Greedy Autoaugment for classification of mycobacterium tuberculosis image via generalized deep CNN using mixed pooling based on minimum square rough entropy
COMPUTERS IN BIOLOGY AND MEDICINE(2022)
摘要
Although tuberculosis (TB) is a disease whose cause, epidemiology and treatment are well known, some infected patients in many parts of the world are still not diagnosed by current methods, leading to further transmission in society. Creating an accurate image-based processing system for screening patients can help in the early diag-nosis of this disease. We provided a dataset containing1078 confirmed negative and 469 positive Mycobacterium tuberculosis instances. An effective method using an improved and generalized convolutional neural network (CNN) was proposed for classifying TB bacteria in microscopic images. In the preprocessing phase, the insig-nificant parts of microscopic images are excluded with an efficient algorithm based on the square rough entropy (SRE) thresholding. Top 10 policies of data augmentation were selected with the proposed model based on the Greedy AutoAugment algorithm to resolve the overfitting problem. In order to improve the generalization of CNN, mixed pooling was used instead of baseline one. The results showed that employing generalized pooling, batch normalization, Dropout, and PReLU have improved the classification of Mycobacterium tuberculosis im-ages. The output of classifiers such as Naive Bayes-LBP, KNN-LBP, GBT-LBP, Naive Bayes-HOG, KNN-HOG, SVM-HOG, GBT-HOG indicated that proposed CNN has the best results with an accuracy of 93.4%. The improvements of CNN based on the proposed model can yield promising results for diagnosing TB.
更多查看译文
关键词
Greedy Autoaugment, Tuberculosis, Convolutional neural network, Dropout, Mixed pooling, Deep learning
AI 理解论文
溯源树
样例
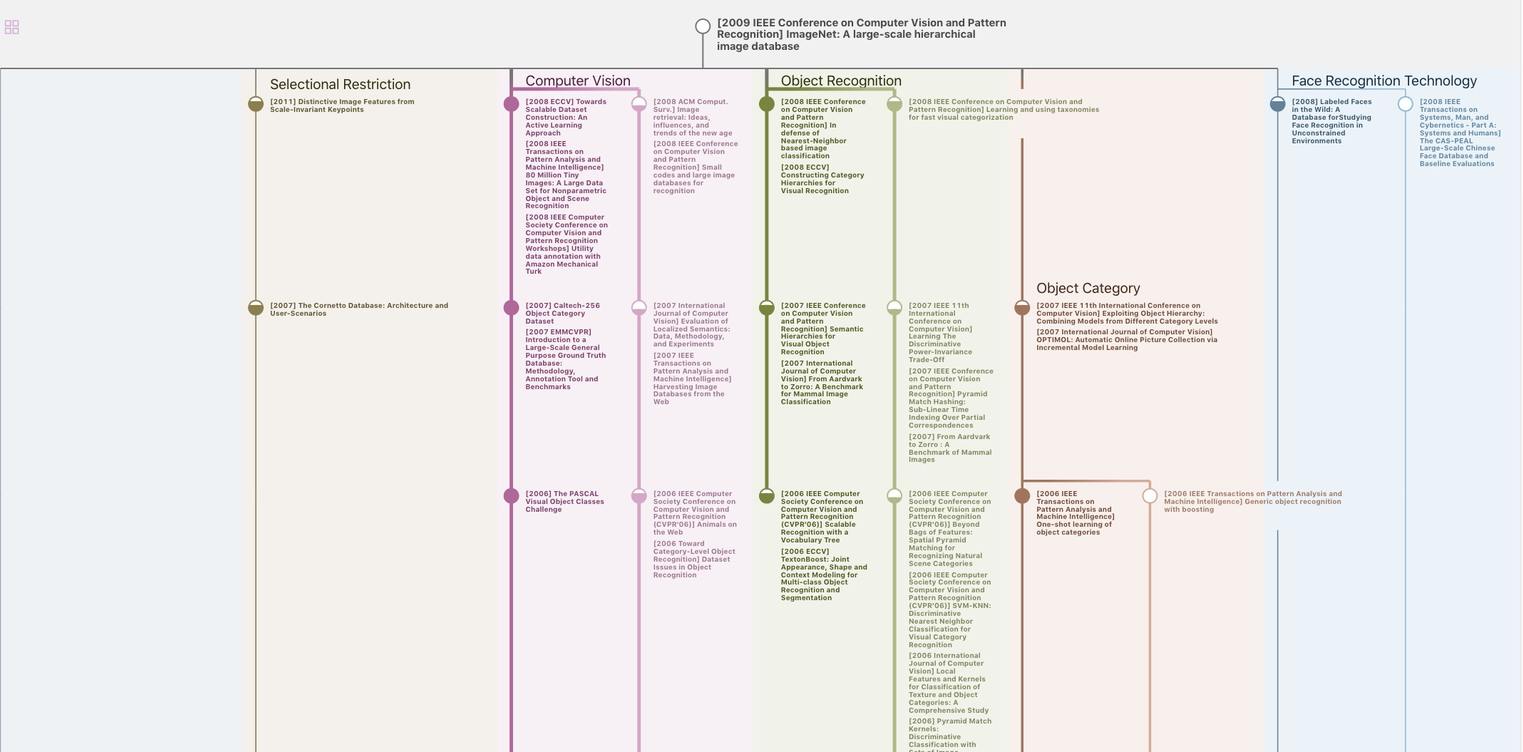
生成溯源树,研究论文发展脉络
Chat Paper
正在生成论文摘要