Sub-mW Keyword Spotting on an MCU: Analog Binary Feature Extraction and Binary Neural Networks
IEEE Transactions on Circuits and Systems I: Regular Papers(2022)
摘要
Keyword spotting (KWS) is a crucial function enabling the interaction with the many ubiquitous smart devices in our surroundings, either activating them through wake-word or directly as a human-computer interface. For many applications, KWS is the entry point for our interactions with the device and, thus, an always-on workload. Many smart devices are mobile and their battery lifetime is heavily impacted by continuously running services. KWS and similar always-on services are thus the focus when optimizing the overall power consumption. This work addresses KWS energy-efficiency on low-cost microcontroller units (MCUs). We combine analog binary feature extraction with binary neural networks. By replacing the digital preprocessing with the proposed analog front-end, we show that the energy required for data acquisition and preprocessing can be reduced by
$29\times $
, cutting its share from a dominating 85% to a mere 16% of the overall energy consumption for our reference KWS application. Experimental evaluations on the Speech Commands Dataset show that the proposed system outperforms state-of-the-art accuracy and energy efficiency, respectively, by 1% and
$4.3\times $
on a 10-class dataset while providing a compelling accuracy-energy trade-off including a 2% accuracy drop for a
$71\times $
energy reduction.
更多查看译文
关键词
Keyword spotting,quantization,binary neural networks,deep learning,feature extraction
AI 理解论文
溯源树
样例
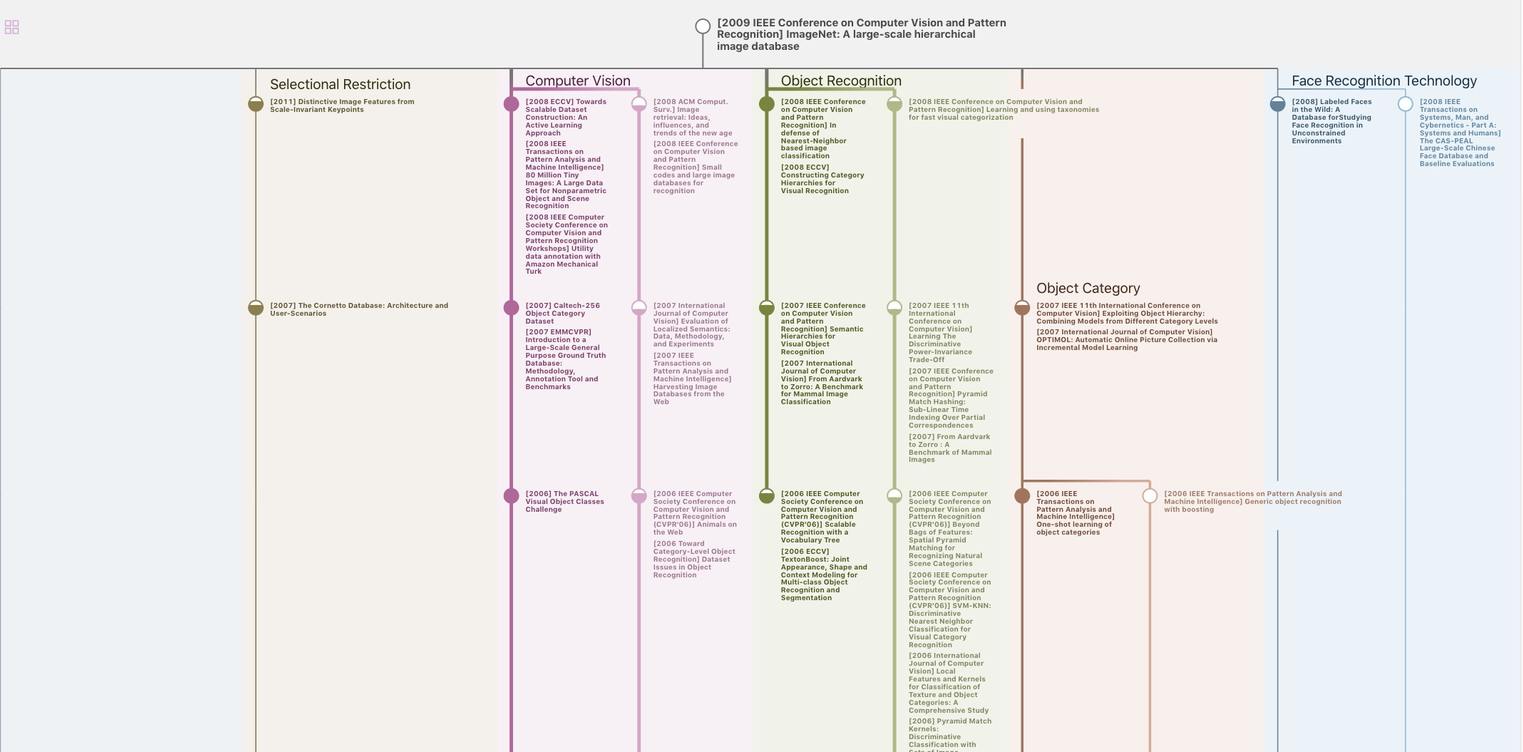
生成溯源树,研究论文发展脉络
Chat Paper
正在生成论文摘要