Understanding the bias in machine learning systems for cardiovascular disease risk assessment: The first of its kind review
COMPUTERS IN BIOLOGY AND MEDICINE(2022)
摘要
Background: Artificial Intelligence (AI), in particular, machine learning (ML) has shown promising results in coronary artery disease (CAD) or cardiovascular disease (CVD) risk prediction. Bias in ML systems is of great interest due to its over-performance and poor clinical delivery. The main objective is to understand the nature of risk-of-bias (RoB) in ML and non-ML studies for CVD risk prediction. Methods: PRISMA model was used to shortlisting 117 studies, which were analyzed to understand the RoB in ML and non-ML using 46 and 32 attributes, respectively. The mean score for each study was computed and then ranked into three ML and non-ML bias categories, namely low-bias (LB), moderate-bias (MB), and high-bias (HB), derived using two cutoffs. Further, bias computation was validated using the analytical slope method. Results: Five types of the gold standard were identified in the ML design for CAD/CVD risk prediction. The low-moderate and moderate-high bias cutoffs for 24 ML studies (5, 10, and 9 studies for each LB, MB, and HB) and 14 non-ML (3, 4, and 7 studies for each LB, MB, and HB) were in the range of 1.5 to 1.95. Bias(ML) < Bias(non-ML )by similar to 43%. A set of recommendations were proposed for lowering RoB. Conclusion: ML showed a lower bias compared to non-ML. For a robust ML-based CAD/CVD prediction design, it is vital to have (i) stronger outcomes like death or CAC score or coronary artery stenosis; (ii) ensuring scientific/ clinical validation; (iii) adaptation of multiethnic groups while practicing unseen AI; (iv) amalgamation of conventional, laboratory, image-based and medication-based biomarkers.
更多查看译文
关键词
Risk prediction, Coronary artery disease, Carotid ultrasound, Artificial intelligence, Bias, Gold standard, And unseen data
AI 理解论文
溯源树
样例
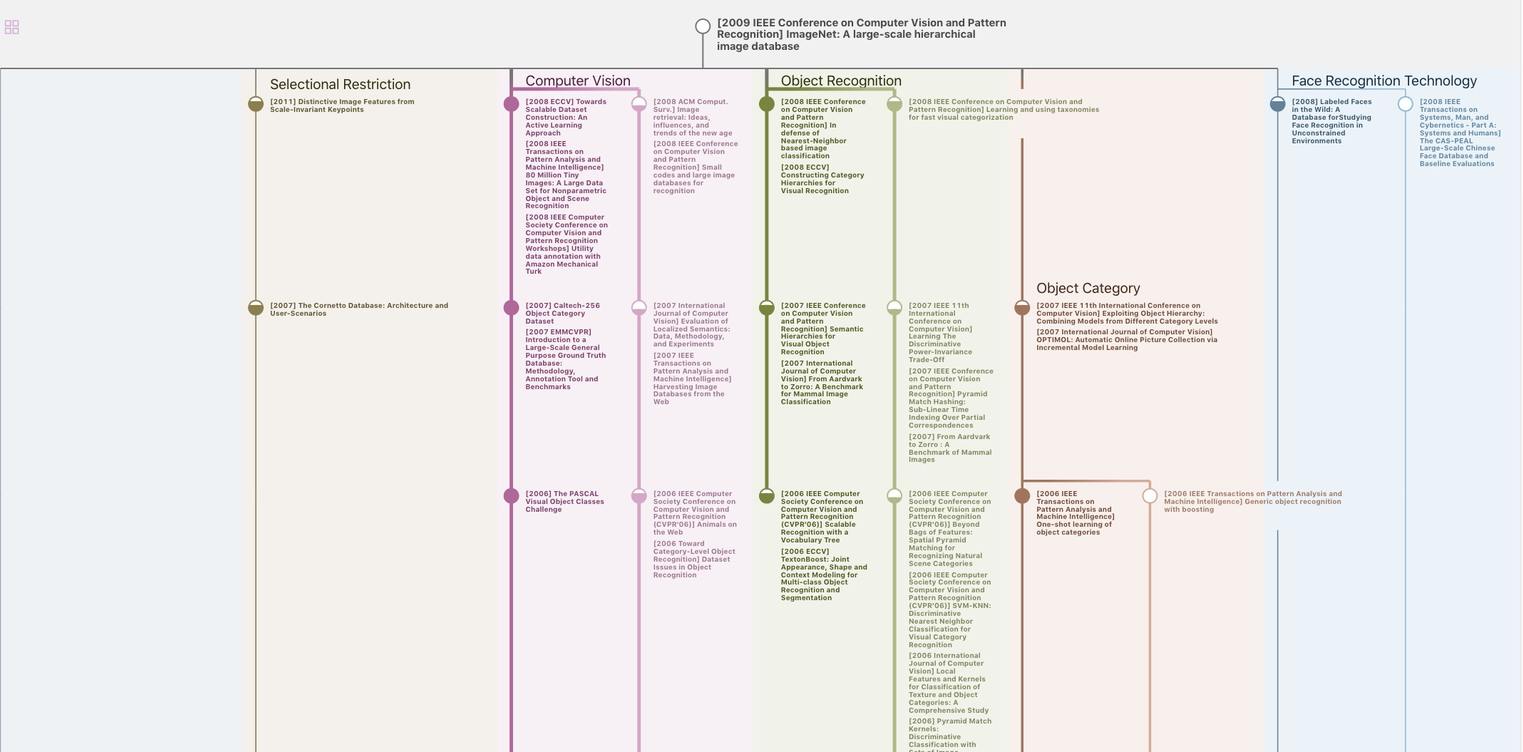
生成溯源树,研究论文发展脉络
Chat Paper
正在生成论文摘要