Effective and Efficient PageRank-based Positioning for Graph Visualization
arxiv(2023)
摘要
Graph visualization is a vital component in many real-world applications (e.g., social network analysis, web mining, and bioinformatics) that enables users to unearth crucial insights from complex data. Lying in the core of graph visualization is the node distance measure, which determines how the nodes are placed on the screen. A favorable node distance measure should be informative in reflecting the full structural information between nodes and effective in optimizing visual aesthetics. However, existing node distance measures yield sub-par visualization quality as they fall short of these requirements. Moreover, most existing measures are computationally inefficient, incurring a long response time when visualizing large graphs. To overcome such deficiencies, we propose a new node distance measure, PDist, geared towards graph visualization by exploiting a well-known node proximity measure, personalized PageRank. Moreover, we propose an efficient algorithm Tau-Push for estimating PDist under both single- and multi-level visualization settings. With several carefully-designed techniques, Tau-Push offers non-trivial theoretical guarantees for estimation accuracy and computation complexity. Extensive experiments show that our proposal significantly outperforms 13 state-of-the-art graph visualization solutions on 12 real-world graphs in terms of both efficiency and effectiveness (including aesthetic criteria and user feedback). In particular, our proposal can interactively produce satisfactory visualizations within one second for billion-edge graphs.
更多查看译文
关键词
visualization,graph,positioning,pagerank-based
AI 理解论文
溯源树
样例
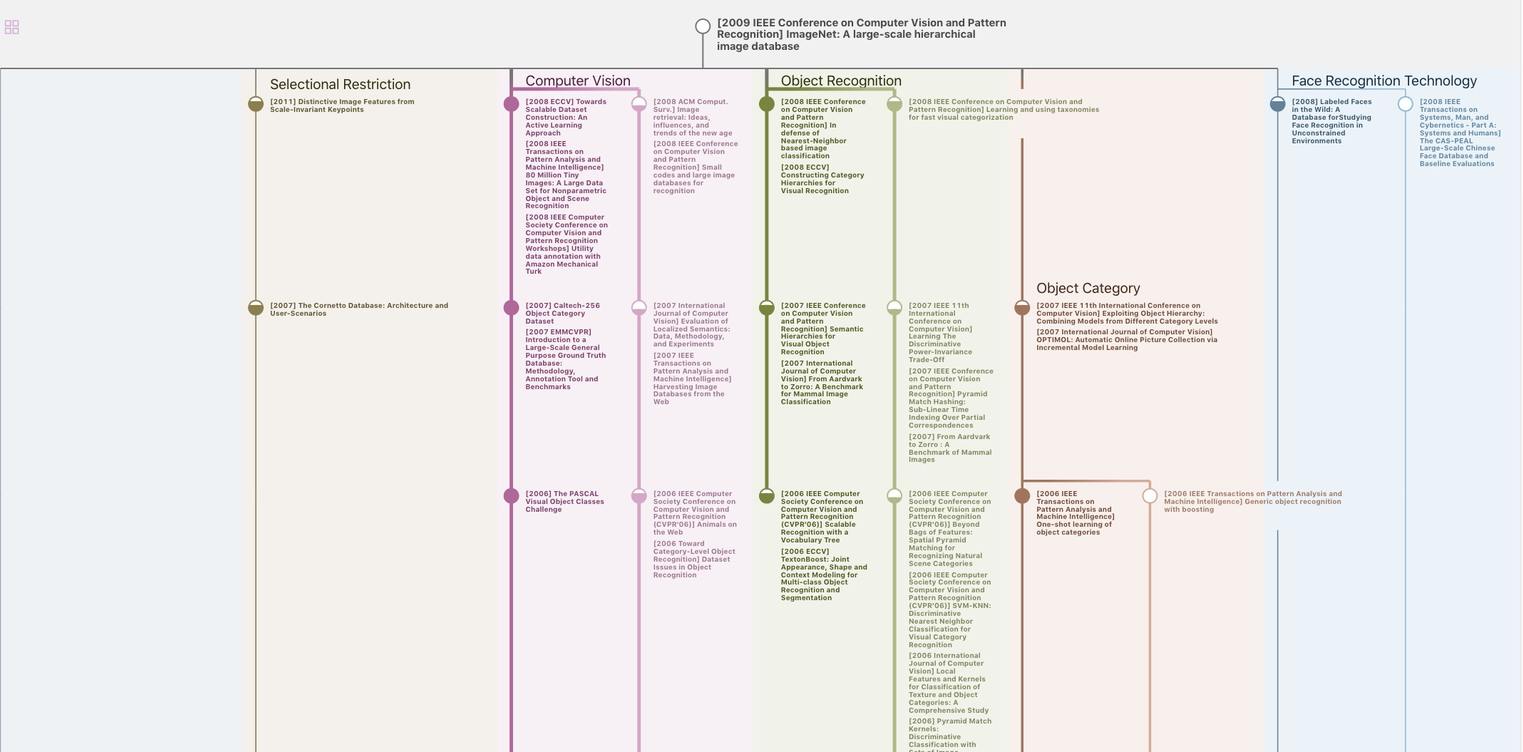
生成溯源树,研究论文发展脉络
Chat Paper
正在生成论文摘要