Reinforcement Learning to Solve NP-hard Problems: an Application to the CVRP
arxiv(2022)
摘要
In this paper, we evaluate the use of Reinforcement Learning (RL) to solve a classic combinatorial optimization problem: the Capacitated Vehicle Routing Problem (CVRP). We formalize this problem in the RL framework and compare two of the most promising RL approaches with traditional solving techniques on a set of benchmark instances. We measure the different approaches with the quality of the solution returned and the time required to return it. We found that despite not returning the best solution, the RL approach has many advantages over traditional solvers. First, the versatility of the framework allows the resolution of more complex combinatorial problems. Moreover, instead of trying to solve a specific instance of the problem, the RL algorithm learns the skills required to solve the problem. The trained policy can then quasi instantly provide a solution to an unseen problem without having to solve it from scratch. Finally, the use of trained models makes the RL solver by far the fastest, and therefore make this approach more suited for commercial use where the user experience is paramount. Techniques like Knowledge Transfer can also be used to improve the training efficiency of the algorithm and help solve bigger and more complex problems.
更多查看译文
关键词
reinforcement learning,np-hard
AI 理解论文
溯源树
样例
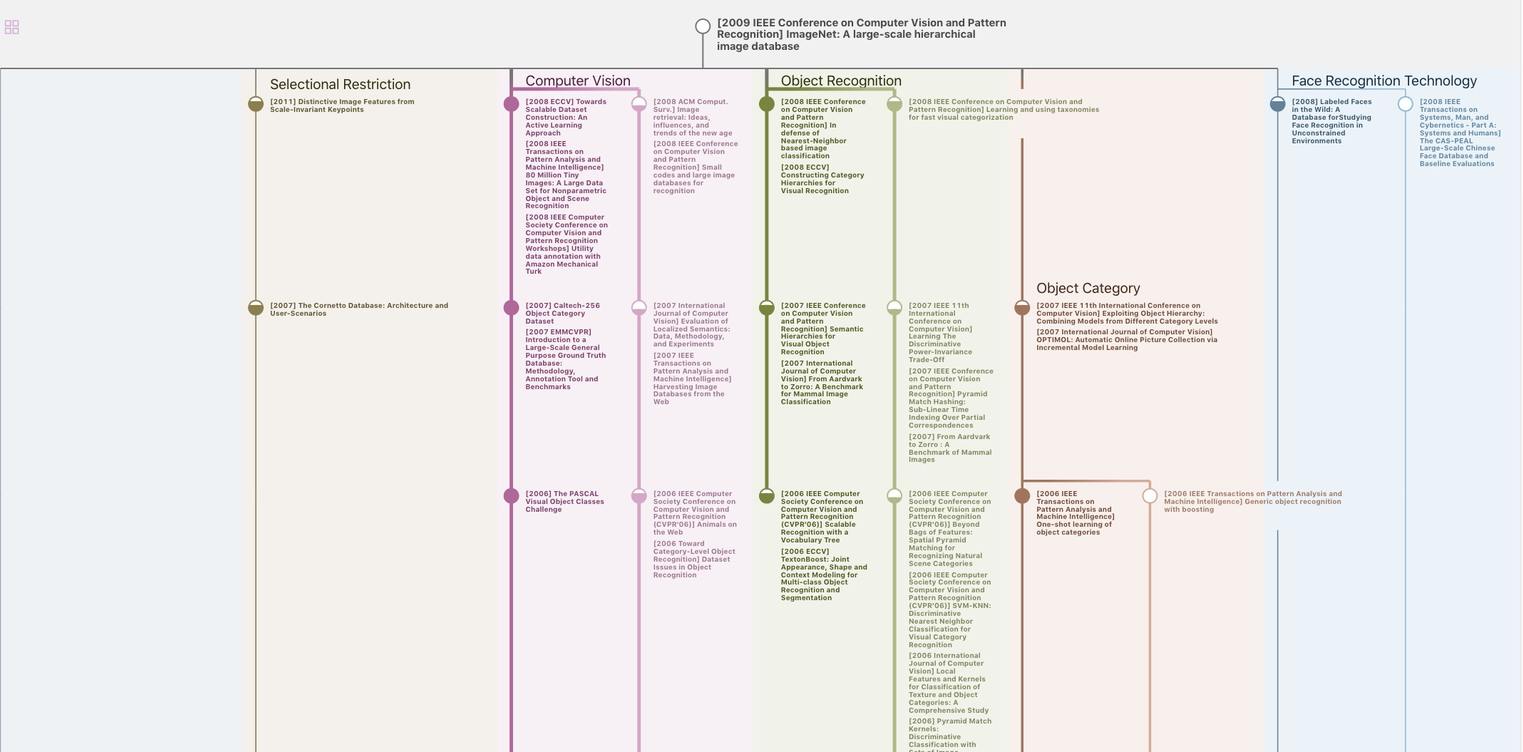
生成溯源树,研究论文发展脉络
Chat Paper
正在生成论文摘要