Target Detection With Unconstrained Linear Mixture Model and Hierarchical Denoising Autoencoder in Hyperspectral Imagery
IEEE TRANSACTIONS ON IMAGE PROCESSING(2022)
摘要
Hyperspectral imagery with very high spectral resolution provides a new insight for subtle nuances identification of similar substances. However, hyperspectral target detection faces significant challenges of intraclass dissimilarity and interclass similarity due to the unavoidable interference caused by atmosphere, illumination, and sensor noise. In order to effectively alleviate these spectral inconsistencies, this paper proposes a novel target detection method without strict assumptions on data distribution based on an unconstrained linear mixture model and deep learning. Our proposed detector firstly reduces interference via a specifically designed deep-learning-based hierarchical denoising autoencoder, and then carries out accurate detection with a two-step subspace projection, aiming at background suppression and target enhancement. Additionally, to generate representative background and reliable target samples required in the detection procedure, an efficient spatial-spectral unified endmember extraction method has been developed. Performance comparison with several state-of-the-art detection methods and further analysis on four real-world hyperspectral images demonstrate the effectiveness and efficiency of our proposed target detector.
更多查看译文
关键词
Detectors, Object detection, Hyperspectral imaging, Noise reduction, Mixture models, Interference, Deep learning, Unconstrained linear mixture model, hierarchical denoising autoencoder, spatial-spectral unification, hyperspectral target detection
AI 理解论文
溯源树
样例
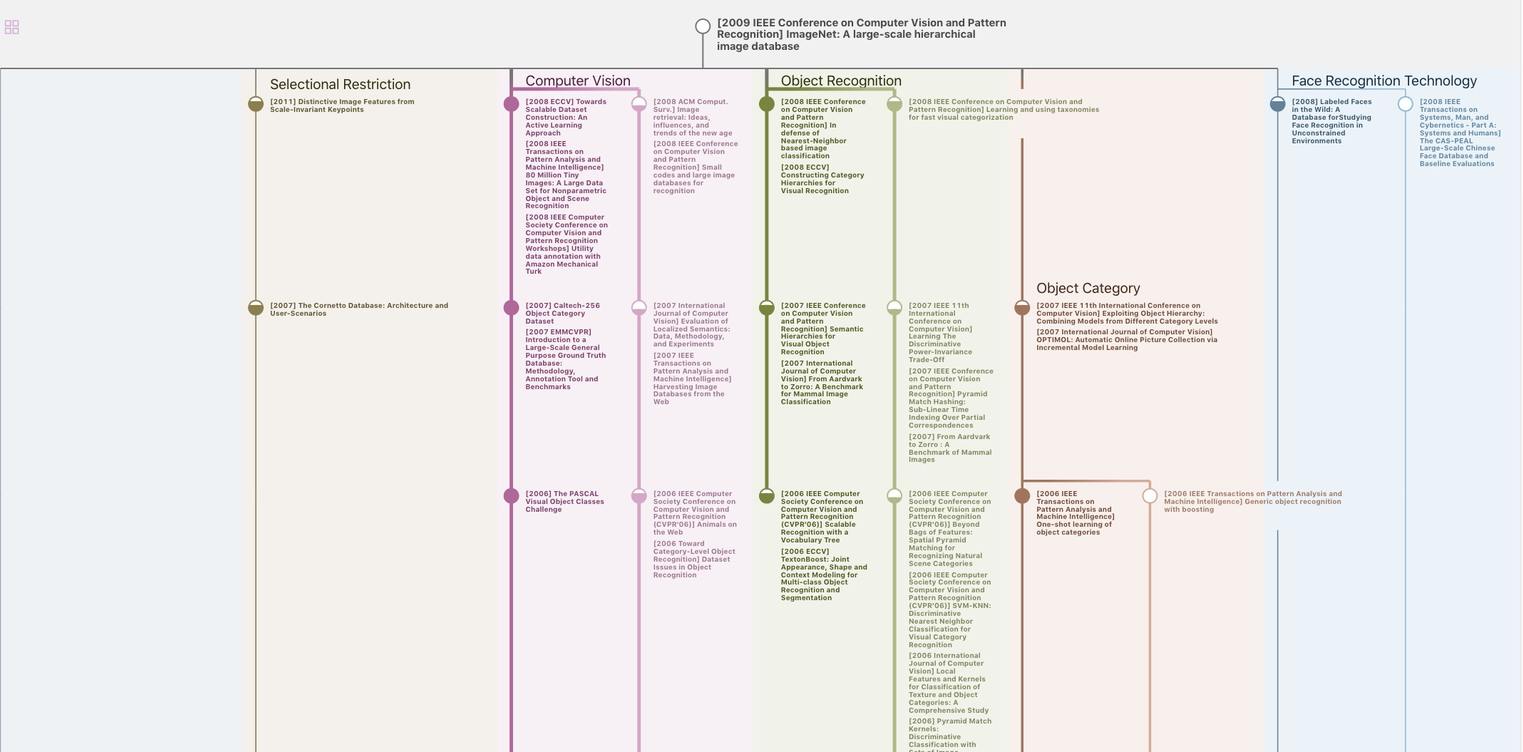
生成溯源树,研究论文发展脉络
Chat Paper
正在生成论文摘要