Transparent single-cell set classification with kernel mean embeddings
Bioinformatics, Computational Biology and Biomedicine(2022)
摘要
BSTRACTModern single-cell flow and mass cytometry technologies measure the expression of several proteins of the individual cells within a blood or tissue sample. Each profiled biological sample is thus represented by a set of hundreds of thousands of multidimensional cell feature vectors, which incurs a high computational cost to predict each biological sample's associated phenotype with machine learning models. Such a large set cardinality also limits the interpretability of machine learning models due to the difficulty in tracking how each individual cell influences the ultimate prediction. We propose using Kernel Mean Embedding to encode the cellular landscape of each profiled biological sample. Although our foremost goal is to make a more transparent model, we find that our method achieves comparable or better accuracies than the state-of-the-art gating-free methods through a simple linear classifier. As a result, our model contains few parameters but still performs similarly to deep learning models with millions of parameters. In contrast with deep learning approaches, the linearity and sub-selection step of our model makes it easy to interpret classification results. Analysis further shows that our method admits rich biological interpretability for linking cellular heterogeneity to clinical phenotype.
更多查看译文
关键词
kernel mean embeddings,classification,single-cell
AI 理解论文
溯源树
样例
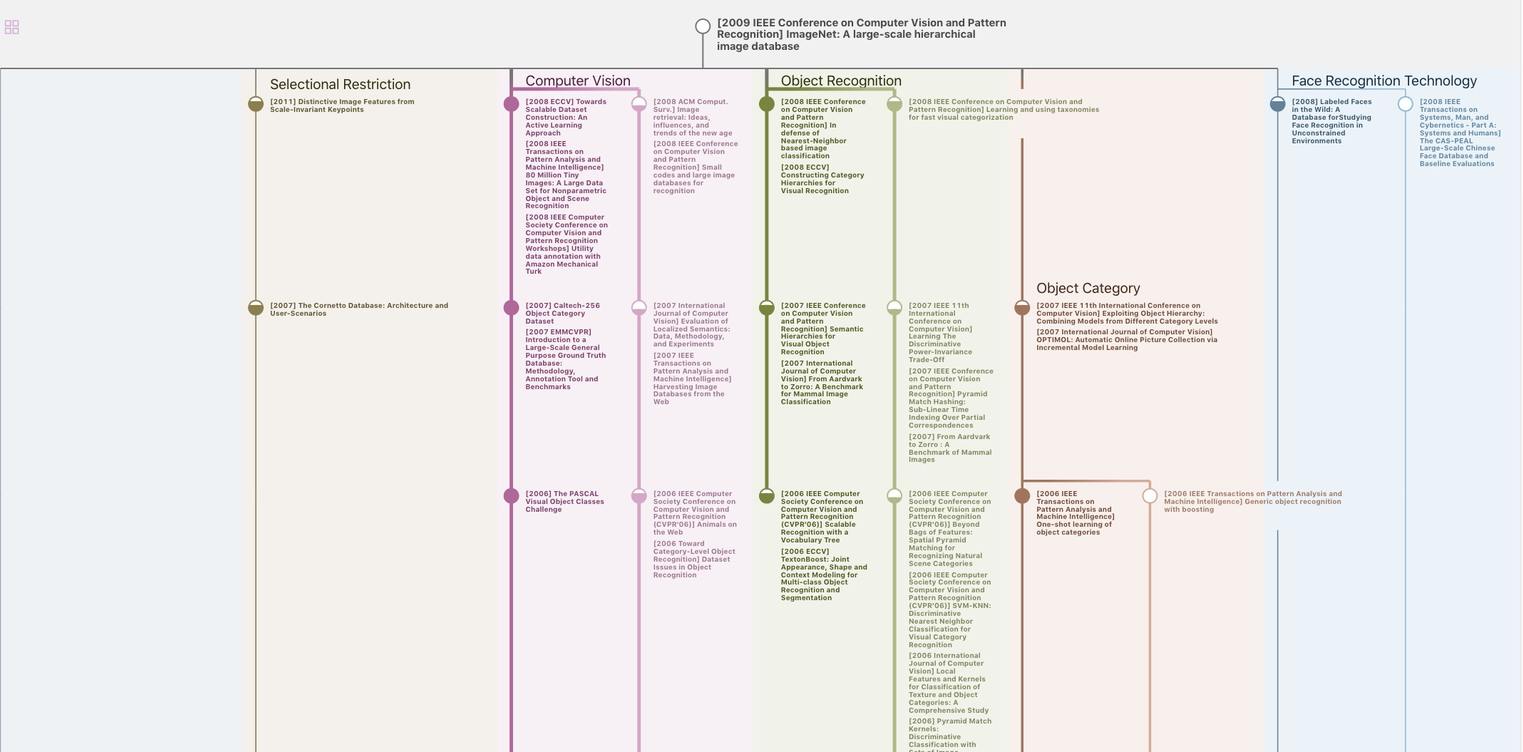
生成溯源树,研究论文发展脉络
Chat Paper
正在生成论文摘要