Localizing Perturbations in Pressurized Water Reactors Using One-Dimensional Deep Convolutional Neural Networks
SENSORS(2022)
摘要
This work outlines an approach for localizing anomalies in nuclear reactor cores during their steady state operation, employing deep, one-dimensional, convolutional neural networks. Anomalies are characterized by the application of perturbation diagnostic techniques, based on the analysis of the so-called "neutron-noise" signals: that is, fluctuations of the neutron flux around the mean value observed in a steady-state power level. The proposed methodology is comprised of three steps: initially, certain reactor core perturbations scenarios are simulated in software, creating the respective perturbation datasets, which are specific to a given reactor geometry; then, the said datasets are used to train deep learning models that learn to identify and locate the given perturbations within the nuclear reactor core; lastly, the models are tested on actual plant measurements. The overall methodology is validated on hexagonal, pre-Konvoi, pressurized water, and VVER-1000 type nuclear reactors. The simulated data are generated by the FEMFFUSION code, which is extended in order to deal with the hexagonal geometry in the time and frequency domains. The examined perturbations are absorbers of variable strength, and the trained models are tested on actual plant data acquired by the in-core detectors of the Temelin VVER-1000 Power Plant in the Czech Republic. The whole approach is realized in the framework of Euratom's CORTEX project.
更多查看译文
关键词
neutron noise, neutron diffusion, deep learning, convolutional neural networks, pressurized water reactor, perturbation localization, VVER-1000, absorber of variable strength, FEMFFUSION
AI 理解论文
溯源树
样例
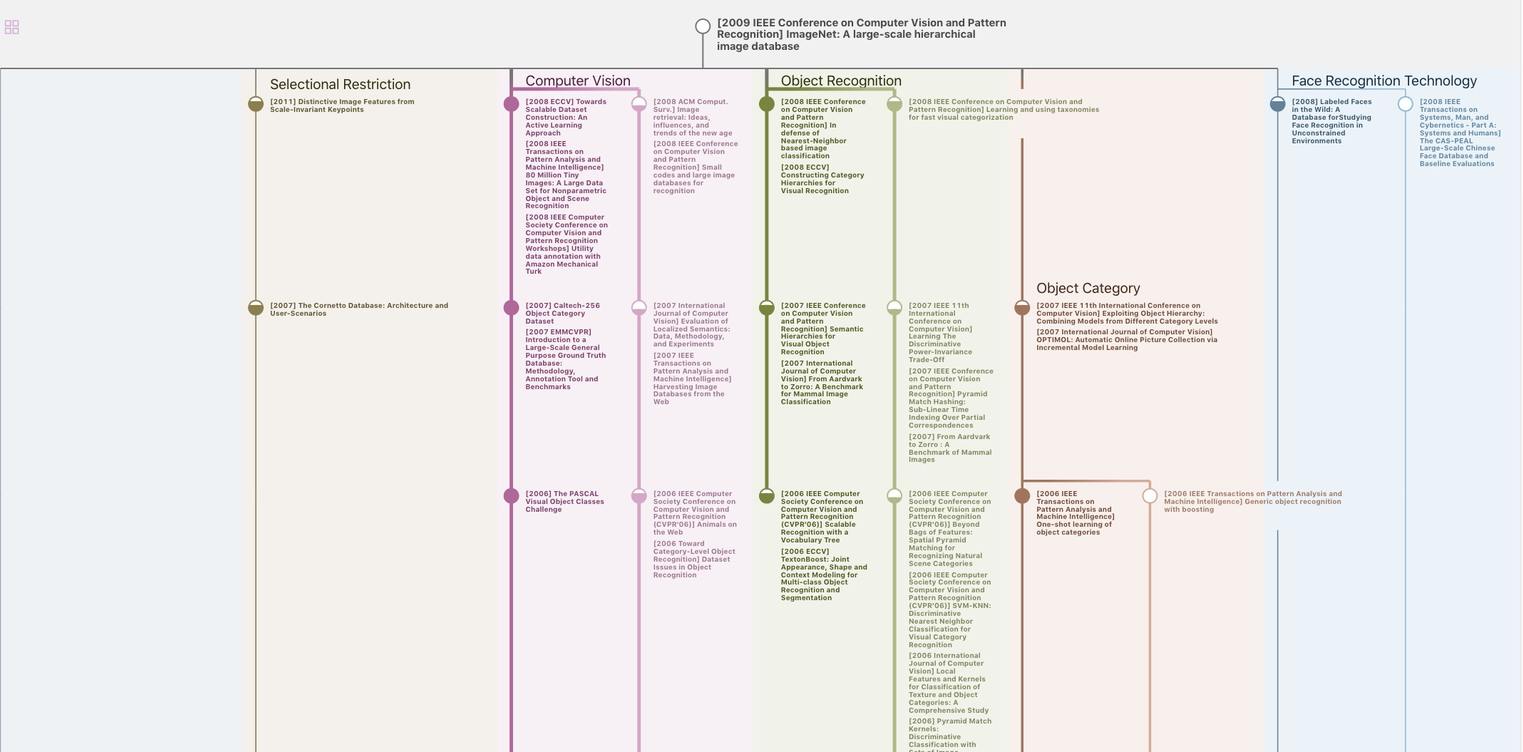
生成溯源树,研究论文发展脉络
Chat Paper
正在生成论文摘要