Automatic detection and segmentation of morphological changes of the maxillary sinus mucosa on cone-beam computed tomography images using a three-dimensional convolutional neural network
Clinical Oral Investigations(2022)
摘要
Objectives To propose and evaluate a convolutional neural network (CNN) algorithm for automatic detection and segmentation of mucosal thickening (MT) and mucosal retention cysts (MRCs) in the maxillary sinus on low-dose and full-dose cone-beam computed tomography (CBCT). Materials and methods A total of 890 maxillary sinuses on 445 CBCT scans were analyzed. The air space, MT, and MRCs in each sinus were manually segmented. Low-dose CBCTs were divided into training, training-monitoring, and testing datasets at a 7:1:2 ratio. Full-dose CBCTs were used as a testing dataset. A three-step CNN algorithm built based on V-Net and support vector regression was trained on low-dose CBCTs and tested on the low-dose and full-dose datasets. Performance for detection of MT and MRCs using area under the curves (AUCs) and for segmentation using Dice similarity coefficient (DSC) was evaluated. Results For the detection of MT and MRCs, the algorithm achieved AUCs of 0.91 and 0.84 on low-dose scans and of 0.89 and 0.93 on full-dose scans, respectively. The median DSCs for segmenting the air space, MT, and MRCs were 0.972, 0.729, and 0.678 on low-dose scans and 0.968, 0.663, and 0.787 on full-dose scans, respectively. There were no significant differences in the algorithm performance between low-dose and full-dose CBCTs. Conclusions The proposed CNN algorithm has the potential to accurately detect and segment MT and MRCs in maxillary sinus on CBCT scans with low-dose and full-dose protocols. Clinical relevance An implementation of this artificial intelligence application in daily practice as an automated diagnostic and reporting system seems possible.
更多查看译文
关键词
Maxillary sinus, Mucosal thickening, Mucosal retention cyst, Artificial intelligence, Convolutional neural network, Cone-beam computed tomography
AI 理解论文
溯源树
样例
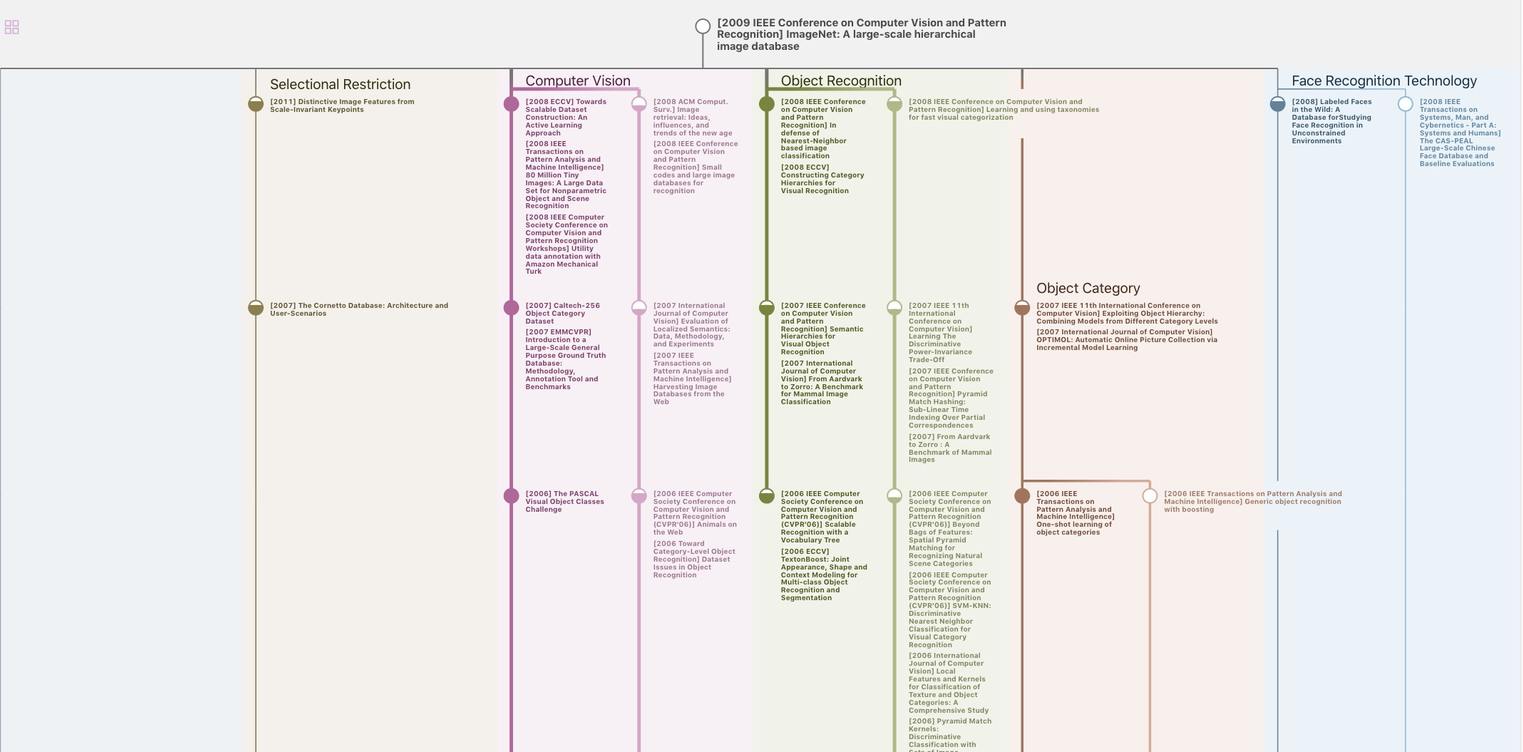
生成溯源树,研究论文发展脉络
Chat Paper
正在生成论文摘要