Reproducible, incremental representation learning with Rosetta VAE
arxiv(2022)
摘要
Variational autoencoders are among the most popular methods for distilling low-dimensional structure from high-dimensional data, making them increasingly valuable as tools for data exploration and scientific discovery. However, unlike typical machine learning problems in which a single model is trained once on a single large dataset, scientific workflows privilege learned features that are reproducible, portable across labs, and capable of incrementally adding new data. Ideally, methods used by different research groups should produce comparable results, even without sharing fully trained models or entire data sets. Here, we address this challenge by introducing the Rosetta VAE (R-VAE), a method of distilling previously learned representations and retraining new models to reproduce and build on prior results. The R-VAE uses post hoc clustering over the latent space of a fully-trained model to identify a small number of Rosetta Points (input, latent pairs) to serve as anchors for training future models. An adjustable hyperparameter, $\rho$, balances fidelity to the previously learned latent space against accommodation of new data. We demonstrate that the R-VAE reconstructs data as well as the VAE and $\beta$-VAE, outperforms both methods in recovery of a target latent space in a sequential training setting, and dramatically increases consistency of the learned representation across training runs.
更多查看译文
关键词
incremental representation,rosetta vae,learning
AI 理解论文
溯源树
样例
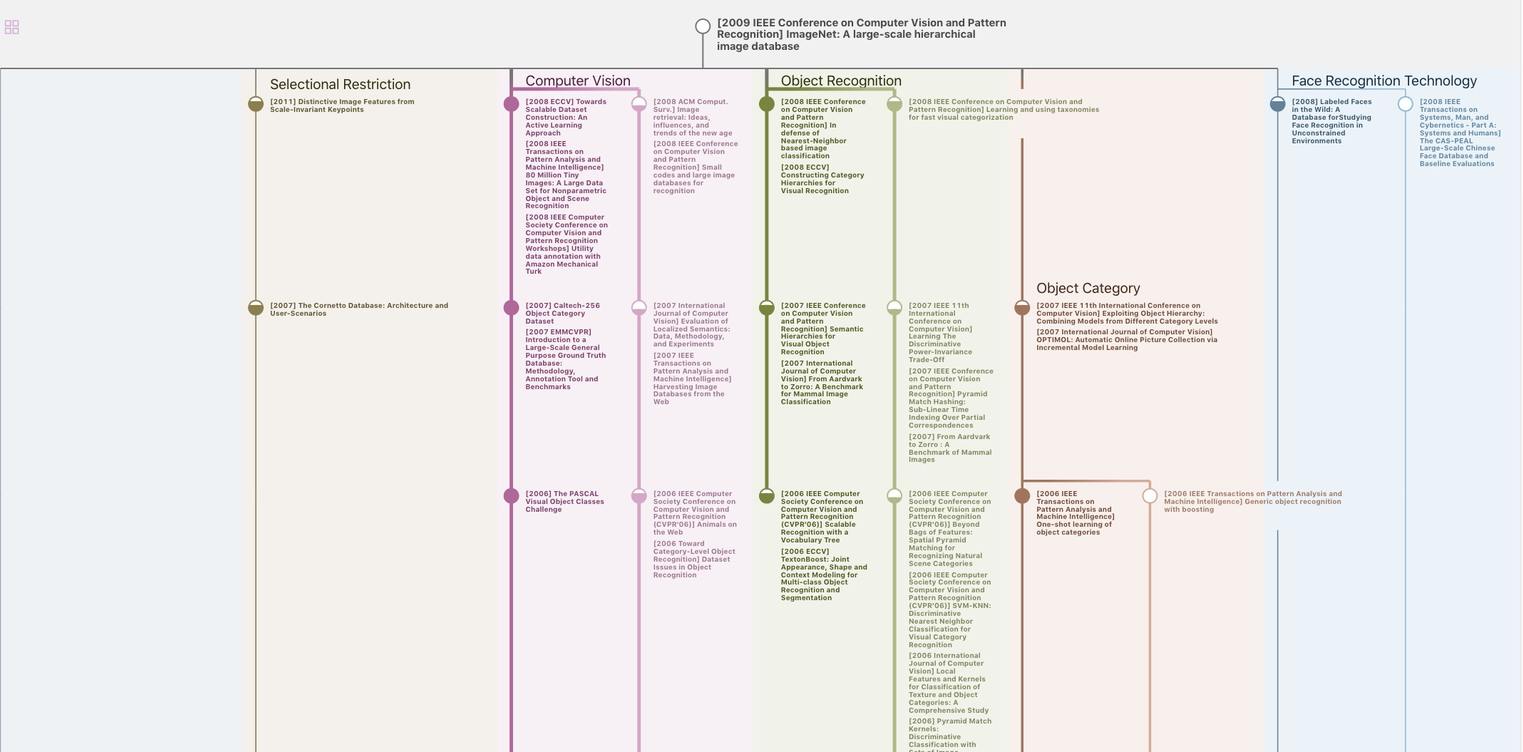
生成溯源树,研究论文发展脉络
Chat Paper
正在生成论文摘要