AutoDerain: Memory-efficient Neural Architecture Search for Image Deraining
2021 International Conference on Visual Communications and Image Processing (VCIP)(2021)
摘要
Learning-based image deraining methods have achieved remarkable success in the past few decades. Currently, most deraining architectures are developed by human experts, which is a laborious and error-prone process. In this paper, we present a study on employing neural architecture search (NAS) to automatically design deraining architectures, dubbed AutoDerain. Specifically, we first propose an U-shaped deraining architecture, which mainly consists of residual squeeze-and-excitation blocks (RSEBs). Then, we define a search space, where we search for the convolutional types and the use of the squeeze-and-excitation block. Considering that the differentiable architecture search is memory-intensive, we propose a memory-efficient differentiable architecture search scheme (MDARTS). In light of the success of training binary neural networks, MDARTS optimizes architecture parameters through the proximal gradient, which only consumes the same GPU memory as training a single deraining model. Experimental results demonstrate that the architecture designed by MDARTS is superior to manually designed derainers.
更多查看译文
关键词
memory-efficient,differentiable architecture search,image deraining
AI 理解论文
溯源树
样例
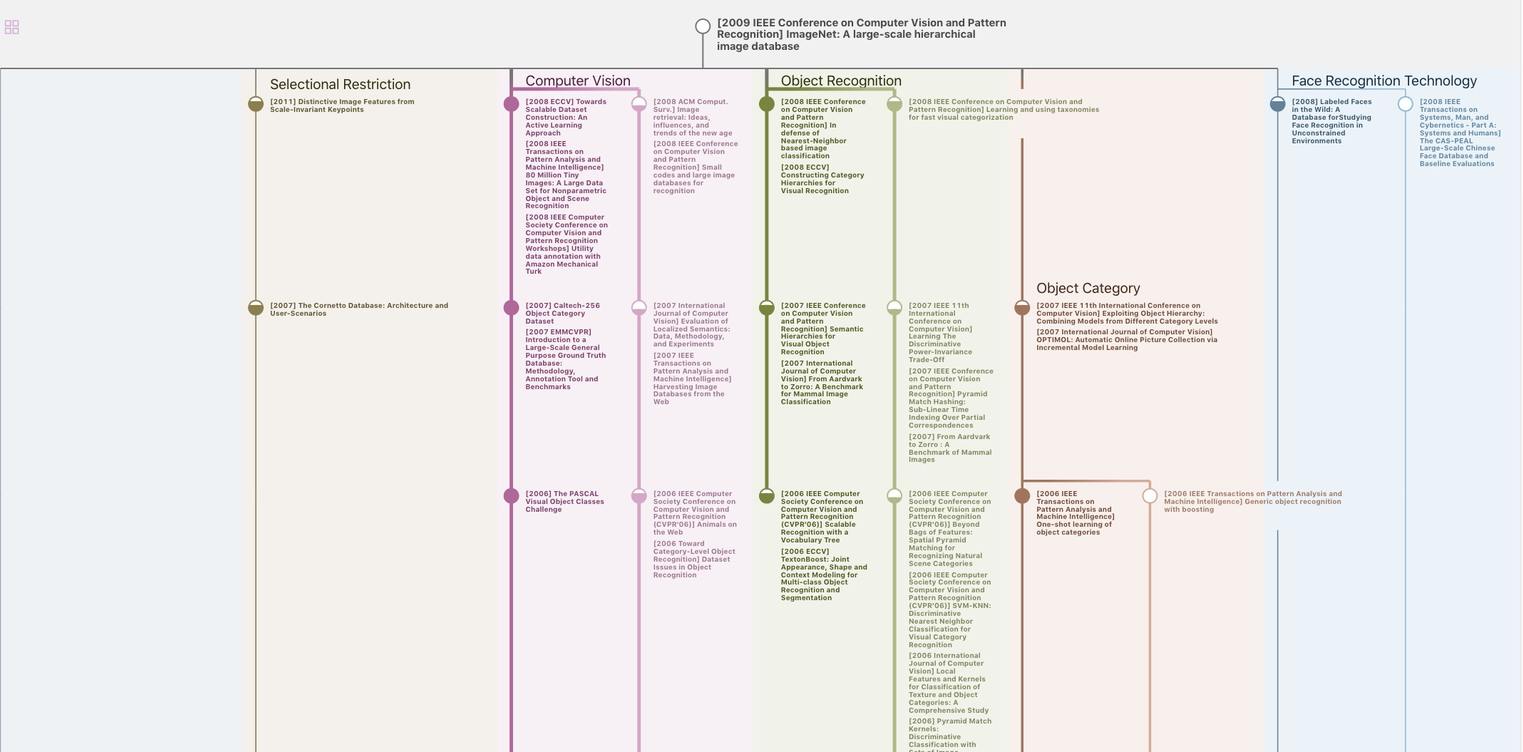
生成溯源树,研究论文发展脉络
Chat Paper
正在生成论文摘要