Automatic brain segmentation in preterm infants with post-hemorrhagic hydrocephalus using 3D Bayesian U-Net
HUMAN BRAIN MAPPING(2022)
摘要
Post-hemorrhagic hydrocephalus (PHH) is a severe complication of intraventricular hemorrhage (IVH) in very preterm infants. PHH monitoring and treatment decisions rely heavily on manual and subjective two-dimensional measurements of the ventricles. Automatic and reliable three-dimensional (3D) measurements of the ventricles may provide a more accurate assessment of PHH, and lead to improved monitoring and treatment decisions. To accurately and efficiently obtain these 3D measurements, automatic segmentation of the ventricles can be explored. However, this segmentation is challenging due to the large ventricular anatomical shape variability in preterm infants diagnosed with PHH. This study aims to (a) propose a Bayesian U-Net method using 3D spatial concrete dropout for automatic brain segmentation (with uncertainty assessment) of preterm infants with PHH; and (b) compare the Bayesian method to three reference methods: DenseNet, U-Net, and ensemble learning using DenseNets and U-Nets. A total of 41 T-2-weighted MRIs from 27 preterm infants were manually segmented into lateral ventricles, external CSF, white and cortical gray matter, brainstem, and cerebellum. These segmentations were used as ground truth for model evaluation. All methods were trained and evaluated using 4-fold cross-validation and segmentation endpoints, with additional uncertainty endpoints for the Bayesian method. In the lateral ventricles, segmentation endpoint values for the DenseNet, U-Net, ensemble learning, and Bayesian U-Net methods were mean Dice score = 0.814 +/- 0.213, 0.944 +/- 0.041, 0.942 +/- 0.042, and 0.948 +/- 0.034 respectively. Uncertainty endpoint values for the Bayesian U-Net were mean recall = 0.953 +/- 0.037, mean negative predictive value = 0.998 +/- 0.005, mean accuracy = 0.906 +/- 0.032, and mean AUC = 0.949 +/- 0.031. To conclude, the Bayesian U-Net showed the best segmentation results across all methods and provided accurate uncertainty maps. This method may be used in clinical practice for automatic brain segmentation of preterm infants with PHH, and lead to better PHH monitoring and more informed treatment decisions.
更多查看译文
关键词
automatic brain segmentation, Bayesian deep learning, Monte Carlo dropout, post-hemorrhagic hydrocephalus, preterm infants, uncertainty assessment
AI 理解论文
溯源树
样例
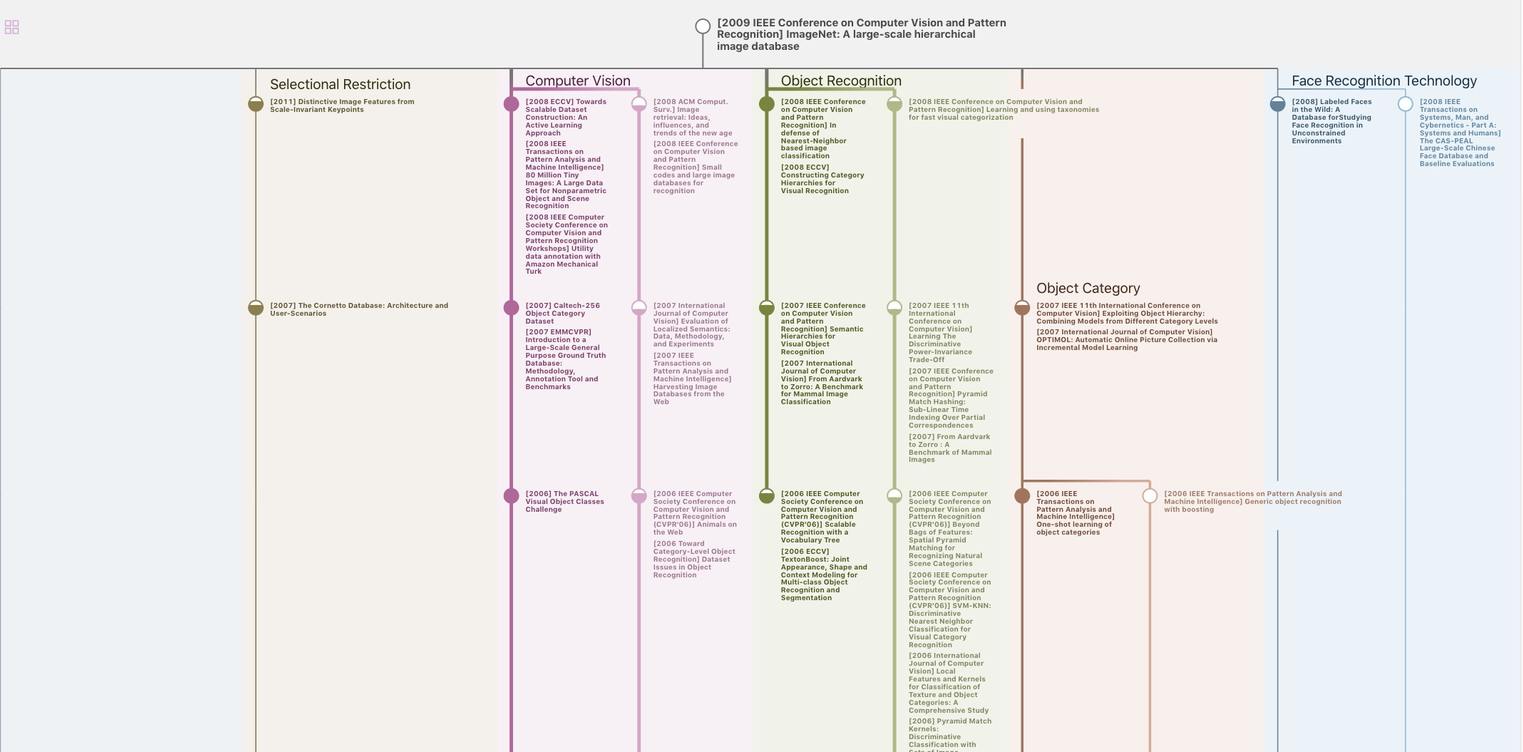
生成溯源树,研究论文发展脉络
Chat Paper
正在生成论文摘要