Machine learning denoising of high-resolution X-ray nanotomography data
JOURNAL OF SYNCHROTRON RADIATION(2022)
摘要
High-resolution X-ray nanotomography is a quantitative tool for investigating specimens from a wide range of research areas. However, the quality of the reconstructed tomogram is often obscured by noise and therefore not suitable for automatic segmentation. Filtering methods are often required for a detailed quantitative analysis. However, most filters induce blurring in the reconstructed tomograms. Here, machine learning (ML) techniques offer a powerful alternative to conventional filtering methods. In this article, we verify that a self-supervised denoising ML technique can be used in a very efficient way for eliminating noise from nanotomography data. The technique presented is applied to high-resolution nanotomography data and compared to conventional filters, such as a median filter and a nonlocal means filter, optimized for tomographic data sets. The ML approach proves to be a very powerful tool that outperforms conventional filters by eliminating noise without blurring relevant structural features, thus enabling efficient quantitative analysis in different scientific fields.
更多查看译文
关键词
nanotomography, full-field X-ray microscopy, Zernike phase contrast, machine learning, denoising
AI 理解论文
溯源树
样例
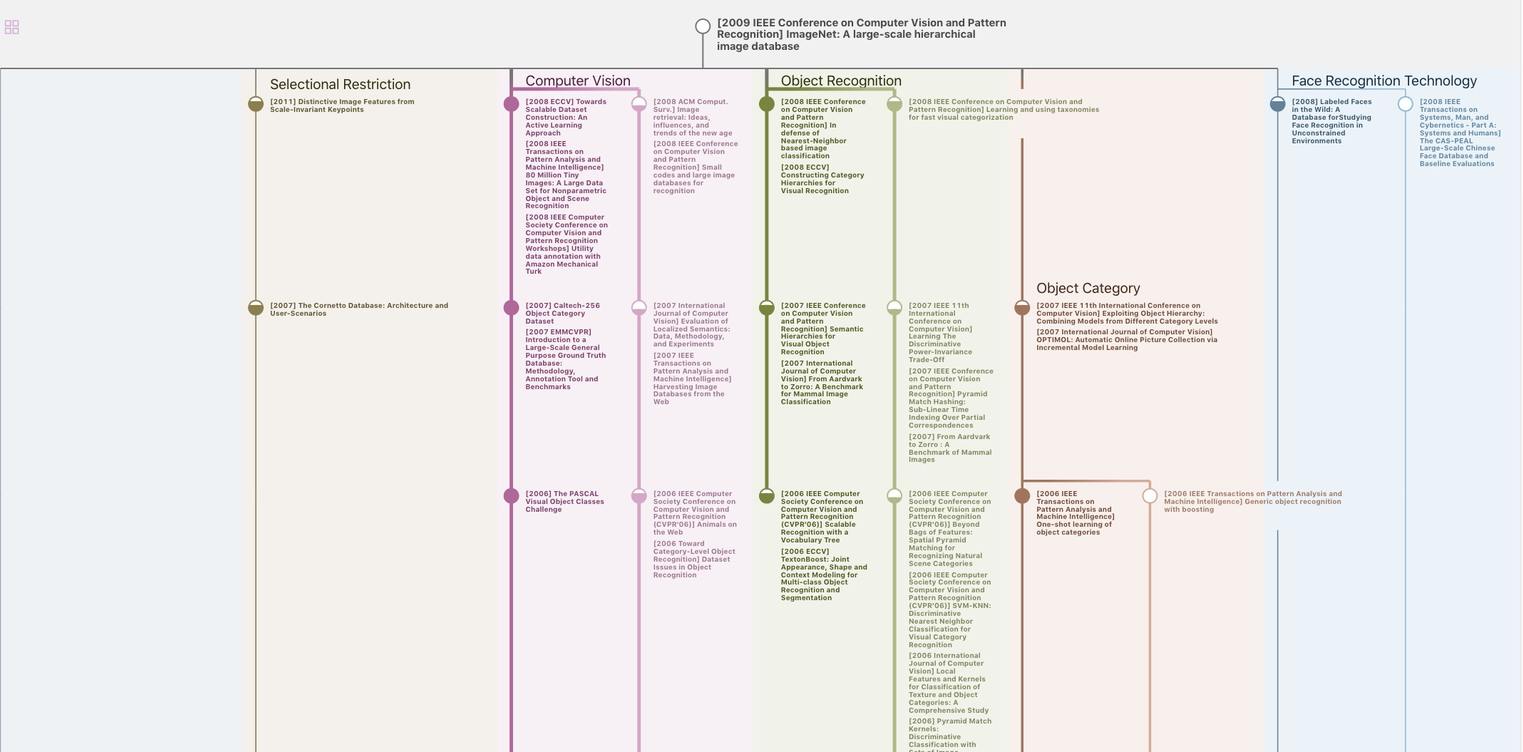
生成溯源树,研究论文发展脉络
Chat Paper
正在生成论文摘要