Bone Suppression on Pediatric Chest Radiographs Via a Deep Learning-Based Cascade Model.
Computer Methods and Programs in Biomedicine(2022)
摘要
Background and objective: Bone suppression images (BSIs) of chest radiographs (CXRs) have been proven to improve diagnosis of pulmonary diseases. To acquire BSIs, dual-energy subtraction (DES) or a deep-learning-based model trained with DES-based BSIs have been used. However, neither technique could be applied to pediatric patients owing to the harmful effects of DES. In this study, we developed a novel method for bone suppression in pediatric CXRs. Methods: First, a model using digitally reconstructed radiographs (DRRs) of adults, which were used to generate pseudo-CXRs from computed tomography images, was developed by training a 2-channel contrastive-unpaired-image-translation network. Second, this model was applied to 129 pediatric DRRs to generate the paired training data of pseudo-pediatric CXRs. Finally, by training a U-Net with these paired data, a bone suppression model for pediatric CXRs was developed. Results: The evaluation metrics were peak signal to noise ratio, root mean absolute error and structural similarity index measure at soft-tissue and bone region of the lung. In addition, an expert radiologist scored the effectiveness of BSIs on a scale of 1-5. The obtained result of 3.31 +/- 0.48 indicates that the BSIs show homogeneous bone removal despite subtle residual bone shadow. Conclusion: Our method shows that the pixel intensity at soft-tissue regions was preserved, and bones were well subtracted; this can be useful for detecting early pulmonary disease in pediatric CXRs. (c) 2022 Elsevier B.V. All rights reserved.
更多查看译文
关键词
Bone suppression,Chest radiograph,Deep learning,Image translation,Pediatric
AI 理解论文
溯源树
样例
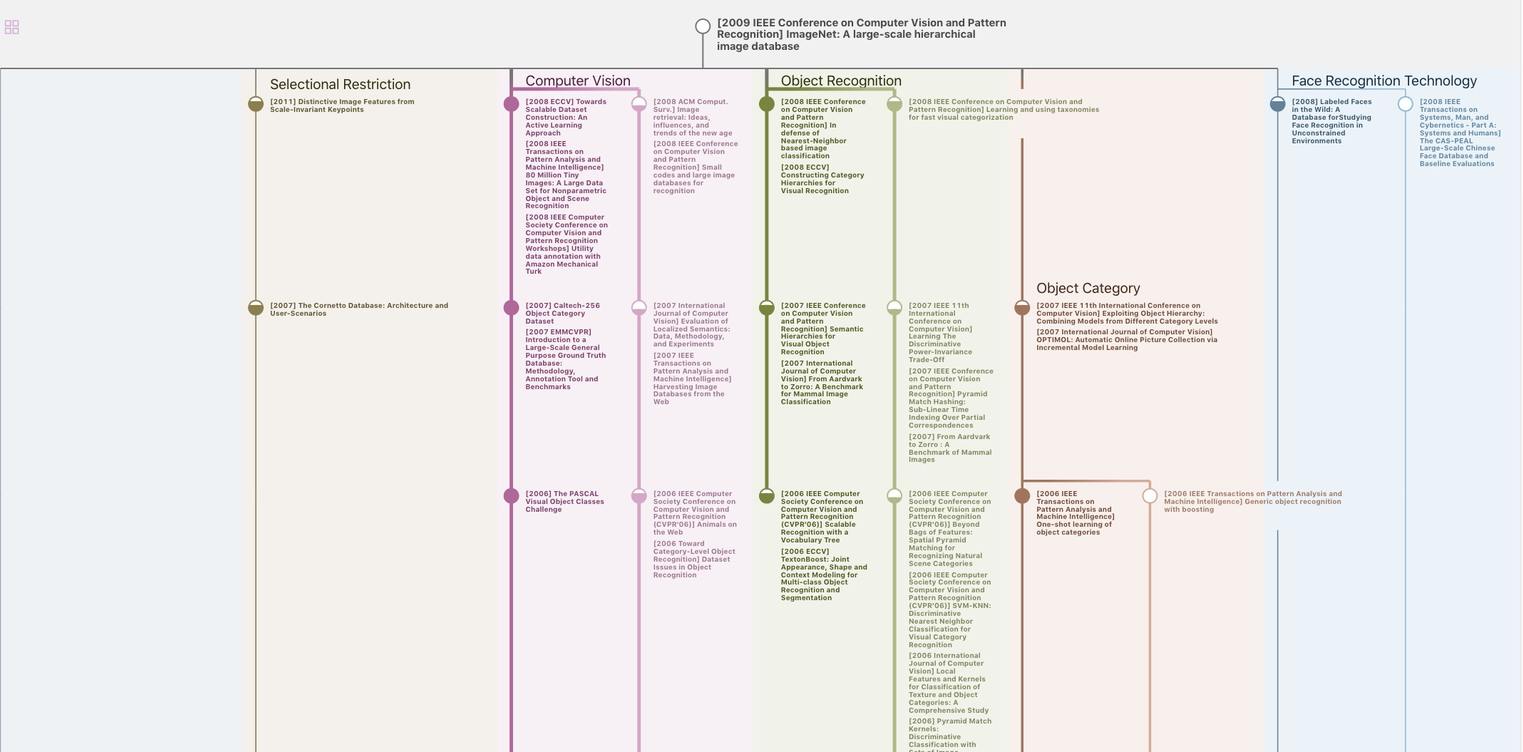
生成溯源树,研究论文发展脉络
Chat Paper
正在生成论文摘要