Drift Lens: Real-time unsupervised Concept Drift detection by evaluating per-label embedding distributions.
2021 International Conference on Data Mining Workshops (ICDMW)(2021)
摘要
Despite the significant improvements made by deep learning models, their adoption in real-world dynamic applications is still limited. Concept drift is among the open issues preventing the widespread exploitation of deep learning models in real-life settings. The dynamic world changes very quickly, and the collected data drifts accordingly. Prediction models, usually trained on static historical data, should be promptly re-trained in case of new real-time drifted data distributions. Although some drift detection methodologies have been proposed over the years, different issues are still open since state-of-the-art solutions show limited effectiveness and efficiency. This paper proposes DRIFT LENS, a novel real-time unsupervised per-label drift detection methodology based on embedding distribution distances in deep learning models. The preliminary experiments performed on a transformer-based model fine-tuned for topic text classification show promising results in drift detection accuracy, drift characterization, and efficient execution time to support real-time concept drift detection.
更多查看译文
关键词
Data drift,MLOps,deep learning,transformerbased models,text topic classification
AI 理解论文
溯源树
样例
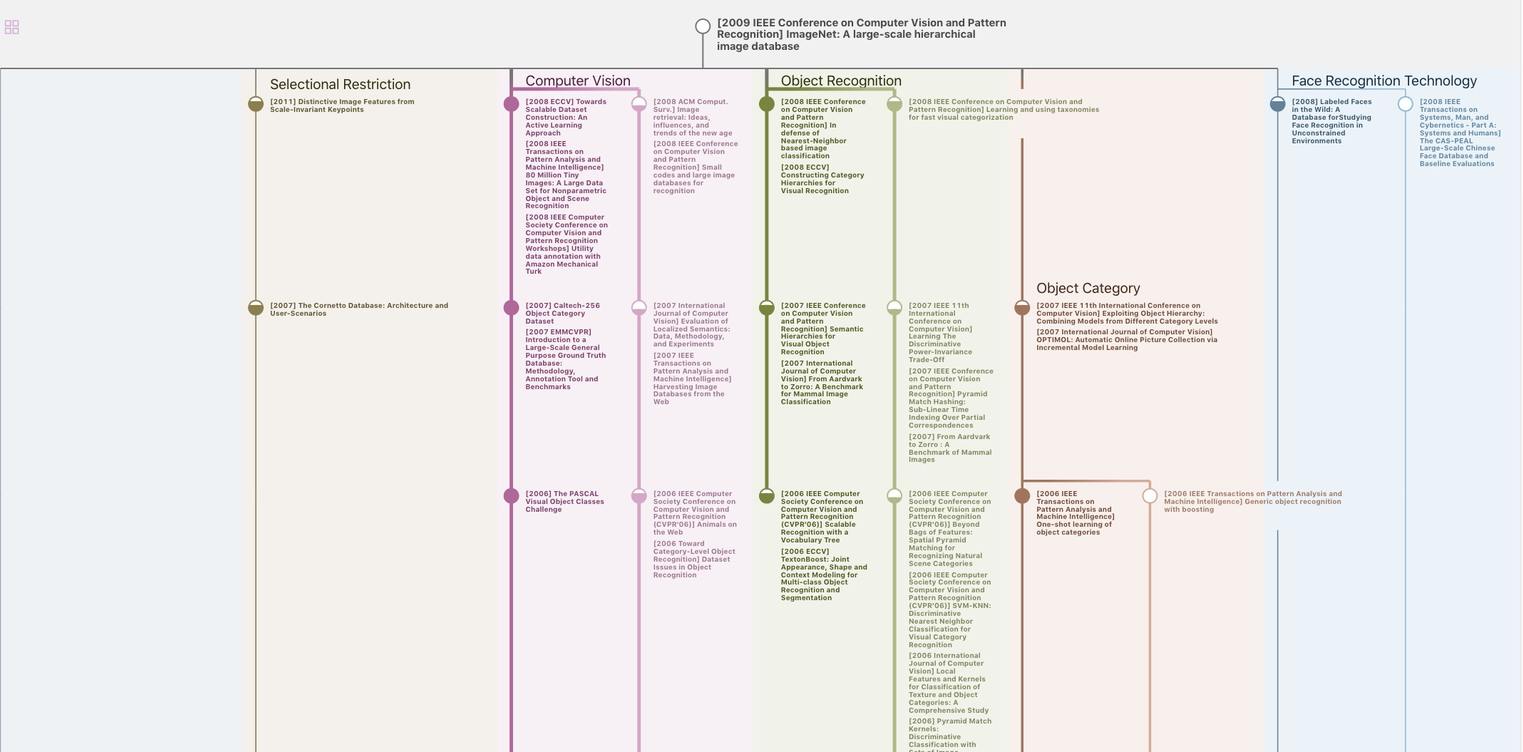
生成溯源树,研究论文发展脉络
Chat Paper
正在生成论文摘要