A Multi-time-scale Time Series Analysis for Click Fraud Forecasting using Binary Labeled Imbalanced Dataset
2019 4th International Conference on Computational Systems and Information Technology for Sustainable Solution (CSITSS)(2019)
Abstract
Click fraud refers to the practice of generating random clicks on a link in order to extract illegitimate revenue from the advertisers. We present a generalized model for modeling temporal click fraud data in the form of probability or learning based anomaly detection and time series modeling with time scales like minutes and hours. The proposed approach consists of seven stages: Pre-processing, data smoothing, fraudulent pattern identification, homogenizing variance, normalizing auto-correlation, developing the AR and MA models and fine tuning along with evaluation of the models. The objective of the proposed work is to first, model multi-time-scale time series data on AR/MA by relying only on time and the label without the need of too many attributes and secondly, to model different time scales separately on Auto-regression (AR) and Moving Average (MA) models. Then, we evaluate the models by tuning forecasting errors and also by minimizing Akaike Information Criteria (AIC) and Bayesian Information Criteria (BIC) to obtain a best fit model for all time scale data. Through our experiments we also demonstrated that the Probability based model approach is better as compared to the Learning based probabilistic estimator model.
MoreTranslated text
Key words
Time Series Analysis,Click Fraud,Binary labeled,Imbalanced Dataset,Auto-regression,Moving Average,Akaike Information Criteria,Bayesian Information Criteria,Data Stationary,homogeneity
AI Read Science
Must-Reading Tree
Example
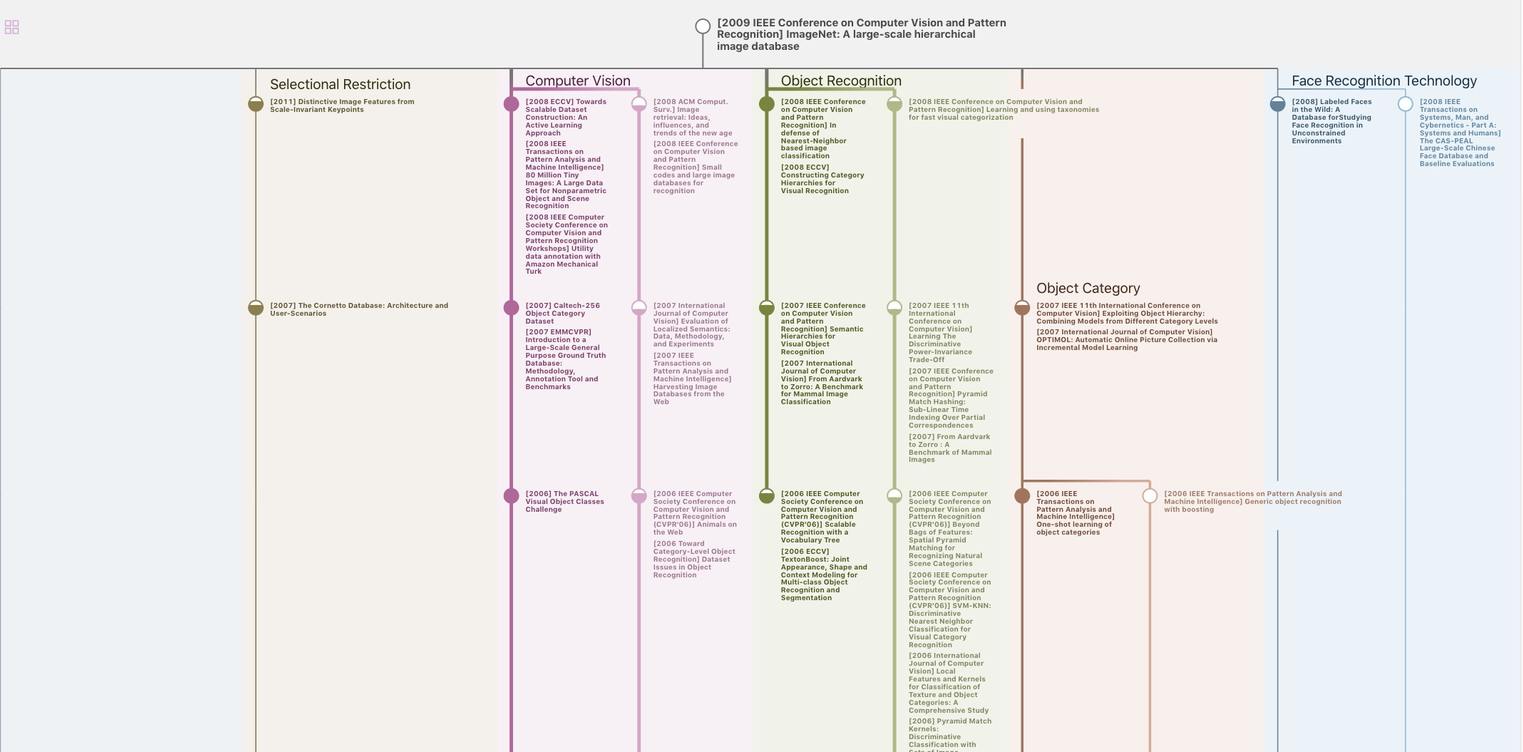
Generate MRT to find the research sequence of this paper
Chat Paper
Summary is being generated by the instructions you defined