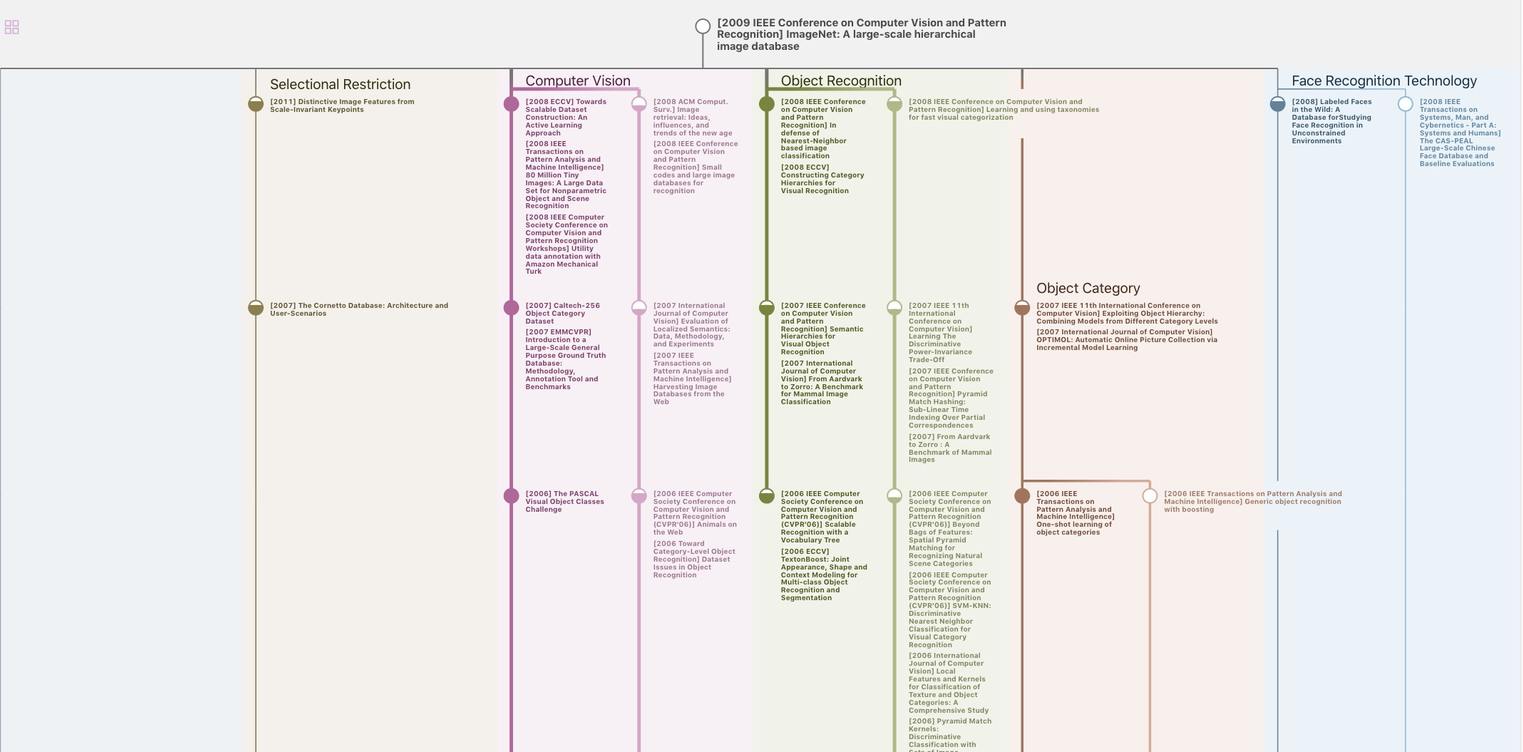
生成溯源树,研究论文发展脉络
Chat Paper
正在生成论文摘要