Prediction of Optimal Conditions of Hydrogenation Reaction Using the Likelihood Ranking Approach
INTERNATIONAL JOURNAL OF MOLECULAR SCIENCES(2022)
摘要
The selection of experimental conditions leading to a reasonable yield is an important and essential element for the automated development of a synthesis plan and the subsequent synthesis of the target compound. The classical QSPR approach, requiring one-to-one correspondence between chemical structure and a target property, can be used for optimal reaction conditions prediction only on a limited scale when only one condition component (e.g., catalyst or solvent) is considered. However, a particular reaction can proceed under several different conditions. In this paper, we describe the Likelihood Ranking Model representing an artificial neural network that outputs a list of different conditions ranked according to their suitability to a given chemical transformation. Benchmarking calculations demonstrated that our model outperformed some popular approaches to the theoretical assessment of reaction conditions, such as k Nearest Neighbors, and a recurrent artificial neural network performance prediction of condition components (reagents, solvents, catalysts, and temperature). The ability of the Likelihood Ranking model trained on a hydrogenation reactions dataset, (similar to 42,000 reactions) from Reaxys(R) database, to propose conditions that led to the desired product was validated experimentally on a set of three reactions with rich selectivity issues.
更多查看译文
关键词
chemoinformatics, reaction informatics, ranking, artificial neural networks, QSAR, condensed graph of reaction, reaction conditions
AI 理解论文
溯源树
样例
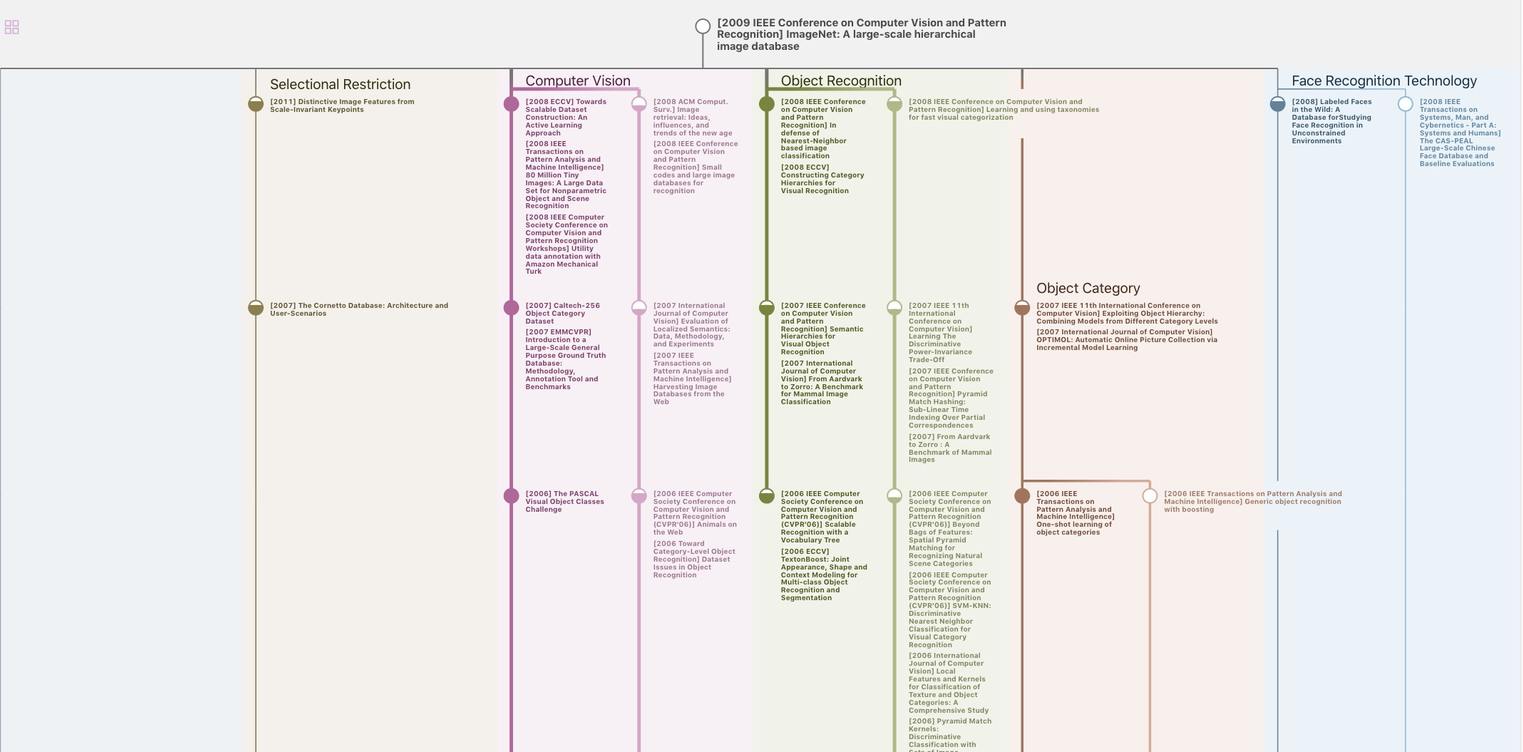
生成溯源树,研究论文发展脉络
Chat Paper
正在生成论文摘要