Revisiting Open World Object Detection
arxiv(2022)
摘要
Open World Object Detection (OWOD), simulating the real dynamic world where knowledge grows continuously, attempts to detect both known and unknown classes and incrementally learn the identified unknown ones. We find that although the only previous OWOD work constructively puts forward to the OWOD definition, the experimental settings are unreasonable with the illogical benchmark, confusing metric calculation, and inappropriate method. In this paper, we rethink the OWOD experimental setting and propose five fundamental benchmark principles to guide the OWOD benchmark construction. Moreover, we design two fair evaluation protocols specific to the OWOD problem, filling the void of evaluating from the perspective of unknown classes. Furthermore, we introduce a novel and effective OWOD framework containing an auxiliary Proposal ADvisor (PAD) and a Class-specific Expelling Classifier (CEC). The non-parametric PAD could assist the RPN in identifying accurate unknown proposals without supervision, while CEC calibrates the over-confident activation boundary and filters out confusing predictions through a class-specific expelling function. Comprehensive experiments conducted on our fair benchmark demonstrate that our method outperforms other state-of-the-art object detection approaches in terms of both existing and our new metrics.\footnote{Our benchmark and code are available at https://github.com/RE-OWOD/RE-OWOD.
更多查看译文
关键词
open world,object detection,auxiliary proposal advisor,class-specific expelling classifier
AI 理解论文
溯源树
样例
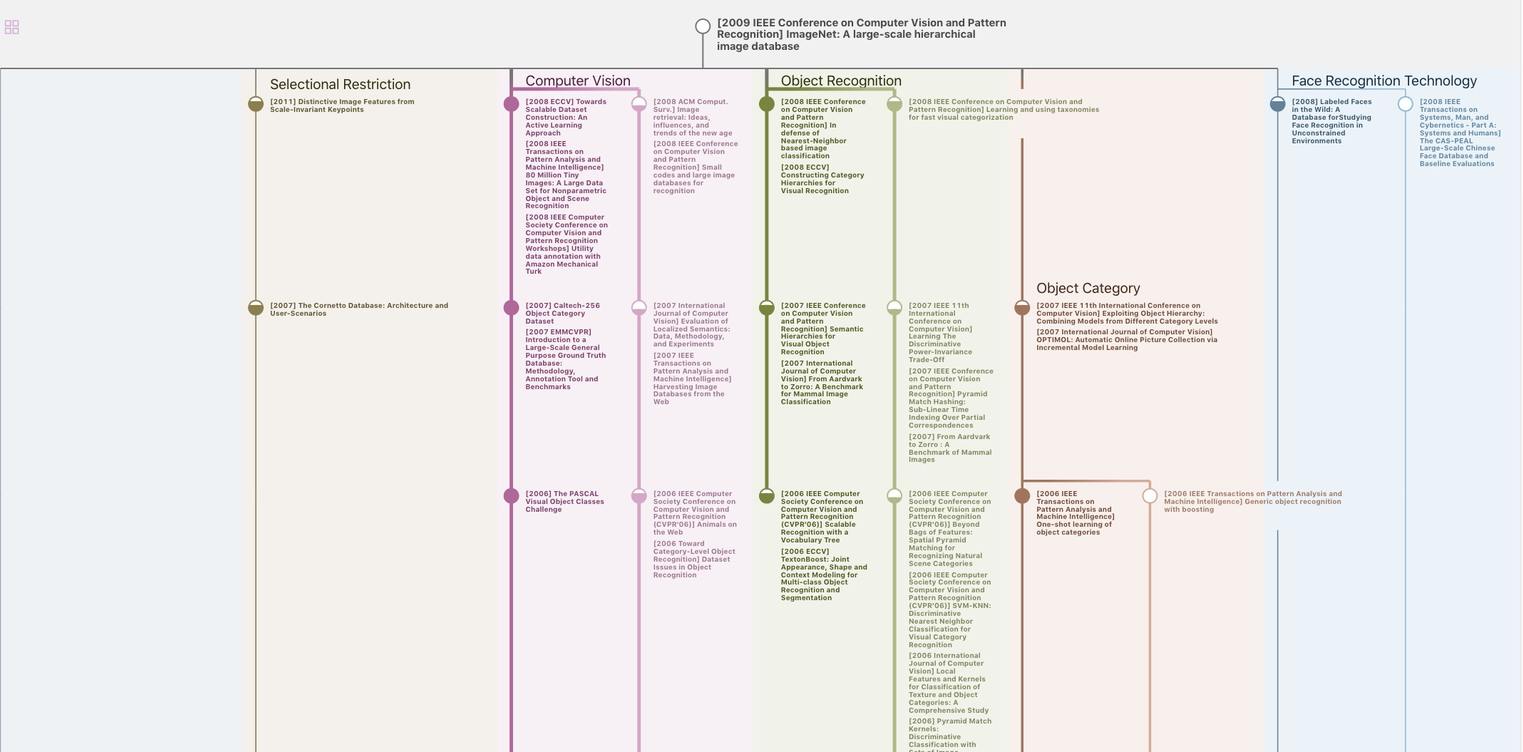
生成溯源树,研究论文发展脉络
Chat Paper
正在生成论文摘要