Robust graphical lasso based on multivariate Winsorization
arxiv(2022)
摘要
We propose the use of a robust covariance estimator based on multivariate Winsorization in the context of the Tarr-Muller-Weber framework for sparse estimation of the precision matrix of a Gaussian graphical model. Likewise Croux-Ollerer's precision matrix estimator, our proposed estimator attains the maximum finite sample breakdown point of 0.5 under cellwise contamination. We conduct an extensive Monte Carlo simulation study to assess the performance of ours and the currently existing proposals. We find that ours has a competitive behavior, regarding the the estimation of the precision matrix and the recovery of the graph. We demonstrate the usefulness of the proposed methodology in a real application to breast cancer data.
更多查看译文
关键词
robust graphical lasso
AI 理解论文
溯源树
样例
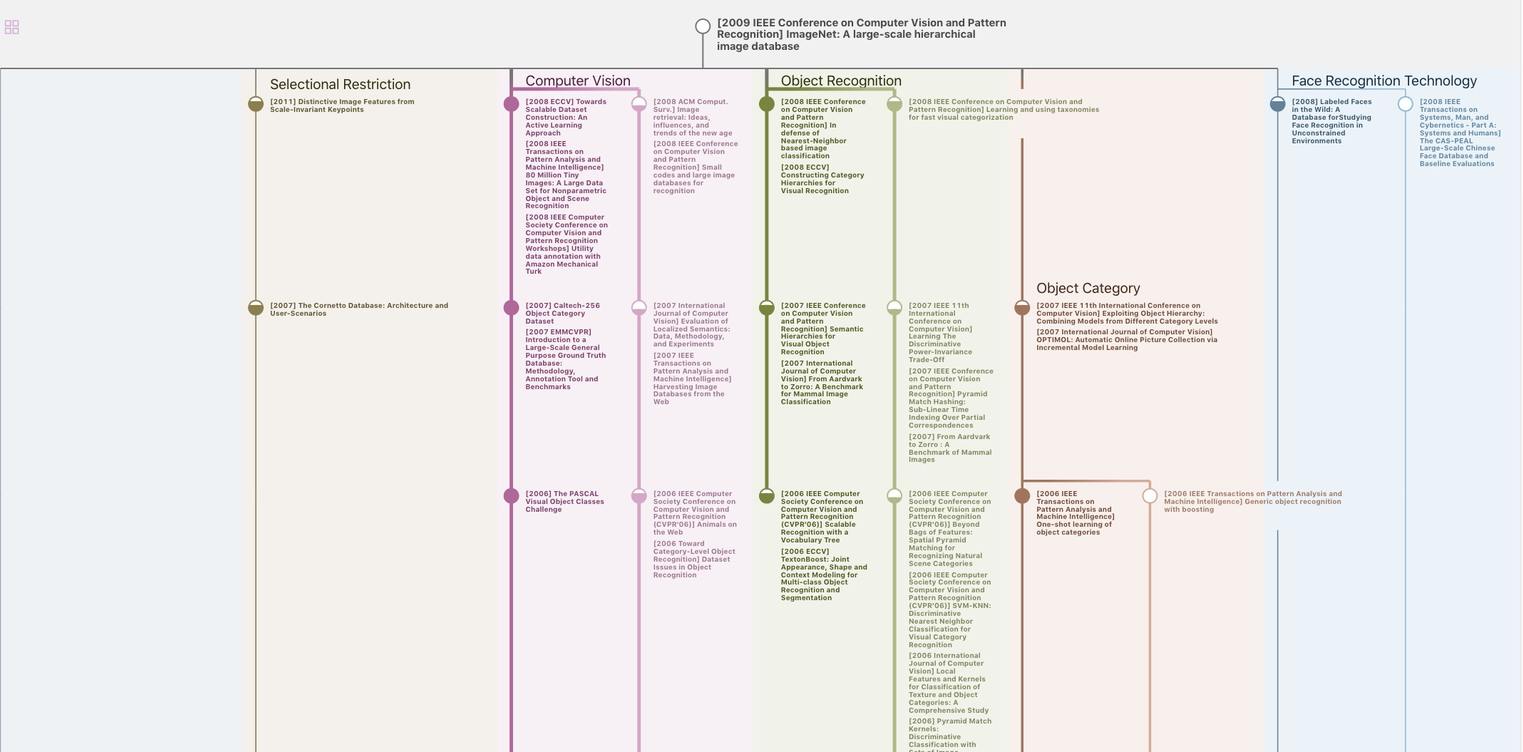
生成溯源树,研究论文发展脉络
Chat Paper
正在生成论文摘要