Semantic Decoupled Representation Learning for Remote Sensing Image Change Detection.
IEEE International Geoscience and Remote Sensing Symposium (IGARSS)(2022)
摘要
Contemporary transfer learning-based methods to alleviate the data insufficiency in change detection (CD) are mainly based on ImageNet pre-training. Self-supervised learning (SSL) has recently been introduced to remote sensing (RS) for learning in-domain representations. Here, we propose a semantic decoupled representation learning for RS image CD. Typically, the object of interest (e.g., building) is relatively small compared to the vast background. Different from existing methods expressing an image into one representation vector that may be dominated by irrelevant land-covers, we disentangle representations of different semantic regions by leveraging the semantic mask. We additionally force the model to distinguish different semantic representations, which benefits the recognition of objects of interest in the downstream CD task. We construct a dataset of bitemporal images with semantic masks in an effortless manner for pre-training. Experiments on two CD datasets show our model outperforms ImageNet pre-training, in-domain supervised pre-training, and several recent SSL methods.
更多查看译文
关键词
remote sensing,detection,learning,representation
AI 理解论文
溯源树
样例
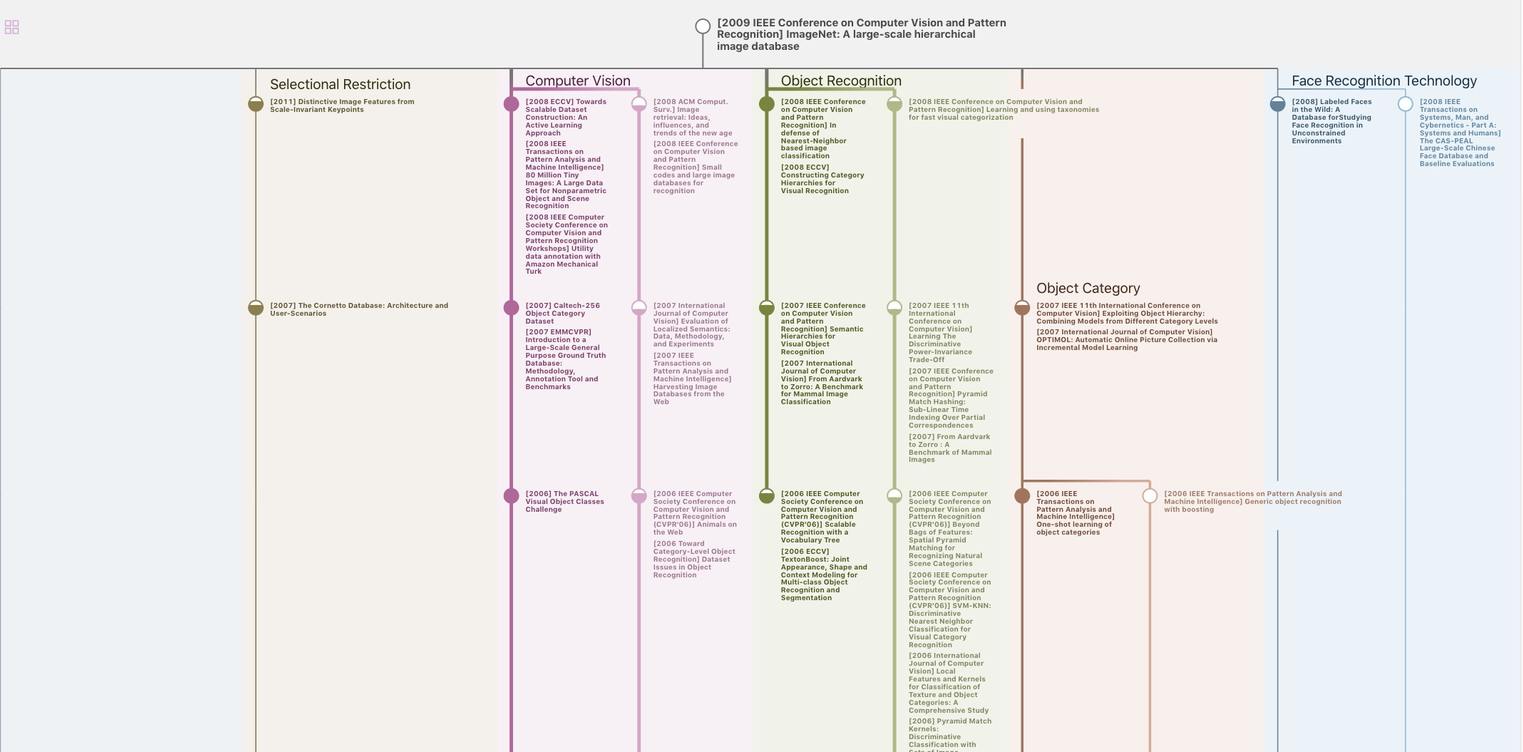
生成溯源树,研究论文发展脉络
Chat Paper
正在生成论文摘要