CELESTIAL: Classification Enabled via Labelless Embeddings with Self-supervised Telescope Image Analysis Learning
arxiv(2022)
摘要
A common class of problems in remote sensing is scene classification, a fundamentally important task for natural hazards identification, geographic image retrieval, and environment monitoring. Recent developments in this field rely label-dependent supervised learning techniques which is antithetical to the 35 petabytes of unlabelled satellite imagery in NASA GIBS. To solve this problem, we establish CELESTIAL-a self-supervised learning pipeline for effectively leveraging sparsely-labeled satellite imagery. This pipeline successfully adapts SimCLR, an algorithm that first learns image representations on unlabelled data and then fine-tunes this knowledge on the provided labels. Our results show CELESTIAL requires only a third of the labels that the supervised method needs to attain the same accuracy on an experimental dataset. The first unsupervised tier can enable applications such as reverse image search for NASA Worldview (i.e. searching similar atmospheric phenomenon over years of unlabelled data with minimal samples) and the second supervised tier can lower the necessity of expensive data annotation significantly. In the future, we hope we can generalize the CELESTIAL pipeline to other data types, algorithms, and applications.
更多查看译文
关键词
labelless embeddings,celestial,classification,learning,image,self-supervised
AI 理解论文
溯源树
样例
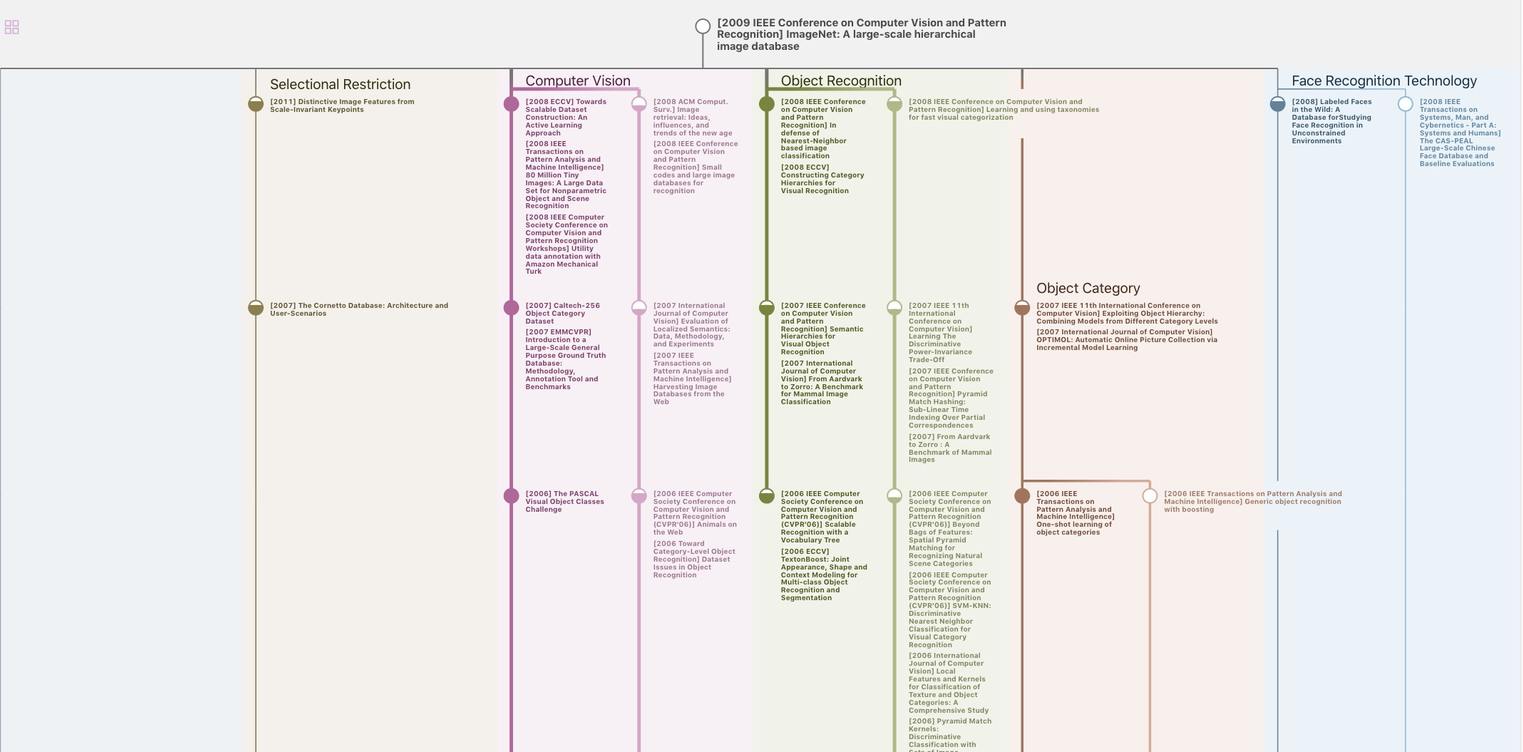
生成溯源树,研究论文发展脉络
Chat Paper
正在生成论文摘要