Distributed Model Predictive Control based on Dual Decomposition with Neural-Network-based Warm Start
2021 EUROPEAN CONTROL CONFERENCE (ECC)(2021)
摘要
This work deals with the application of neural networks to speed up the convergence of a distributed model predictive control (DMPC) algorithm based on dual decomposition. While dual decomposition methods are known to converge to the centralized MPC solution, numerous iterations may be required before convergence is attained, thus increasing computation and communication burden. In this paper, a database containing system states and optimal Lagrange multipliers is created offline to train a neural network, which is incorporated into the online operation of the distributed system. Numerical results on an input-coupled 16 tanks benchmark are provided.
更多查看译文
关键词
distributed model predictive control,dual decomposition,centralized MPC solution,distributed system,neural-network-based warm start,DMPC,system states,optimal Lagrange multipliers,neural network training,convergence,iterations
AI 理解论文
溯源树
样例
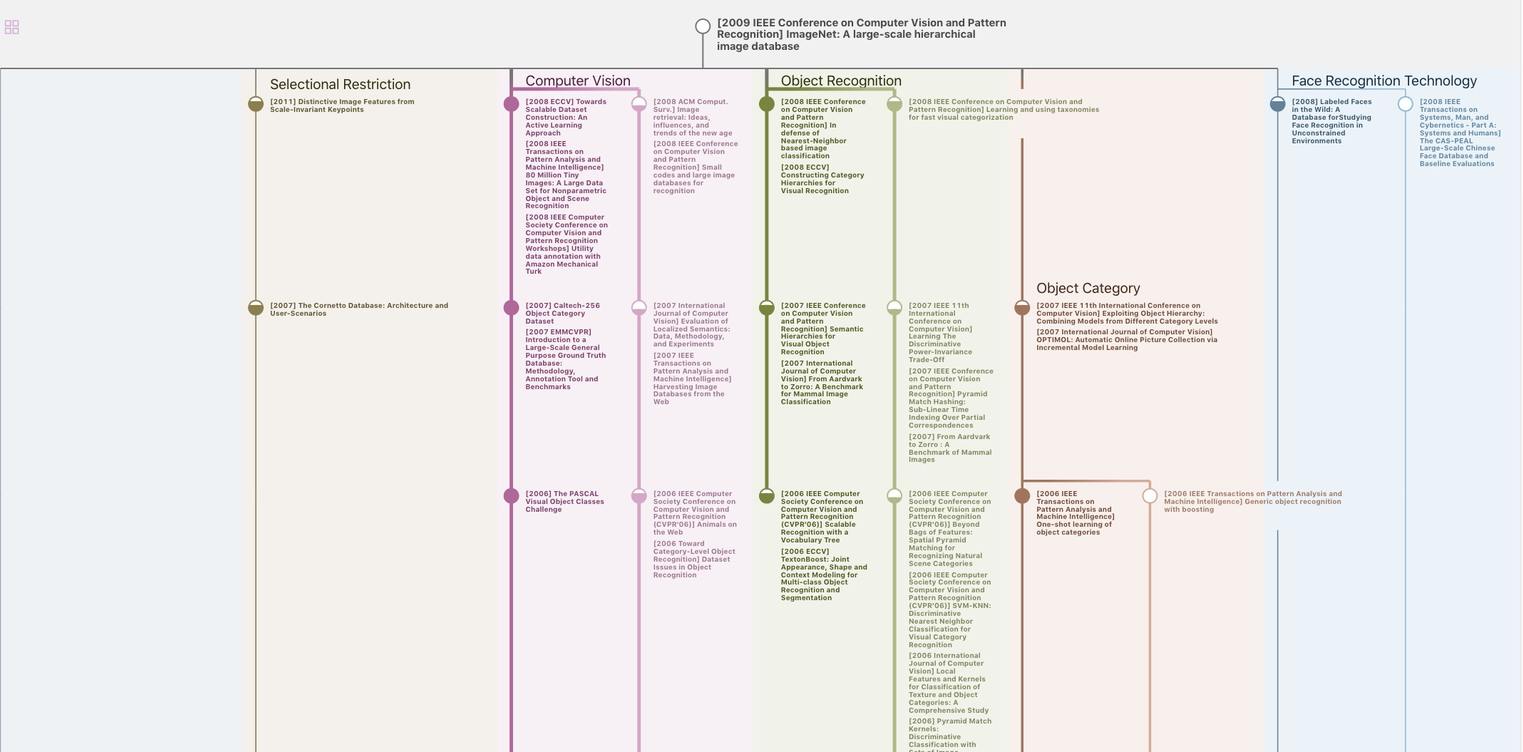
生成溯源树,研究论文发展脉络
Chat Paper
正在生成论文摘要