Knowledge matters: Chest radiology report generation with general and specific knowledge
MEDICAL IMAGE ANALYSIS(2022)
摘要
Automatic chest radiology report generation is critical in clinics which can relieve experienced radiologists from the heavy workload and remind inexperienced radiologists of misdiagnosis or missed diagnose. Existing approaches mainly formulate chest radiology report generation as an image captioning task and adopt the encoder-decoder framework. However, in the medical domain, such pure data-driven approaches suffer from the following problems: 1) visual and textual bias problem; 2) lack of expert knowledge. In this paper, we propose a knowledge-enhanced radiology report generation approach introduces two types of medical knowledge: 1) General knowledge, which is input independent and provides the broad knowledge for report generation; 2) Specific knowledge, which is input dependent and provides the fine-grained knowledge for chest X-ray report generation. To fully utilize both the general and specific knowledge, we also propose a knowledge-enhanced multi-head attention mechanism. By merging the visual features of the radiology image with general knowledge and specific knowledge, the proposed model can improve the quality of generated reports. The experimental results on the publicly available IU-Xray dataset show that the proposed knowledge-enhanced approach outperforms state-of-the-art methods in almost all metrics. And the results of MIMIC-CXR dataset show that the proposed knowledge-enhanced approach is on par with state-of-the-art methods. Ablation studies also demonstrate that both general and specific knowledge can help to improve the performance of chest radiology report generation.(c) 2022 The Author(s). Published by Elsevier B.V. This is an open access article under the CC BY-NC-ND license( http://creativecommons.org/licenses/by-nc-nd/4.0/ )
更多查看译文
关键词
Chest radiology report generation, Knowledge graph, Multi-head attention
AI 理解论文
溯源树
样例
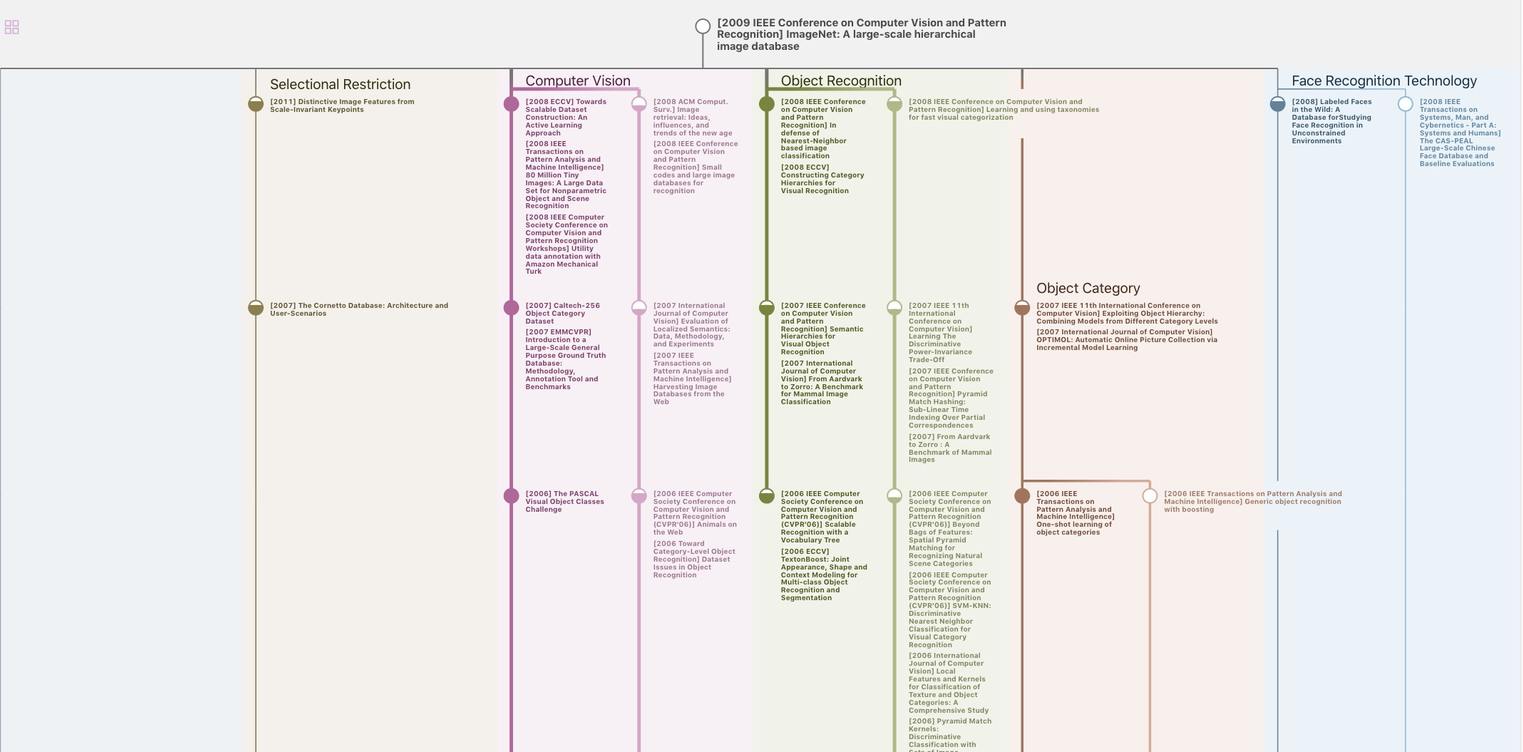
生成溯源树,研究论文发展脉络
Chat Paper
正在生成论文摘要