Benchmarking Deep Reinforcement Learning Algorithms for Vision-based Robotics
ArXiv(2022)
摘要
This paper presents a benchmarking study of some of the state-of-the-art reinforcement learning algorithms used for solving two simulated vision-based robotics problems. The algorithms considered in this study include soft actor-critic (SAC), proximal policy optimization (PPO), interpolated policy gradients (IPG), and their variants with Hindsight Experience replay (HER). The performances of these algorithms are compared against PyBullet's two simulation environments known as KukaDiverseObjectEnv and RacecarZEDGymEnv respectively. The state observations in these environments are available in the form of RGB images and the action space is continuous, making them difficult to solve. A number of strategies are suggested to provide intermediate hindsight goals required for implementing HER algorithm on these problems which are essentially single-goal environments. In addition, a number of feature extraction architectures are proposed to incorporate spatial and temporal attention in the learning process. Through rigorous simulation experiments, the improvement achieved with these components are established. To the best of our knowledge, such a benchmarking study is not available for the above two vision-based robotics problems making it a novel contribution in the field.
更多查看译文
关键词
deep reinforcement learning,deep reinforcement learning algorithms,benchmarking,vision-based
AI 理解论文
溯源树
样例
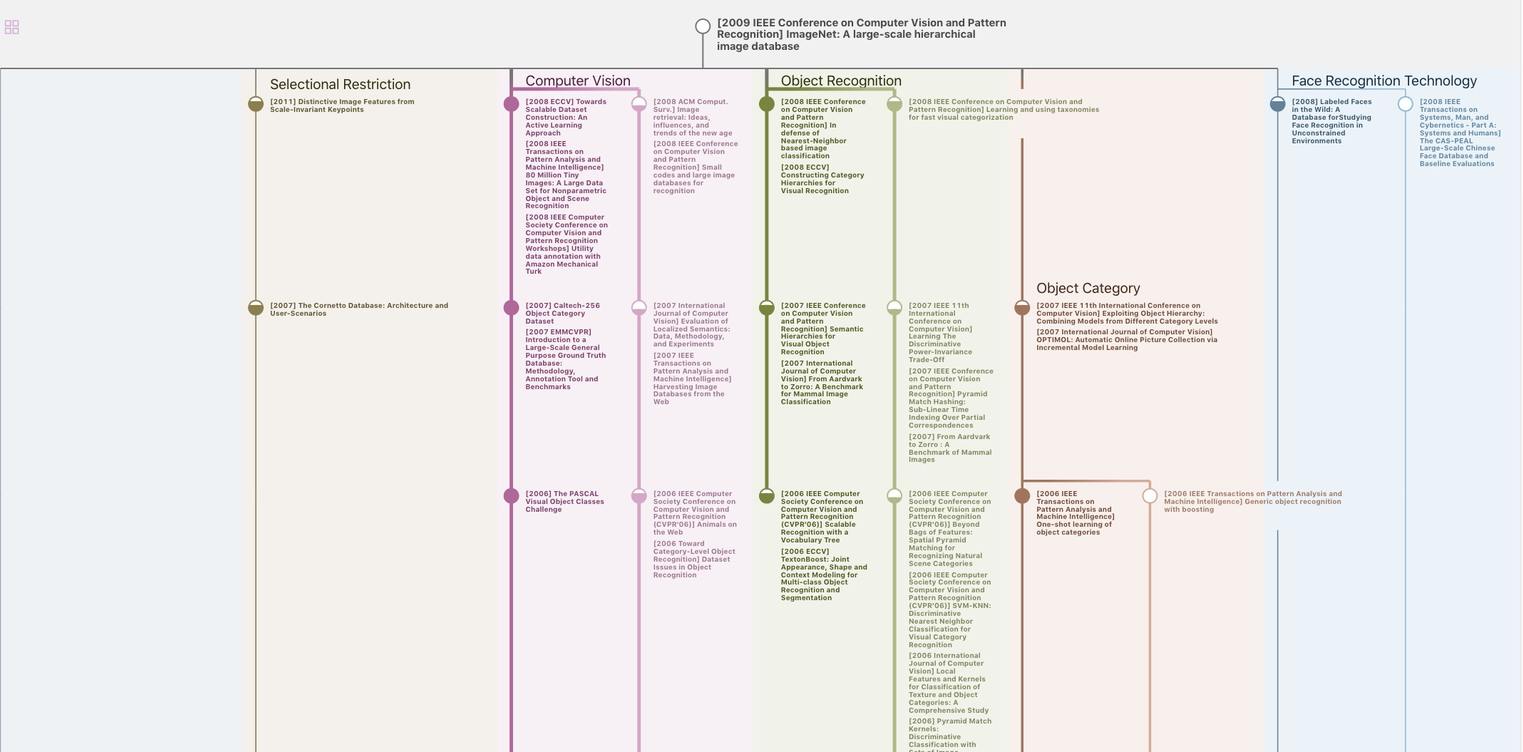
生成溯源树,研究论文发展脉络
Chat Paper
正在生成论文摘要