Uncertainty analysis method of slope safety factor based on quantile-based ensemble learning
BULLETIN OF ENGINEERING GEOLOGY AND THE ENVIRONMENT(2023)
摘要
To overcome the problem that the point prediction method of slope safety factor has uncertainty in its prediction and hence cannot a reliable slope safety factor, a quantile-based ensemble learning regression method is proposed that quantifies the uncertainty of point prediction models by predicting the value interval of the slope safety factor. Firstly, the slope safety factor and its influencing factors are preprocessed to obtain an effective dataset. Secondly, with minimizing the quantile loss as the model optimization goal, three commonly used ensemble learning models (Random Forest, LightGBM, and CatBoost) are optimized by grid search and nested 10-fold cross-validation to obtain three quantile-based ensemble learning models (QRF, QLightGBM, and QCatBoost) that can directly construct prediction intervals. Finally, the corrected predictive interval accuracy (CPIA) index is introduced to compare the prediction performance of the different models under different quantile conditions. The results show that the three proposed models can eliminate the influence of the uncertainty of the traditional point prediction model. Among them, the point prediction performance of the models is 7.9%, 4.1%, and 0.5% higher than that of the traditional model in terms of R 2 , and CPIA is 0.713, 0.729, and 0.703, respectively. The proposed method can provide an effective value interval for the prediction of slope safety factors and provide a new idea for the uncertainty analysis of safety factors.
更多查看译文
关键词
Interval prediction,Slope safety factor,Quantile loss,Nested cross-validation,Ensemble learning
AI 理解论文
溯源树
样例
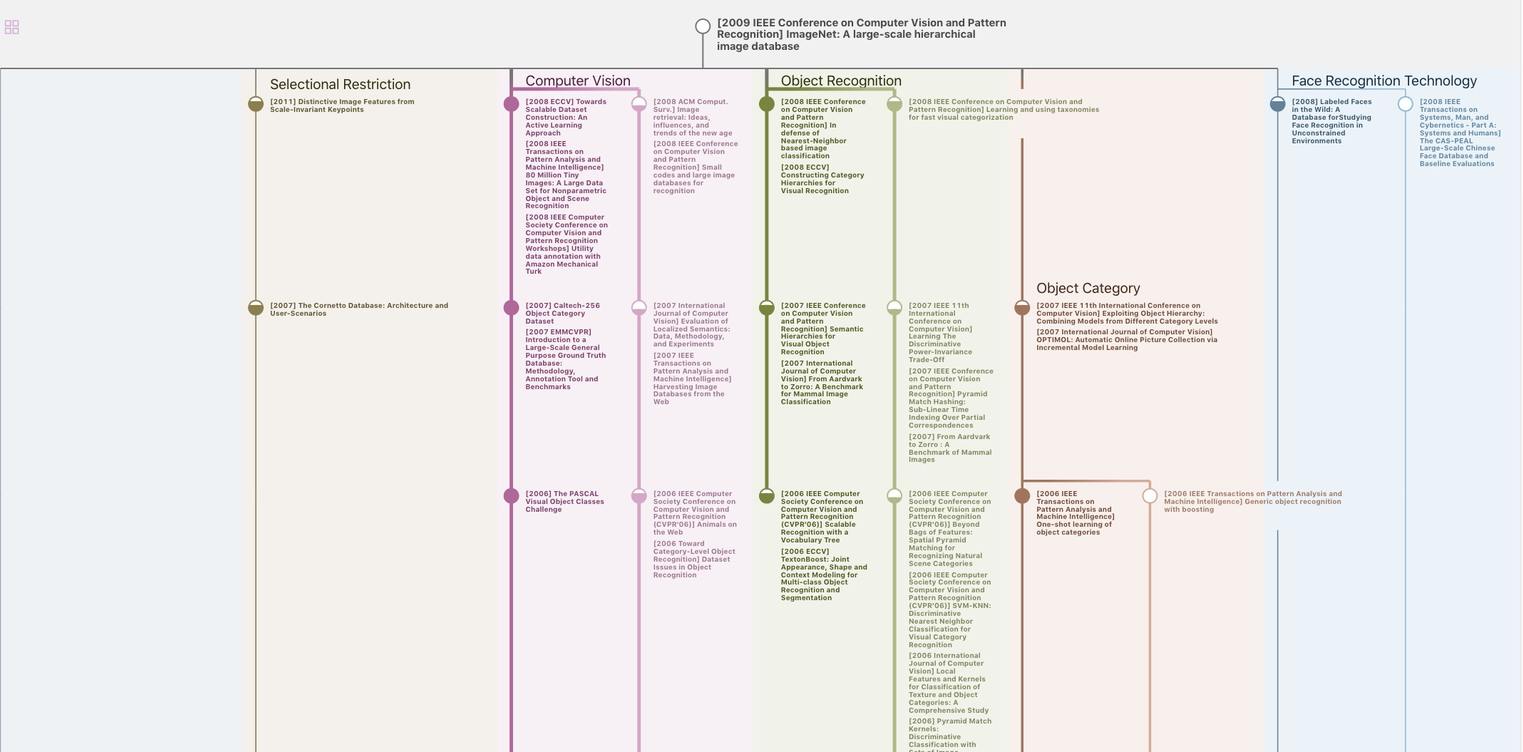
生成溯源树,研究论文发展脉络
Chat Paper
正在生成论文摘要