Multiview point cloud registration with anisotropic and space-varying localization noise
arxiv(2022)
摘要
In this paper, we address the problem of registering multiple point clouds corrupted with high anisotropic localization noise. Our approach follows the widely used framework of Gaussian mixture model (GMM) reconstruction with an expectation-maximization (EM) algorithm. Existing methods are based on an implicit assumption of space-invariant isotropic Gaussian noise. However, this assumption is violated in practice in applications such as single molecule localization microscopy (SMLM). To address this issue, we propose to introduce an explicit localization noise model that decouples shape modeling with the GMM from noise handling. We design a stochastic EM algorithm that considers noise-free data as a latent variable, with closed-form solutions at each EM step. The first advantage of our approach is to handle space-variant and anisotropic Gaussian noise with arbitrary covariances. The second advantage is to leverage the explicit noise model to impose prior knowledge about the noise that may be available from physical sensors. We show on various simulated data that our noise handling strategy improves significantly the robustness to high levels of anisotropic noise. We also demonstrate the performance of our method on real SMLM data.
更多查看译文
关键词
multiview point cloud registration,localization,anisotropic,space-varying
AI 理解论文
溯源树
样例
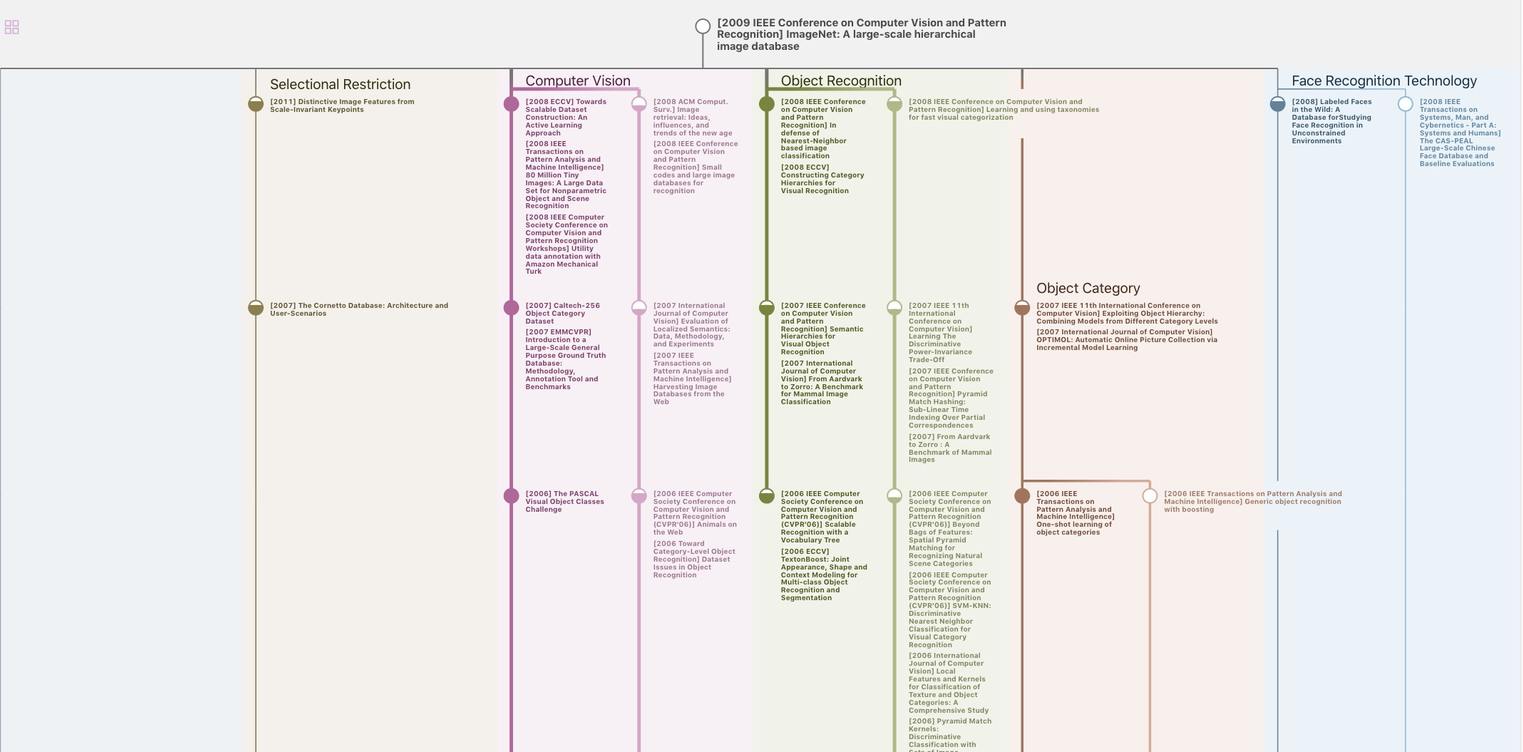
生成溯源树,研究论文发展脉络
Chat Paper
正在生成论文摘要