Attentive and context-aware deep network for saliency prediction on omni-directional images
DIGITAL SIGNAL PROCESSING(2022)
摘要
Understanding visual attention of observers on omni-directional images gains interest along with the booming trend of virtual reality applications. In this paper, we propose a novel attentive and context-aware network for saliency prediction on omni-directional images, which is named as ACSalNet. In this architecture, considering the problem of insufficient receptive fields of high-level features, a Deformable Attention Bottleneck (DAB) is first proposed to strengthen the high-level feature extractor and effectively focus the limited receptive field of the model to the key areas. Then, to reduce the semantic gap between features of different levels and introduce context-aware information, we further design a Context-aware Feature Pyramid Module (CFPM). In the testing phase, in order to reduce the error of prediction directly on the equirectangular images while retaining their integrity, a novel projection method called Multiple Sphere Rotation (MSR) is proposed. Extensive experiments illustrate that the proposed method outperforms the state-of-the-art models under different evaluation metrics on the public saliency benchmarks. (C) 2021 Elsevier Inc. All rights reserved.
更多查看译文
关键词
Omni-directional image, Saliency prediction, ACSalNet, Virtual reality
AI 理解论文
溯源树
样例
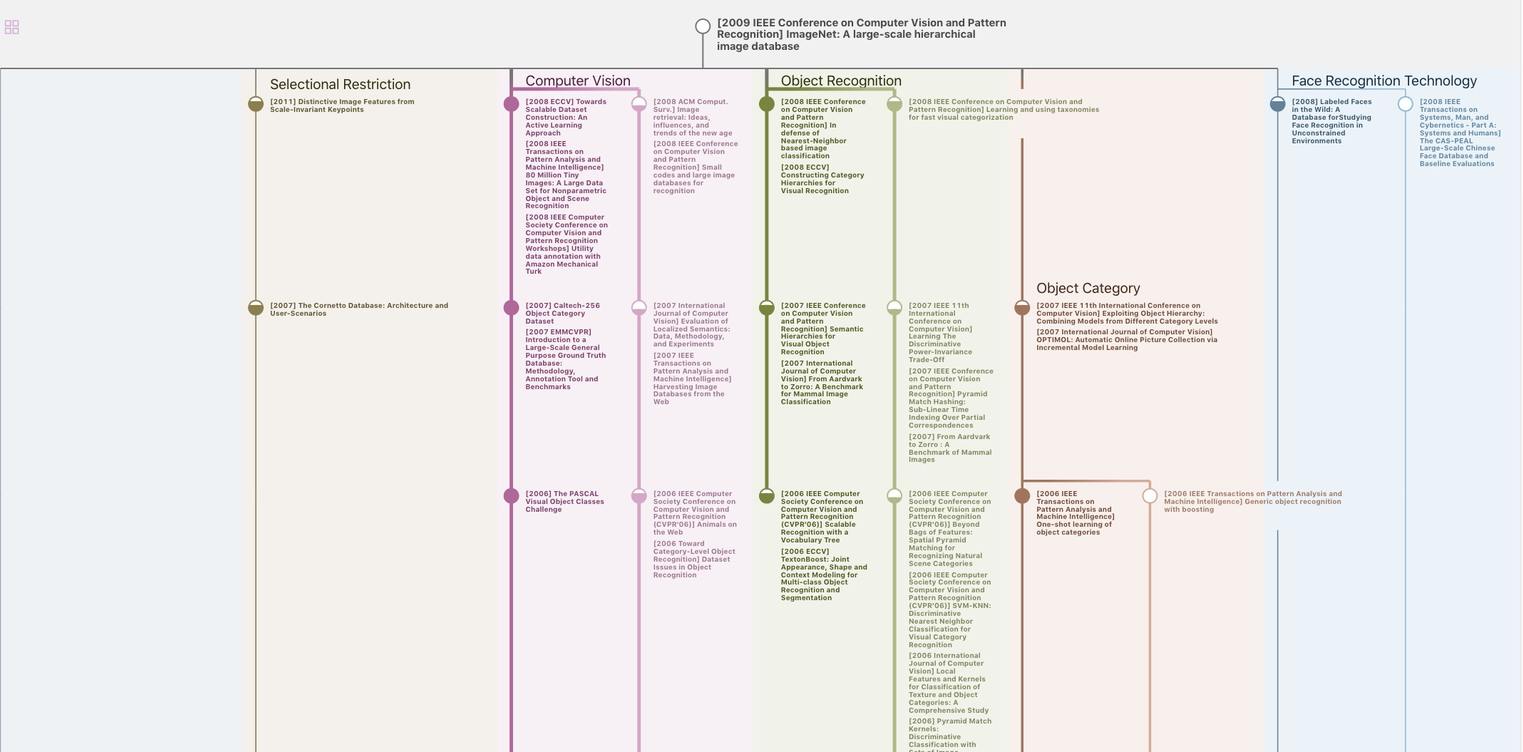
生成溯源树,研究论文发展脉络
Chat Paper
正在生成论文摘要