Neural network training under semidefinite constraints
arxiv(2022)
摘要
This paper is concerned with the training of neural networks (NNs) under semidefinite constraints, which allows for NN training with robustness and stability guarantees. In particular, we focus on Lipschitz bounds for NNs. Exploiting the banded structure of the underlying matrix constraint, we set up an efficient and scalable training scheme for NN training problems of this kind based on interior point methods. Our implementation allows to enforce Lipschitz constraints in the training of large-scale deep NNs such as Wasserstein generative adversarial networks (WGANs) via semidefinite constraints. In numerical examples, we show the superiority of our method and its applicability to WGAN training.
更多查看译文
关键词
neural network training,constraints
AI 理解论文
溯源树
样例
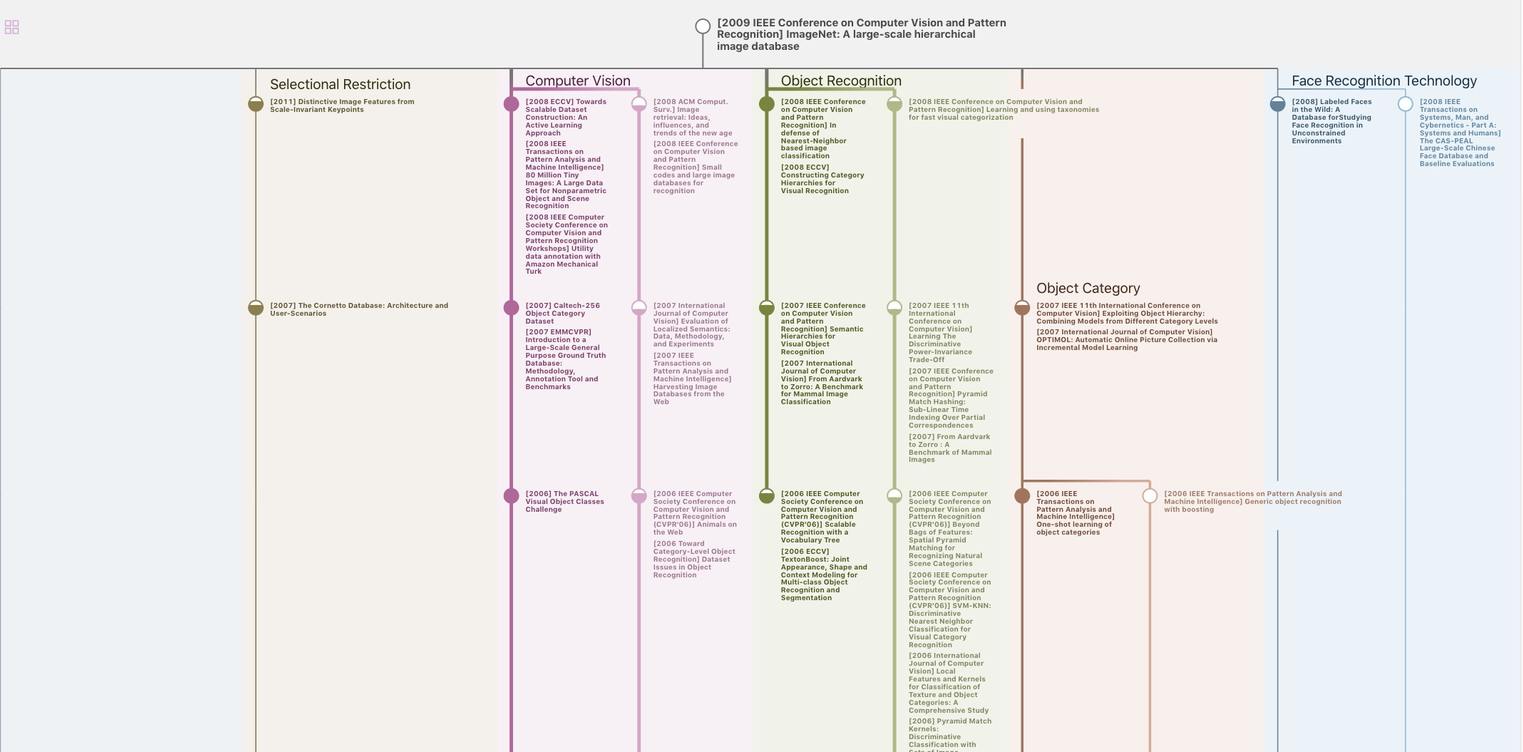
生成溯源树,研究论文发展脉络
Chat Paper
正在生成论文摘要