Semantics-Preserved Distortion for Personal Privacy Protection in Information Management
arxiv(2022)
摘要
In recent years, machine learning - particularly deep learning - has
significantly impacted the field of information management. While several
strategies have been proposed to restrict models from learning and memorizing
sensitive information from raw texts, this paper suggests a more
linguistically-grounded approach to distort texts while maintaining semantic
integrity. To this end, we leverage Neighboring Distribution Divergence, a
novel metric to assess the preservation of semantic meaning during distortion.
Building on this metric, we present two distinct frameworks for
semantic-preserving distortion: a generative approach and a substitutive
approach. Our evaluations across various tasks, including named entity
recognition, constituency parsing, and machine reading comprehension, affirm
the plausibility and efficacy of our distortion technique in personal privacy
protection. We also test our method against attribute attacks in three
privacy-focused assignments within the NLP domain, and the findings underscore
the simplicity and efficacy of our data-based improvement approach over
structural improvement approaches. Moreover, we explore privacy protection in a
specific medical information management scenario, showing our method
effectively limits sensitive data memorization, underscoring its practicality.
更多查看译文
AI 理解论文
溯源树
样例
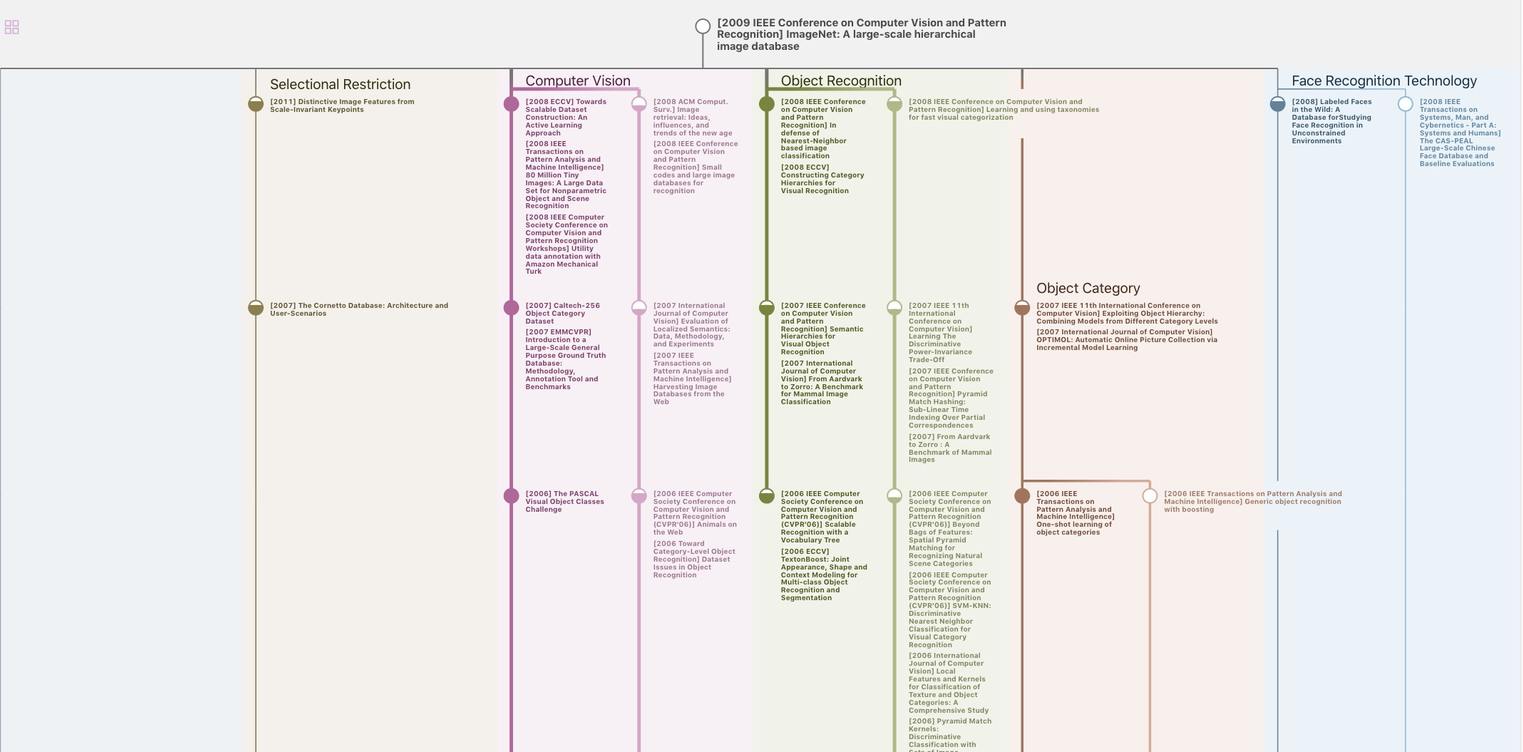
生成溯源树,研究论文发展脉络
Chat Paper
正在生成论文摘要