Hierarchical Multilabel Text Classification via Multitask Learning
2021 IEEE 33RD INTERNATIONAL CONFERENCE ON TOOLS WITH ARTIFICIAL INTELLIGENCE (ICTAI 2021)(2021)
摘要
Hierarchical multilabel classification is a variant of classification where instances might belong to multiple labels and these labels come from a hierarchy. In this paper, we solve the hierarchical multilabel text classification problem of professionally-generated content via multitask learning. More specifically, we focus on (1) how to build models that can share features well in multitask learning, (2) how to incorporate the label dependence into the training procedure of the models, and (3) how to combine the predicted labels of different levels in the hierarchy. To make the experiments simple and comparable, we bring in the state-of-art BERT model as the base model in our work. Experiment results show that the multitask models we build are competitive, the penalty loss we propose is able to improve the performance, and the union operation is the best choice to handle prediction contradiction. In other words, the time cost is reduced but performance is improved via our multitask learning approach.
更多查看译文
关键词
multilabel classification, hierarchical multilabel classification, multitask learning, text classification
AI 理解论文
溯源树
样例
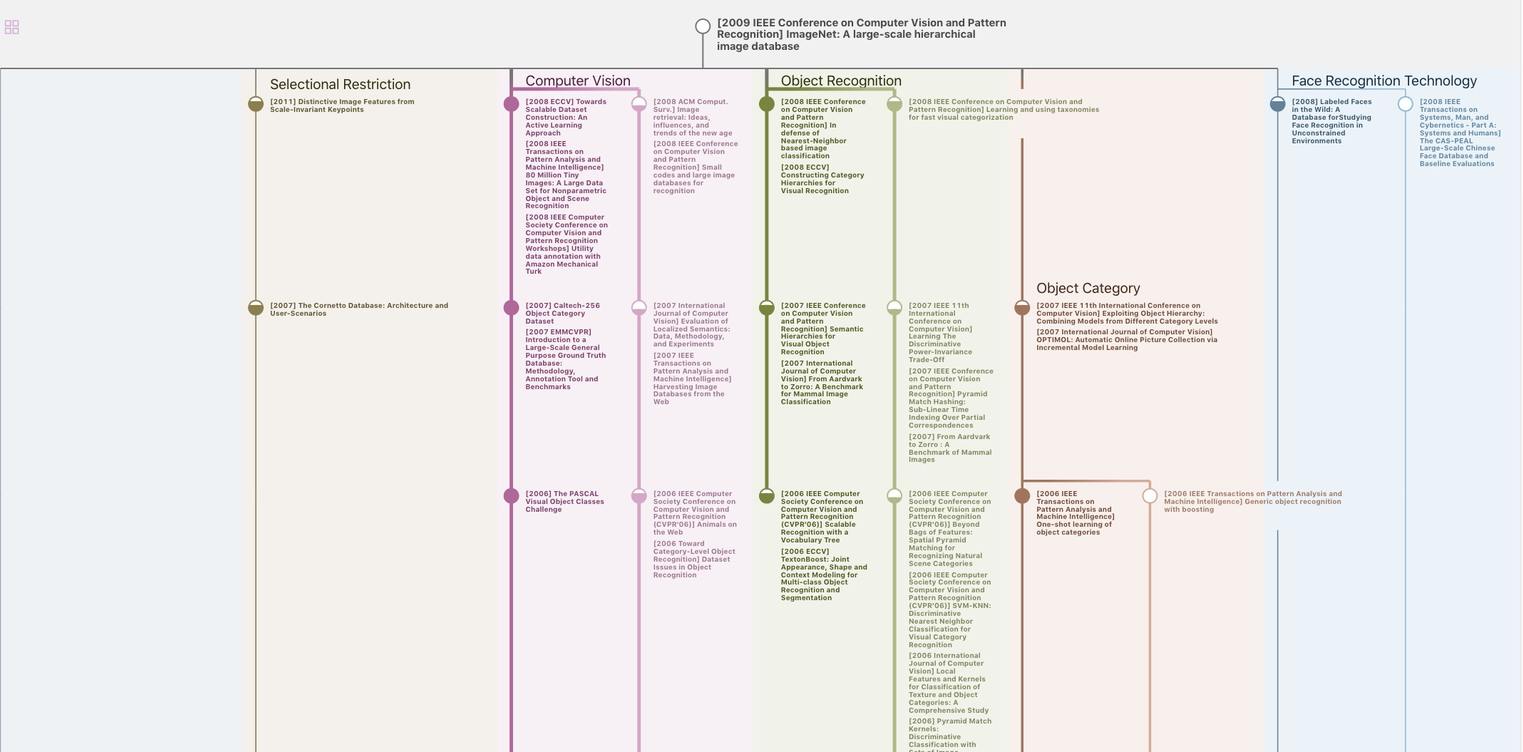
生成溯源树,研究论文发展脉络
Chat Paper
正在生成论文摘要