Bayesian Prediction with Covariates Subject to Detection Limits
arxiv(2022)
摘要
Missing values in covariates due to censoring by signal interference or lack of sensitivity in the measuring devices are common in industrial problems. We propose a full Bayesian solution to the prediction problem with an efficient Markov Chain Monte Carlo (MCMC) algorithm that updates all the censored covariate values jointly in a random scan Gibbs sampler. We show that the joint updating of missing covariate values can be at least two orders of magnitude more efficient than univariate updating. This increased efficiency is shown to be crucial for quickly learning the missing covariate values and their uncertainty in a real-time decision making context, in particular when there is substantial correlation in the posterior for the missing values. The approach is evaluated on simulated data and on data from the telecom sector. Our results show that the proposed Bayesian imputation gives substantially more accurate predictions than na\"ive imputation, and that the use of auxiliary variables in the imputation gives additional predictive power.
更多查看译文
关键词
bayesian prediction,detection,covariates subject,limits
AI 理解论文
溯源树
样例
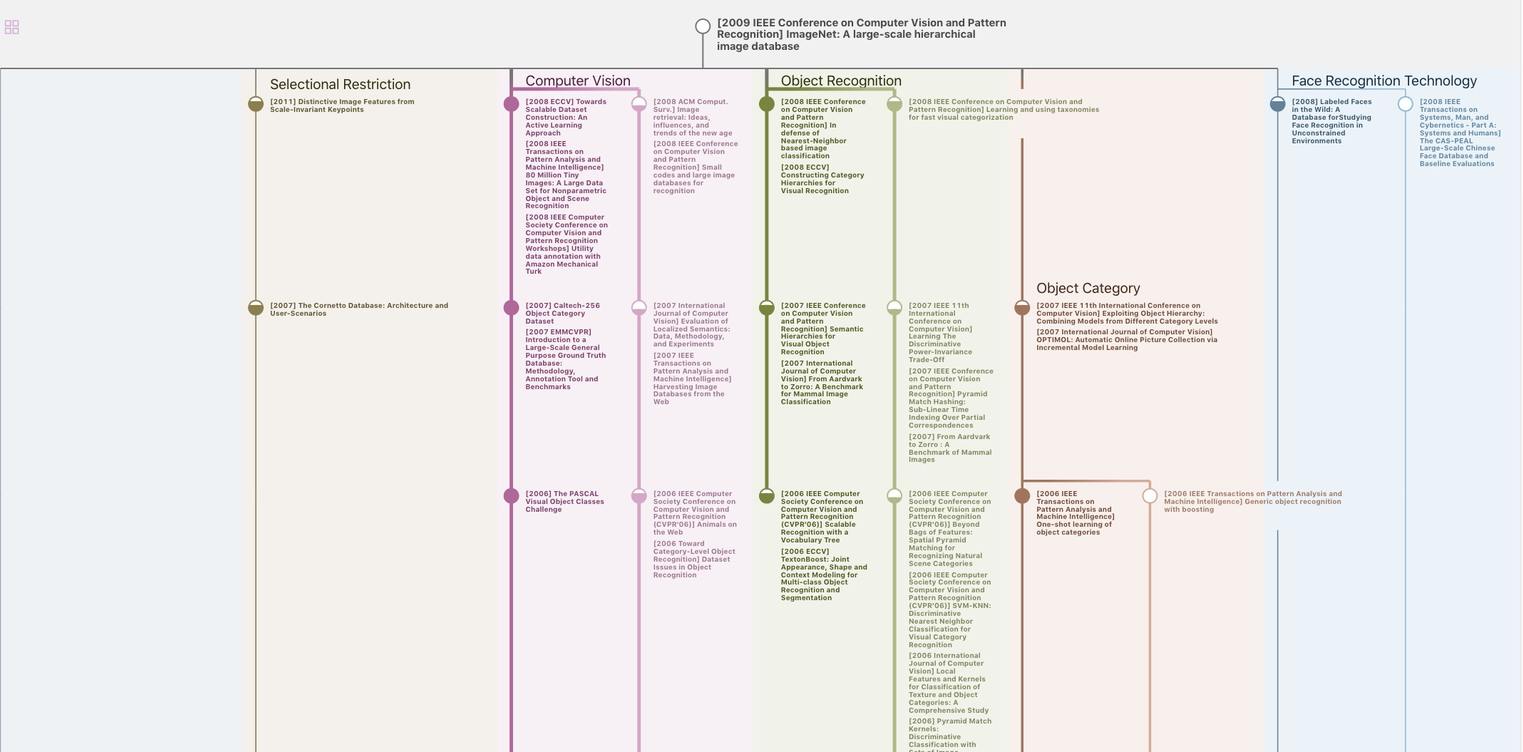
生成溯源树,研究论文发展脉络
Chat Paper
正在生成论文摘要