Graph Neural Networks for Double-Strand DNA Breaks Prediction
arxiv(2022)
摘要
Double-strand DNA breaks (DSBs) are a form of DNA damage that can cause abnormal chromosomal rearrangements. Recent technologies based on high-throughput experiments have obvious high costs and technical challenges.Therefore, we design a graph neural network based method to predict DSBs (GraphDSB), using DNA sequence features and chromosome structure information. In order to improve the expression ability of the model, we introduce Jumping Knowledge architecture and several effective structural encoding methods. The contribution of structural information to the prediction of DSBs is verified by the experiments on datasets from normal human epidermal keratinocytes (NHEK) and chronic myeloid leukemia cell line (K562), and the ablation studies further demonstrate the effectiveness of the designed components in the proposed GraphDSB framework. Finally, we use GNNExplainer to analyze the contribution of node features and topology to DSBs prediction, and proved the high contribution of 5-mer DNA sequence features and two chromatin interaction modes.
更多查看译文
关键词
dna,graph,neural networks,prediction,double-strand
AI 理解论文
溯源树
样例
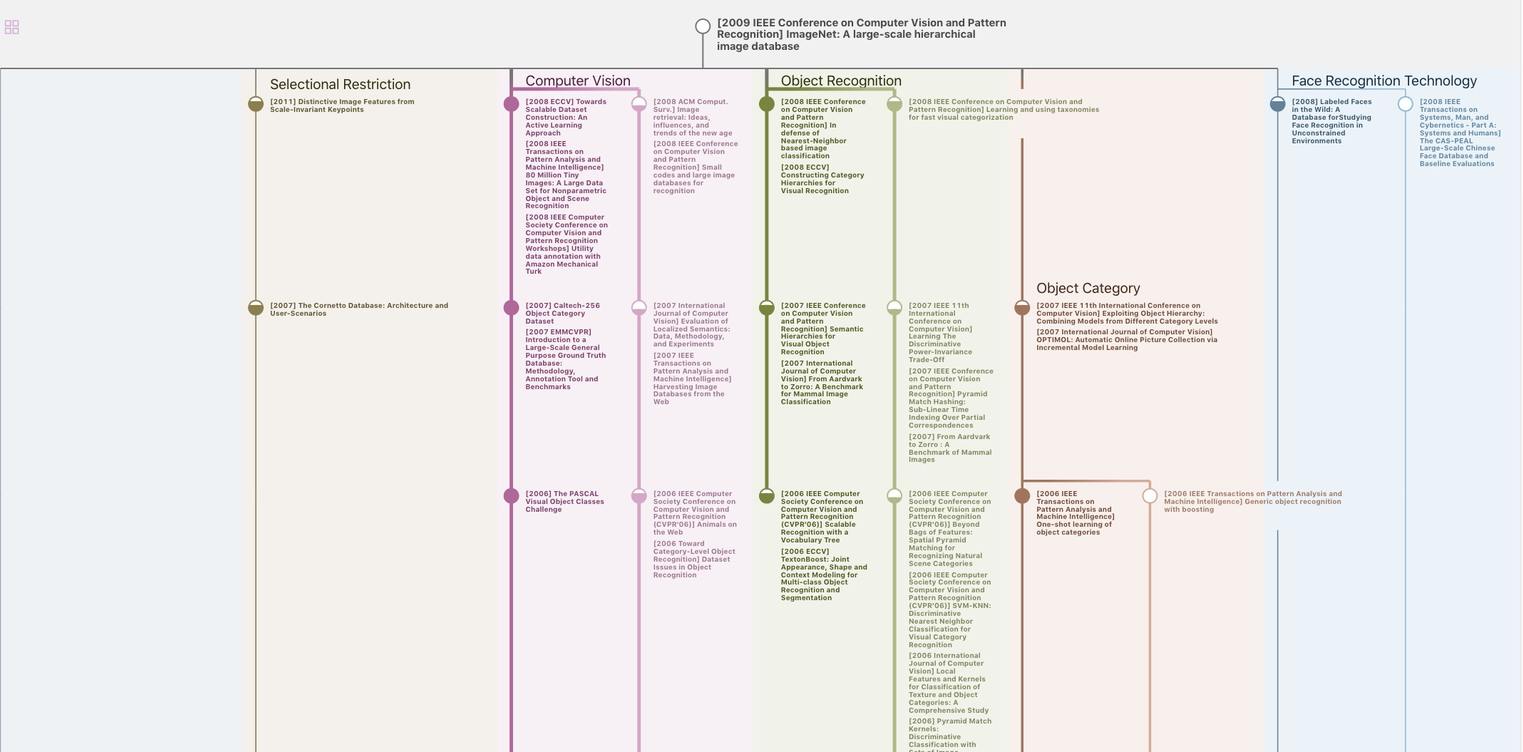
生成溯源树,研究论文发展脉络
Chat Paper
正在生成论文摘要