Forecast-based Multi-aspect Framework for Multivariate Time-series Anomaly Detection
IEEE BigData(2021)
摘要
Today’s cyber-world is vastly multivariate. Metrics collected at extreme varieties demand multivariate algorithms to properly detect anomalies. However, forecast-based algorithms, as widely proven approaches, often perform sub-optimally or inconsistently across datasets. A key common issue is they strive to be one-size-fits-all but anomalies are distinctive in nature. We propose a method that tailors to such distinction. Presenting FMUAD - a Forecast-based, Multi-aspect, Unsupervised Anomaly Detection framework. FMUAD explicitly and separately captures the signature traits of anomaly types - spatial change, temporal change and correlation change - with independent modules. The modules then jointly learn an optimal feature representation, which is highly flexible and intuitive, unlike most other models in the category. Extensive experiments show our FMUAD framework consistently outperforms other state-of-the-art forecast-based anomaly detectors.
更多查看译文
关键词
Anomaly Detection,Multivariate Time Series,Unsupervised Learning
AI 理解论文
溯源树
样例
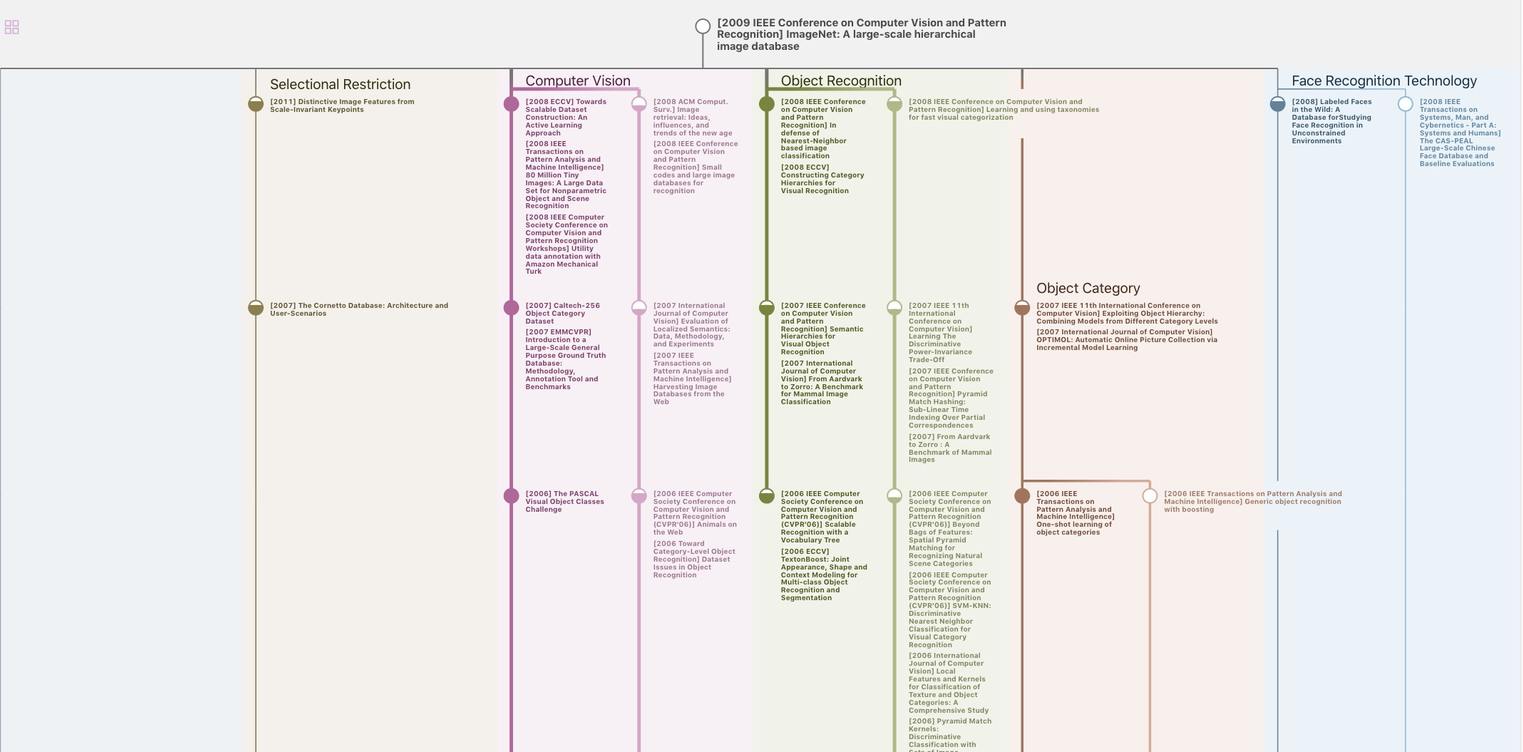
生成溯源树,研究论文发展脉络
Chat Paper
正在生成论文摘要