Convergence acceleration in machine learning potentials for atomistic simulations
arxiv(2022)
摘要
Machine learning potentials (MLPs) for atomistic simulations have an enormous prospective impact on materials modeling, offering orders of magnitude speedup over density functional theory (DFT) calculations without appreciably sacrificing accuracy in the prediction of material properties. However, the generation of large datasets needed for training MLPs is daunting. Herein, we show that MLP-based material property predictions converge faster with respect to precision for Brillouin zone integrations than DFT-based property predictions. We demonstrate that this phenomenon is robust across material properties for different metallic systems. Further, we provide statistical error metrics to accurately determine a priori the precision level required of DFT training datasets for MLPs to ensure accelerated convergence of material property predictions, thus significantly reducing the computational expense of MLP development.
更多查看译文
关键词
machine learning potentials,atomistic
AI 理解论文
溯源树
样例
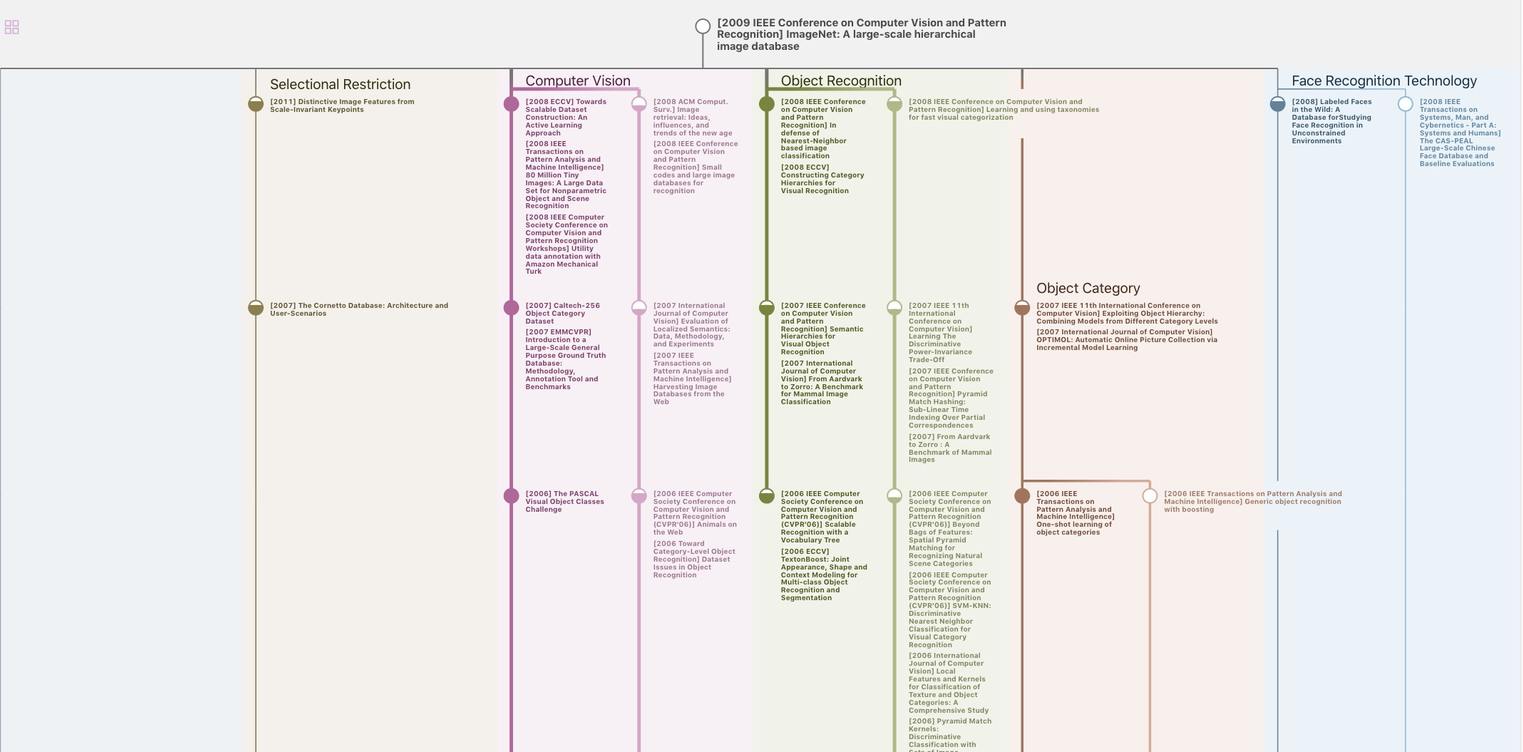
生成溯源树,研究论文发展脉络
Chat Paper
正在生成论文摘要