Improving Peer Assessment Accuracy by Incorporating Grading Behaviors
2021 IEEE 33RD INTERNATIONAL CONFERENCE ON TOOLS WITH ARTIFICIAL INTELLIGENCE (ICTAI 2021)(2021)
摘要
Peer assessment, which asks students to evaluate their peers' submissions, has become the mainstream paradigm for solving the massive grading challenge of open-ended assignments faced by teachers at MOOC platforms. Since peer grades may be biased and unreliable, a group of probabilistic graph models are proposed to improve the estimation to the true scores of assignments derived based on peer grades, by explicitly modeling the bias and reliability of each grader. However, these models assume that graders' reliability are only impacted by their knowledge/ability levels while ignoring their grading behaviors. In real life, graders' grading behaviors (e.g., the time consumed for reviewing an assignment) reflect the seriousness of the graders in the assessment and greatly affect their reliability. Following this intuition, we propose two novel probabilistic graph models for cardinal peer assessment, which optimizes the modeling of the reliability of graders by incorporating various grading behaviors of them. In specific, a GBDT-based regressor is firstly built to quantify the grading seriousness of graders according to their behaviors. Second, the grading seriousness values together with knowledge/ability levels of graders are both employed to model their reliability. Finally, an algorithm based on Gibbs sampling is designed to infer true scores of assignments according to the models. Experimental results on a real peer assessment dataset show the superiority of the proposed models in improving the estimation accuracy to the true scores of assignments by leveraging grader grading behaviors.
更多查看译文
关键词
peer assessment, probabilistic graph models, GBDT, online education
AI 理解论文
溯源树
样例
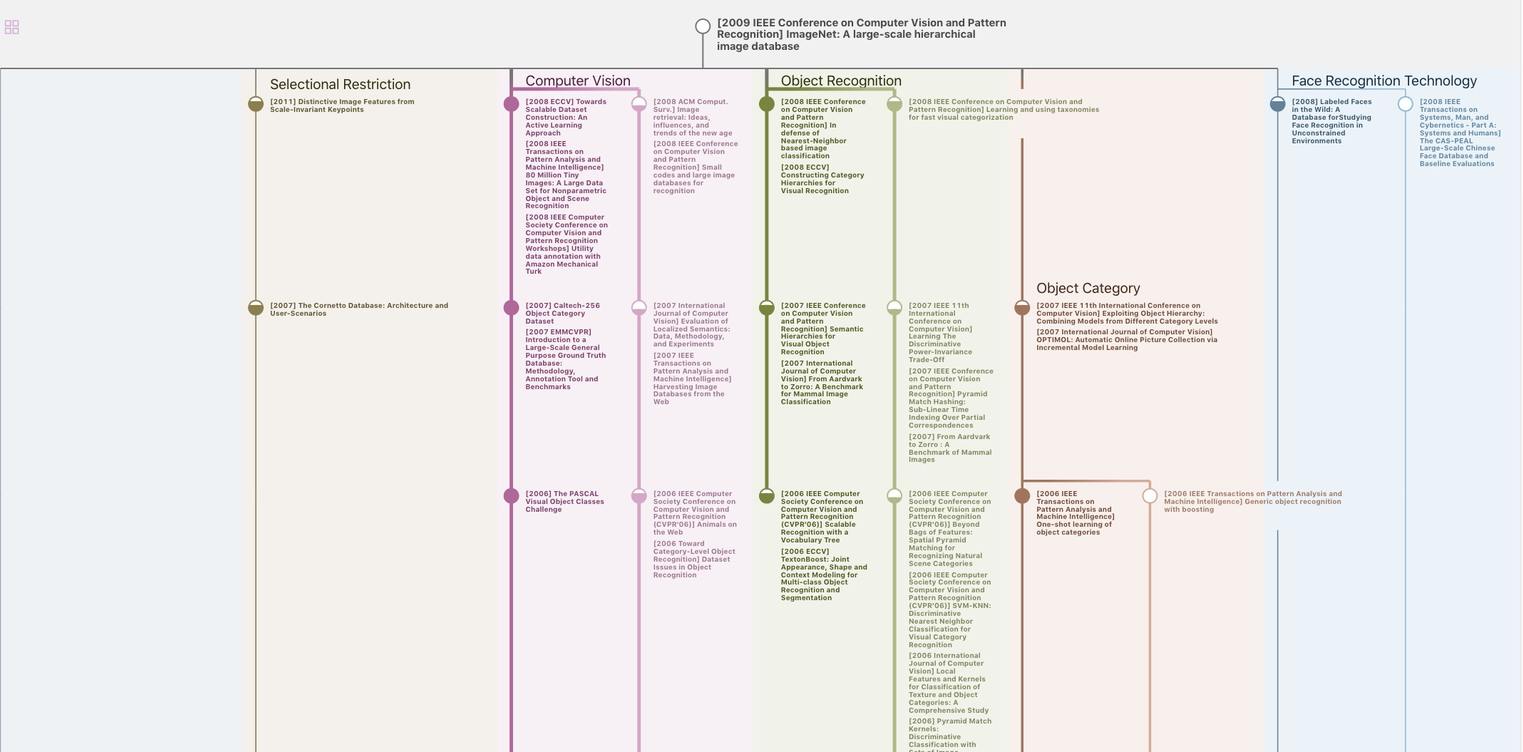
生成溯源树,研究论文发展脉络
Chat Paper
正在生成论文摘要