Principal component analysis denoising improves sensitivity of MR diffusion to detect white matter injury in neuroHIV
JOURNAL OF NEUROIMAGING(2022)
摘要
Background and Purpose Diffusion-weighted imaging is able to capture important information about cerebral white matter (WM) structure. However, diffusion data can suffer from MRI and biological noise that degrades the quality of the images and makes finding important features difficult. We investigated how effectively local and nonlocal denoising increased the sensitivity to detect differences in cerebral WM in neuroHIV. Methods We utilized principal component analysis (PCA) denoising to detect WM differences using fractional anisotropy. Local and nonlocal PCA denoising paradigms were implemented that varied in search area and number of components. We examined different-sized WM tracts that consistently show differences between people living with Human Immunodeficiency Virus (HIV) (PWH) and HIV-negative individuals (corpus callosum, forceps minor, and right uncinate fasciculus), and size-matched tracts not typically associated with HIV-related differences (spinothalamic, right medial lemniscus, and left occipitopontine). We first conducted a full sample comparison of WM differences between groups, and then randomly reduced the sample to the point where we still found differences in WM. Results Nonlocal PCA denoising allowed us to detect differences after a sample reduction of 35% in the forceps minor, 17% in the right uncinate fasciculus, and 6% in the corpus callosum. Conclusions PCA denoising had a beneficial effect on detecting significant differences in PWH after sample size reduction. The smaller forceps minor tract and right uncinate fasciculus showed greater sensitivity to PCA denoising than the larger corpus callosum. These results show the importance of identifying the most effective PCA denoising strategy when investigating WM in PWH.
更多查看译文
关键词
denoising, diffusion tensor imaging, HIV, white matter
AI 理解论文
溯源树
样例
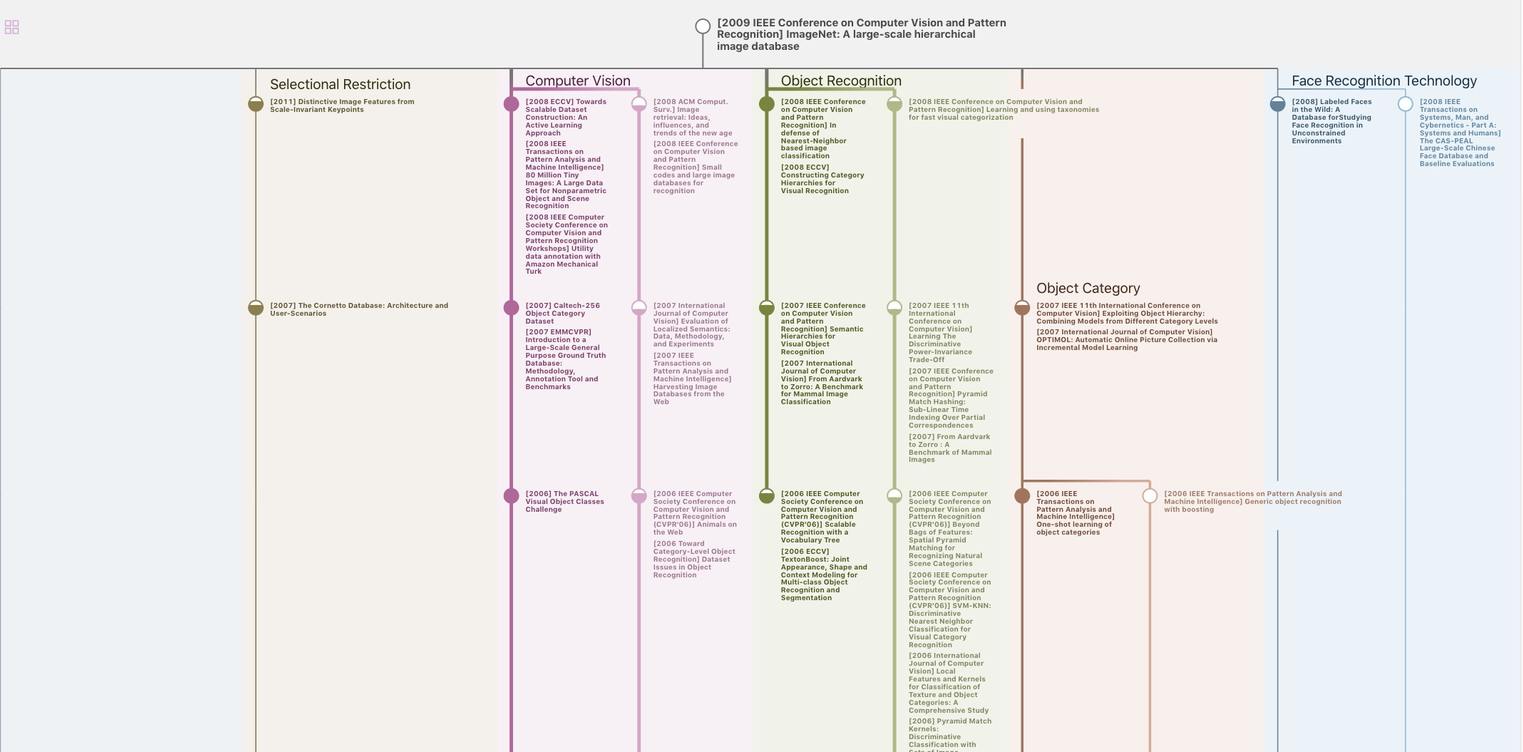
生成溯源树,研究论文发展脉络
Chat Paper
正在生成论文摘要