Visual Identification of Problematic Bias in Large Label Spaces
arxiv(2022)
摘要
While the need for well-trained, fair ML systems is increasing ever more, measuring fairness for modern models and datasets is becoming increasingly difficult as they grow at an unprecedented pace. One key challenge in scaling common fairness metrics to such models and datasets is the requirement of exhaustive ground truth labeling, which cannot always be done. Indeed, this often rules out the application of traditional analysis metrics and systems. At the same time, ML-fairness assessments cannot be made algorithmically, as fairness is a highly subjective matter. Thus, domain experts need to be able to extract and reason about bias throughout models and datasets to make informed decisions. While visual analysis tools are of great help when investigating potential bias in DL models, none of the existing approaches have been designed for the specific tasks and challenges that arise in large label spaces. Addressing the lack of visualization work in this area, we propose guidelines for designing visualizations for such large label spaces, considering both technical and ethical issues. Our proposed visualization approach can be integrated into classical model and data pipelines, and we provide an implementation of our techniques open-sourced as a TensorBoard plug-in. With our approach, different models and datasets for large label spaces can be systematically and visually analyzed and compared to make informed fairness assessments tackling problematic bias.
更多查看译文
关键词
problematic bias,large label spaces,visual identification
AI 理解论文
溯源树
样例
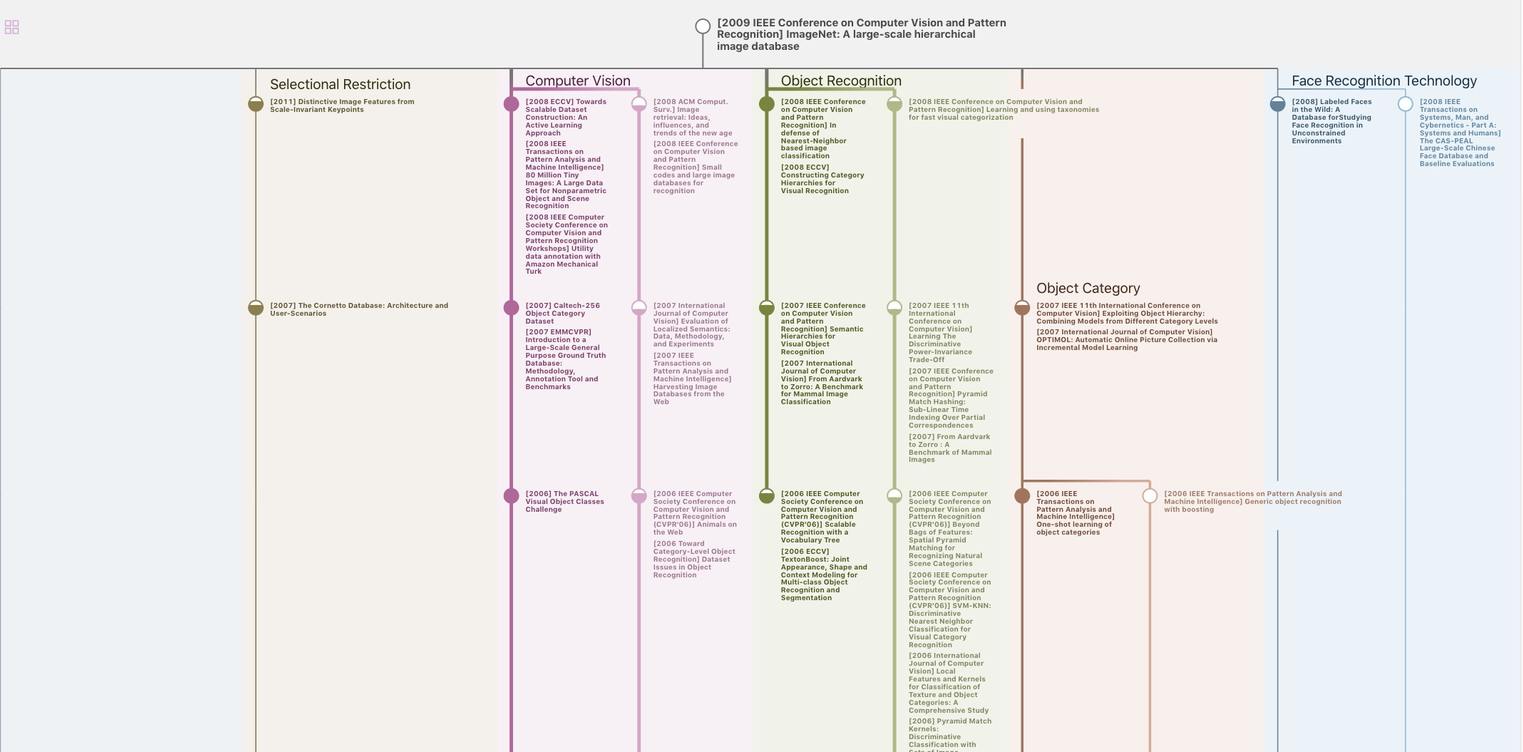
生成溯源树,研究论文发展脉络
Chat Paper
正在生成论文摘要