Interpretable CNN for Ischemic Stroke Subtype Classification with Active Model Adaptation.
BMC medical informatics and decision making(2022)
摘要
BACKGROUND:TOAST subtype classification is important for diagnosis and research of ischemic stroke. Limited by experience of neurologist and time-consuming manual adjudication, it is a big challenge to finish TOAST classification effectively. We propose a novel active deep learning architecture to classify TOAST.METHODS:To simulate the diagnosis process of neurologists, we drop the valueless features by XGB algorithm and rank the remaining ones. Utilizing active learning framework, we propose a novel causal CNN, in which it combines with a mixed active selection criterion to optimize the uncertainty of samples adaptively. Meanwhile, KL-focal loss derived from the enhancement of Focal loss by KL regularization is introduced to accelerate the iterative fine-tuning of the model.RESULTS:To evaluate the proposed method, we construct a dataset which consists of totally 2310 patients. In a series of sequential experiments, we verify the effectiveness of each contribution by different evaluation metrics. Experimental results show that the proposed method achieves competitive results on each evaluation metric. In this task, the improvement of AUC is the most obvious, reaching 77.4.CONCLUSIONS:We construct a backbone causal CNN to simulate the neurologist process of that could enhance the internal interpretability. The research on clinical data also indicates the potential application value of this model in stroke medicine. Future work we would consider various data types and more comprehensive patient types to achieve fully automated subtype classification.
更多查看译文
关键词
Interpretability,Ischemic Stroke,Active learning,Classification algorithm,Loss function
AI 理解论文
溯源树
样例
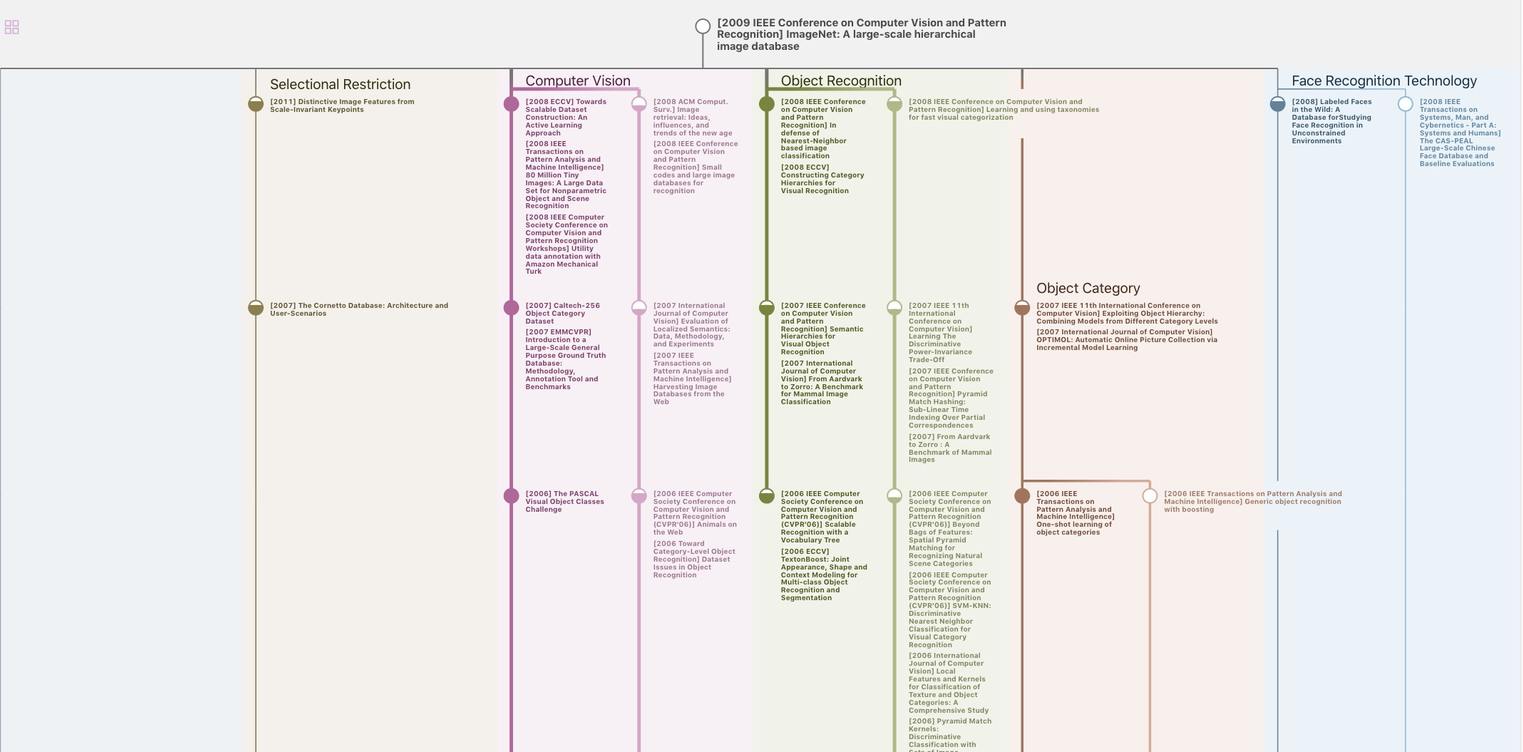
生成溯源树,研究论文发展脉络
Chat Paper
正在生成论文摘要